Boundary-Driven Active Learning for Anomaly Detection in Time Series Data Streams
ICASSP 2024 - 2024 IEEE International Conference on Acoustics, Speech and Signal Processing (ICASSP)(2024)
摘要
The key to anomaly detection in time series data streams (TSDS) lies in the ability to adapt to evolving data. Active learning for anomaly detection has shown such ability by leveraging expert feedback. However, many studies in this research line strive to optimize performance by exhausting the query budget, lacking consideration of query necessity, which means some unnecessary queries may wrongly lead the model to overfit the trivial information and incur additional consumption in both human labeling and model execution. This paper proposes Boundary-driven Active Learning for Anomaly Detection (BALAD). BALAD utilizes deep one-class classification to construct a hypersphere boundary to sense data abnormality and filters out unnecessary queries by dividing the boundary region. We further harness the hypersphere boundary to quantitatively measure data difficulty, and a focal loss is introduced to prioritize hard samples. The boundary is flexibly adapted during each feedback iteration to accommodate changes in TSDS. Extensive experiments on six datasets demonstrate that BALAD significantly outperforms the state-of-the-art anomaly detection methods.
更多查看译文
关键词
Time Series Data Streams,Anomaly Detection,Active Learning,Hypersphere Boundary
AI 理解论文
溯源树
样例
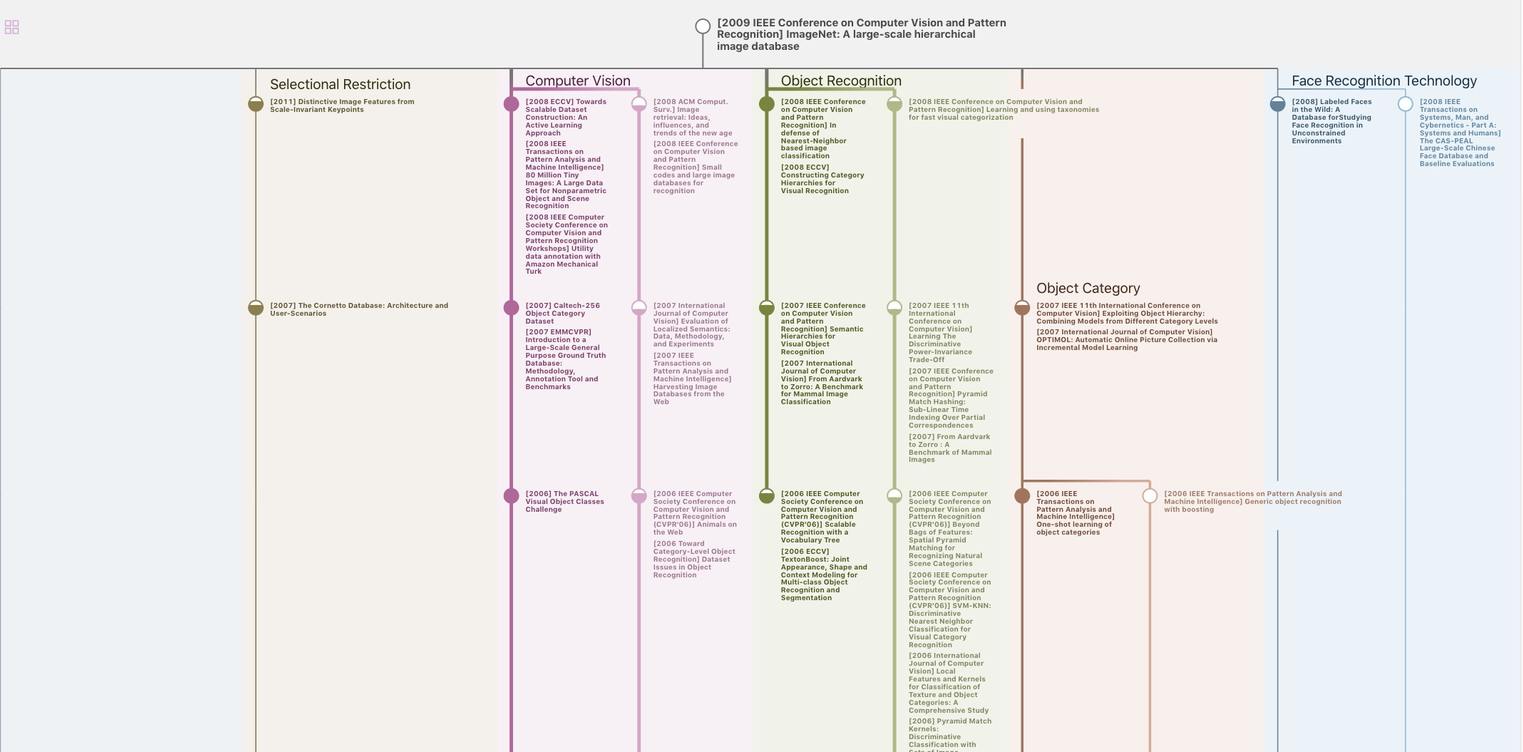
生成溯源树,研究论文发展脉络
Chat Paper
正在生成论文摘要