Large Language Models Augmented Rating Prediction in Recommender System
ICASSP 2024 - 2024 IEEE International Conference on Acoustics, Speech and Signal Processing (ICASSP)(2024)
摘要
Recently, large language models (LLMs) have demonstrated impressive capabilities and gained widespread applications. However, their direct application to recommendation tasks (e.g., rating prediction task) often falls short of optimal results due to a lack of understanding of collaborative information in recommendations. In this paper, we propose Large lAnguage Model Augmented Recommendation (LAMAR) framework to address this limitation. Instead of relying solely on LLMs, our framework combines their outputs with traditional recommendation models, leveraging both collaborative and semantic information. We further enhance the recommendation performance through an ensemble of diverse prompts and utilize LLMs to extract side information for augmenting traditional recommendation models. Empirical studies on real-world datasets demonstrate that LAMAR outperforms existing approaches, highlighting the benefits of leveraging LLMs in recommendation systems. Code is available at https: //github.com/sichunluo/LAMAR.
更多查看译文
关键词
recommender system,large language model,rating prediction
AI 理解论文
溯源树
样例
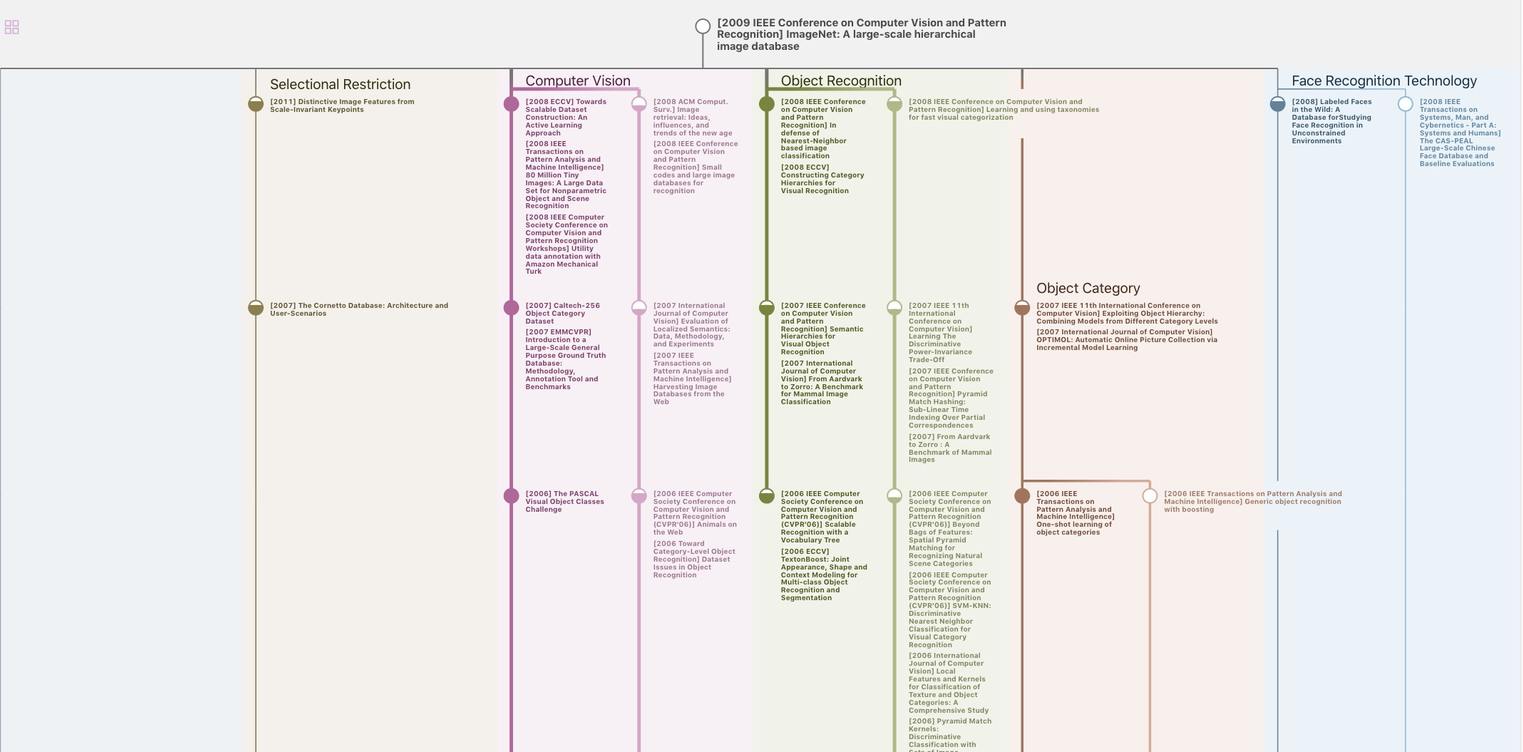
生成溯源树,研究论文发展脉络
Chat Paper
正在生成论文摘要