An Interpretable and Generalizable Speech Detector Based on a CNN-LSTM Framework
ICASSP 2024 - 2024 IEEE International Conference on Acoustics, Speech and Signal Processing (ICASSP)(2024)
摘要
Speech brain-computer interface (speech BCI) aims to reconstruct speech from recorded brain signals. Real-time speech BCI relies on speech detection, which is greatly impacted by the selection of speech-related neural frequency features. However, most studies did not investigate this aspect when designing speech detectors. In this study, both electrocorticography (ECoG) dataset and stereo-electroencephalography (sEEG) dataset were utilized to investigate the impact of brain signal type on the contribution of frequency bands to speech detection. We calculated the mutual information (MI) between neural frequency bands and the audio envelope and found that the distributions of frequency bands varied between the two types of brain signals. Specifically, the 40-60Hz of ECoG signal and 0-20Hz of sEEG signal got the highest MI values. To address this, we propose a two-module detector that combines convolutional neural networks and long short-term memory (CNN-LSTM) for feature extraction and speech prediction. Our detector outperformed three commonly used detectors, including Linear discriminant analysis (LDA), Support Vector Machine (SVM), and LSTM. Notably, a high correlation was found between CNN output and the frequency bands, and high MI values were observed in both types of brain signals. These findings confirm the interpretability and generalizability of our proposed speech detector.
更多查看译文
关键词
Speech Brain-computer Interfaces,Electrocorticography,Speech Detection,Frequency Band Analysis,CNN-LSTM
AI 理解论文
溯源树
样例
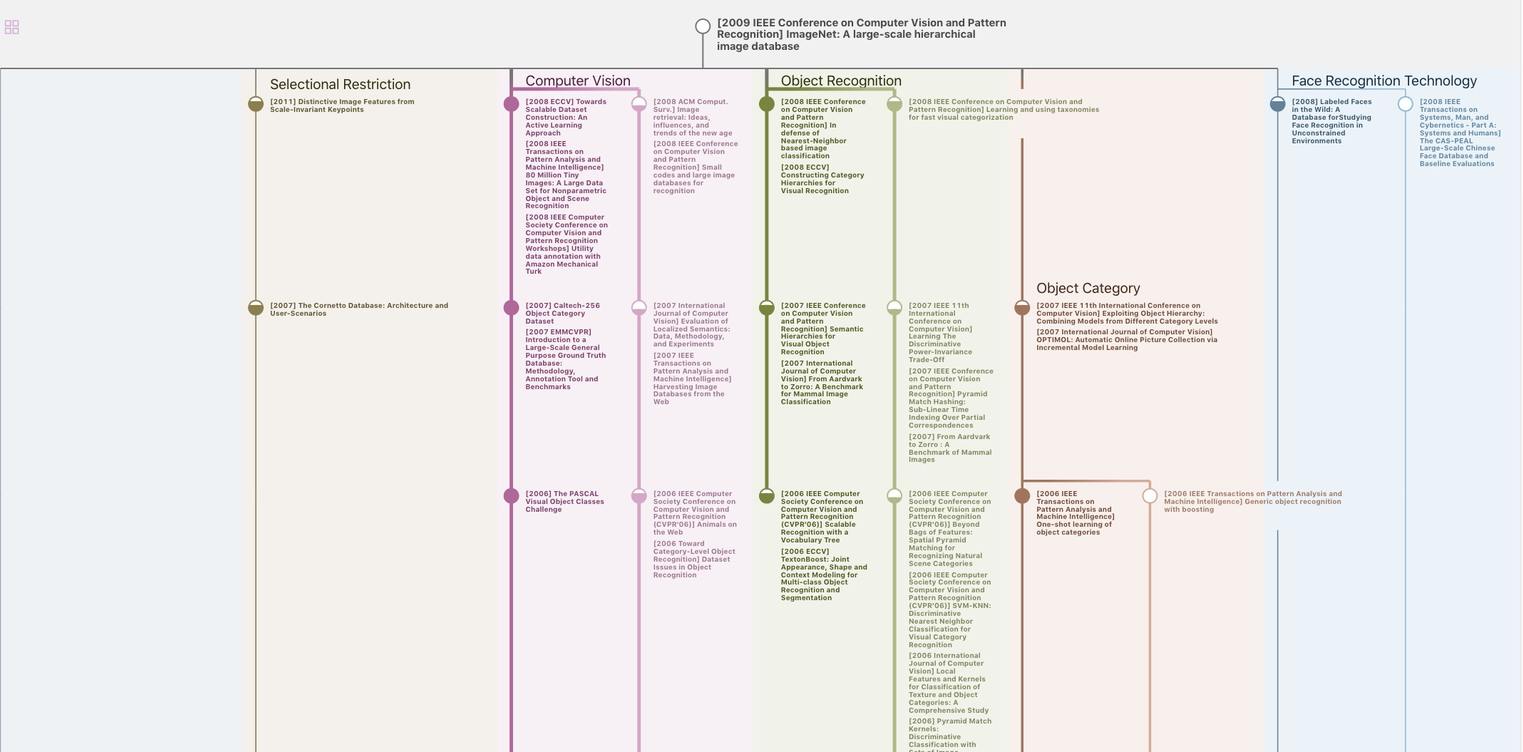
生成溯源树,研究论文发展脉络
Chat Paper
正在生成论文摘要