Mutual Information Assisted Graph Convolution Network for Cold-Start Recommendation
ICASSP 2024 - 2024 IEEE International Conference on Acoustics, Speech and Signal Processing (ICASSP)(2024)
摘要
To solve the cold-start issue that cold items have no historical interactions to obtain collaborative feature as their representation, existing methods often represent them totally based on content feature obtained from inherent content (i.e., image, video and attributes). However, these methods will lose efficacy in representing the cold item whose inherent content is much different from that of warm items, when the cold item first appears in the test stage. In this paper, we propose a mutual information assisted graph convolution network (MIGCN), which represents the cold item by simultaneously considering its inherent content and its related users’ feature captured by pair-wise mutual information (PMI). In addition, for improving the overall performance, a joint objective function that contains a relation loss and a similarity error loss is employed to achieve a better trade-off of the representation similarity between warm and cold items. Experiments conducted on the Amazon dataset demonstrate the superiority of our method, as compared with the state-of-the-art methods.
更多查看译文
关键词
Cold-start recommendation,historical interactions,inherent content,pair-wise mutual information
AI 理解论文
溯源树
样例
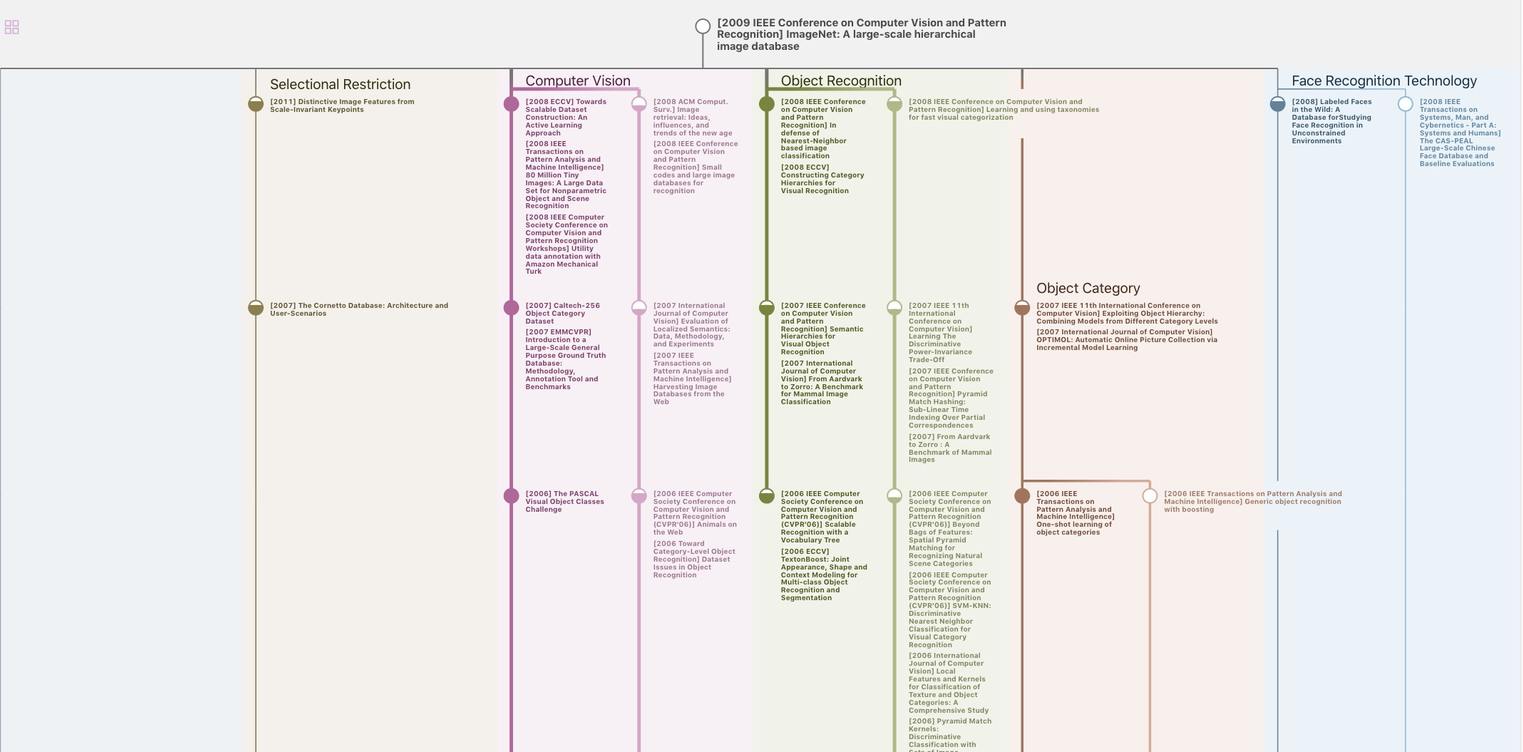
生成溯源树,研究论文发展脉络
Chat Paper
正在生成论文摘要