Online Caching With Switching Cost and Operational Long-Term Constraints: An Online Learning Approach
ICASSP 2024 - 2024 IEEE International Conference on Acoustics, Speech and Signal Processing (ICASSP)(2024)
摘要
The design of effective online caching policies is an increasingly important problem for content distribution networks, online recommender systems, and edge computing services, etc. Exiting literature usually tackles this problem through the lens of optimistic online learning and aims to achieve sublinear regret. In this paper, we focus on a non-trivial extension of classic online caching problem inspired by operational requirements of real-world systems including switching costs and long-term constraints. To tackle the challenges of switching costs and operational long-term constraints in the online caching, we introduce the Block-structured Follow-the-Regularized-Leader (B-FTRL) caching policy. Our approach incorporates a block structure that divides time into blocks to minimize caching switching costs. The theoretical analysis shows that B-FTRL achieves a utility regret bound of $O\left( {{T^{\frac{{2a - b + 1}}{{1 + a}}}} + {T^{\frac{b}{{1 + a}}}}} \right)$ and switching costs bound of $O\left( {{T^{\frac{1}{{1 + a}}}}} \right)$, where a and b are tunable algorithm parameters. By carefully selecting the values of a and b, we are able to limit the total regret to O(T
2/3
) while satisfying the operational long-term constraints in expectation. Additionally, we provide high-probability constraint violation bounds of $O\left( {\sqrt T } \right)$. The performance of the proposed algorithm is evaluated with detailed trace-driven numerical tests.
更多查看译文
关键词
Online Learning,Operational Constraints,Switching Costs,Online Learning Approach,Long-term Constraints,Algorithm Parameters,Recommender Systems,Block Structure,Constraint Violation,Extension Of Problem,Learning Rate,Performance Metrics,Time Slot,Long-term Perspective,Linear Constraints,Time Block,Probability 1,Utility Maximization,Overhead Costs,Configuration Time,Cache Hit
AI 理解论文
溯源树
样例
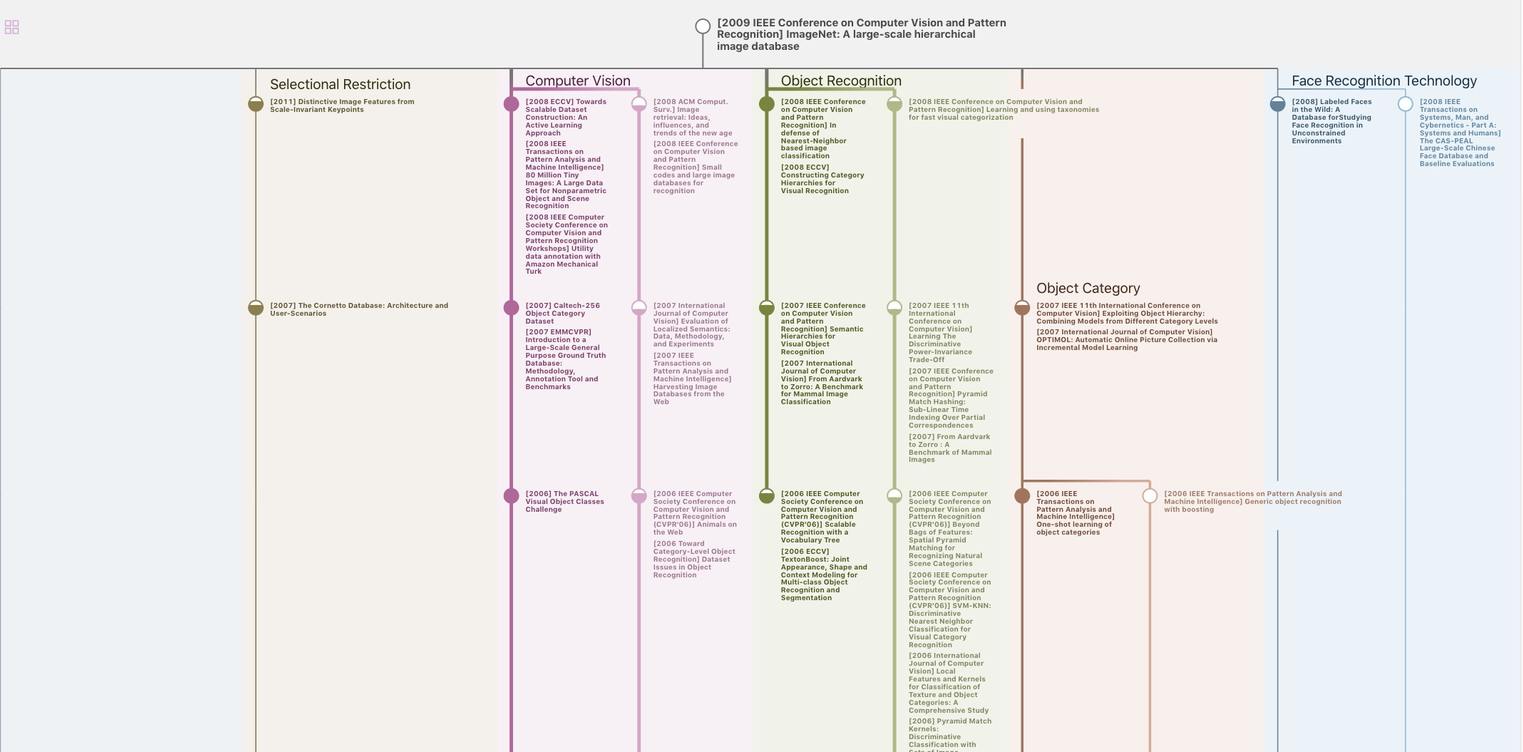
生成溯源树,研究论文发展脉络
Chat Paper
正在生成论文摘要