Language-Driven Open-Vocabulary 3D Semantic Segmentation with Knowledge Distillation
ICASSP 2024 - 2024 IEEE International Conference on Acoustics, Speech and Signal Processing (ICASSP)(2024)
摘要
3D open-vocabulary semantic segmentation is a challenge in the task of 3D scene understanding, as most current models trained on closed-set datasets struggle to effectively identify categories that were not seen during training. To address this, we introduce a framework called LSWKD. It distills knowledge from a pre-trained 3D open-world model, thereby enhancing the alignment between visual and semantic features. Furthermore, we employ Point-discriminative Contrastive Learning to compute caption loss in the teacher model instead of CLIP-style Contrastive Loss in order to let each point be supervised with its all related language captions, which improves the teacher model’s performance. We conducted experiments on ScanNet and S3DIS datasets. The results demonstrate that our approach achieves better hIoU compared with state-of-the-art models. Code will be released at https://github.com/wu39848/LSWKD.
更多查看译文
关键词
3D open-vocabulary semantic segmentation,Vision-language model,Knowledge Distillation,Contrastive Learning
AI 理解论文
溯源树
样例
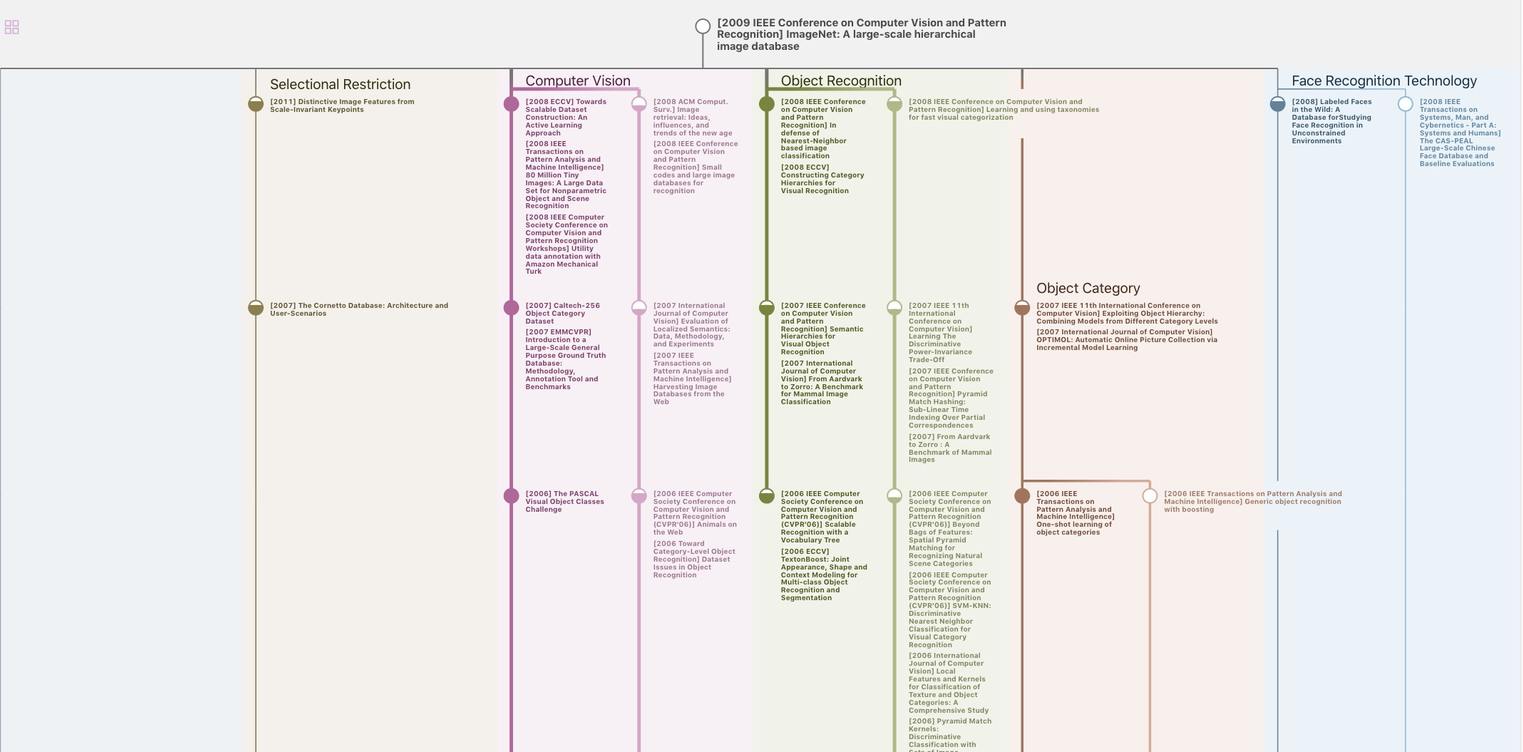
生成溯源树,研究论文发展脉络
Chat Paper
正在生成论文摘要