Drop Sparse Convolution for 3D Object Detection
ICASSP 2024 - 2024 IEEE International Conference on Acoustics, Speech and Signal Processing (ICASSP)(2024)
摘要
3D object detection based on point clouds is crucial for the safety of autonomous vehicles. The 3D feature network significantly impacts the feature extraction of the object detectors. However, the 3D feature network often exhibits poor performance due to submanifold dilation. In this paper, we propose a drop sparse convolution network, built using drop sparse convolution (Drop Conv), to mitigate the effects of submanifold dilation. Drop Conv calculates the activity of each feature by encoding itself and removes inactive features to preserve feature connectivity while suppressing dilated features. The proposed module can be easily integrated into existing 3D backbone networks. By combining it with existing sparse convolutions, it fills the gap in 3D backbone networks where sparsity cannot be enhanced. Extensive experiments on KITTI and nuScenes have demonstrated that the proposed method can effectively enhance the performance of state-of-the-art 3D object detectors using 3D feature networks.
更多查看译文
关键词
3D object detection,3D feature extraction,submanifold dilation,sparse convolution
AI 理解论文
溯源树
样例
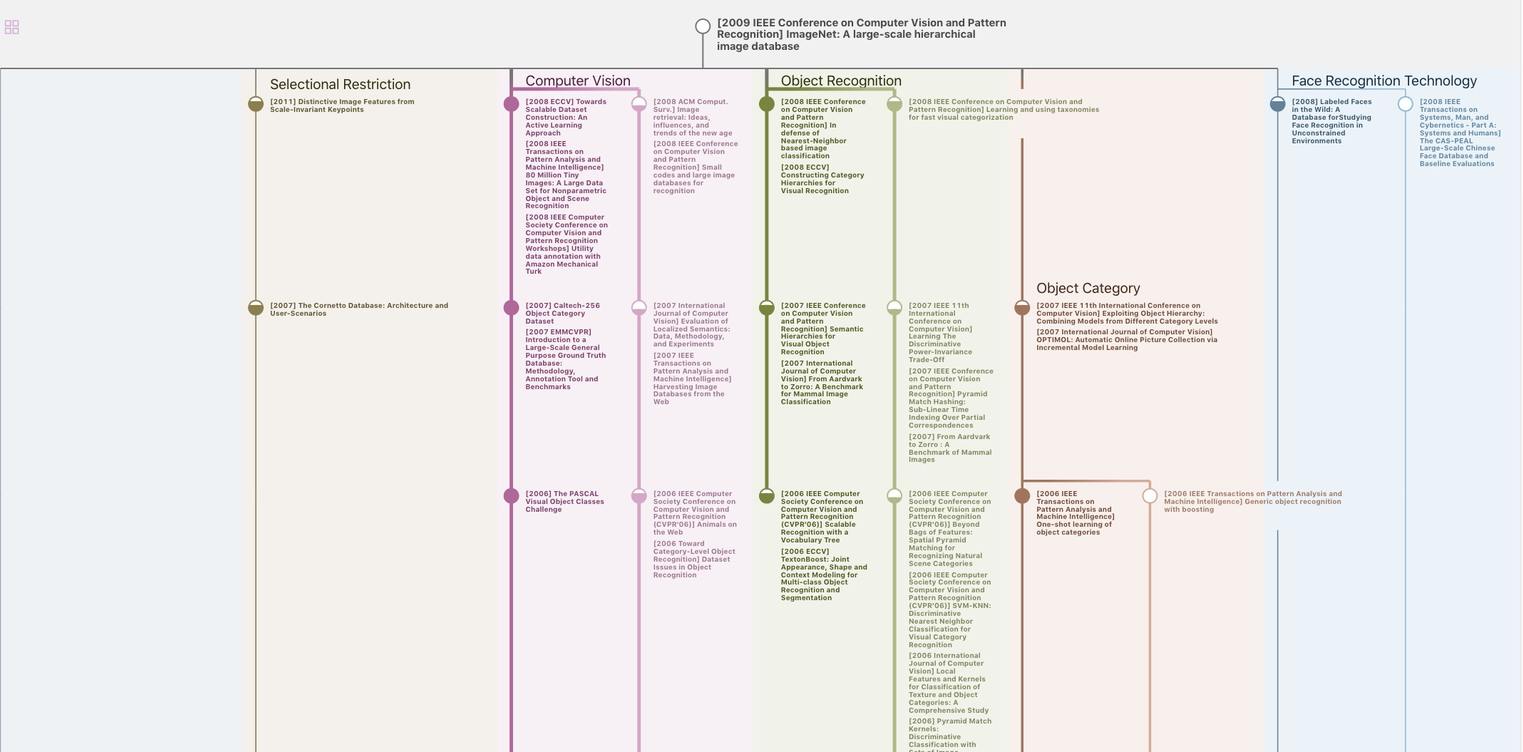
生成溯源树,研究论文发展脉络
Chat Paper
正在生成论文摘要