Spatial-Temporal Interaction Decoding Transformer for Unsupervised Multivariate Time Series Anomaly Detection
ICASSP 2024 - 2024 IEEE International Conference on Acoustics, Speech and Signal Processing (ICASSP)(2024)
摘要
Time series data consists of a temporal dimension and features associated with each timestamp. Anomaly detection in this context necessitates the consideration of both temporal and spatial features. However, existing work focuses on separately addressing temporal and spatial features, neglecting the interactive features between them. In this paper, we aim to leverage spatial-temporal interaction and propose a Spatial-Temporal inTeraction Decoding (STTD) model for time series anomaly detection. First, we employ the parallel transformer encoder to capture temporal dependencies at various scales and spatial dependencies among variables. Second, we propose a parallel transformer decoder with cross-attention to fuse spatial-temporal features. Moreover, we also utilize channel-attention to aggregate spatial features for better fusion. Experimental results on eight public datasets show that STTD outperforms state-of-the-art models, which shows the effectiveness of capturing spatial-temporal interaction.
更多查看译文
关键词
Deep learning,Time series,Anomaly detection,Transformer
AI 理解论文
溯源树
样例
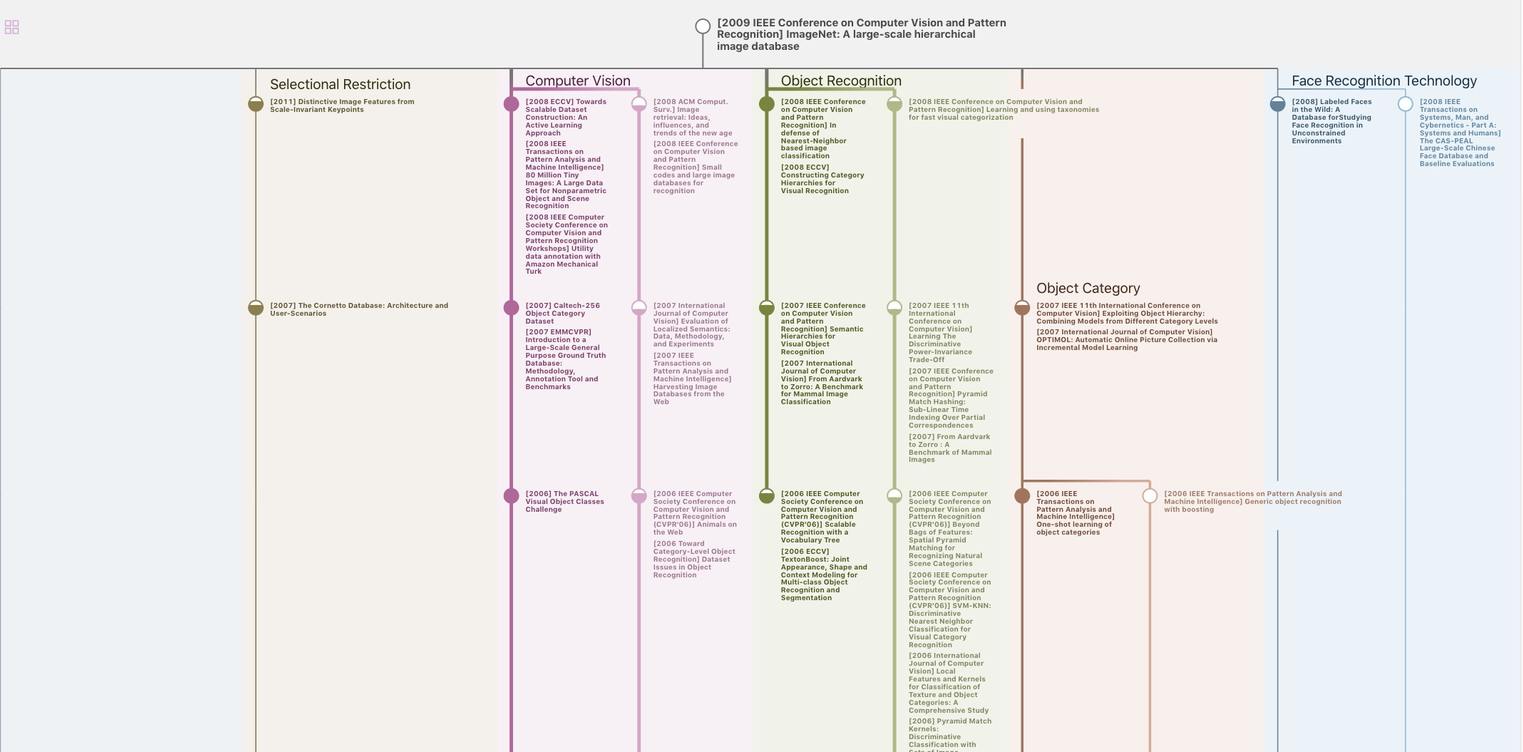
生成溯源树,研究论文发展脉络
Chat Paper
正在生成论文摘要