Correlation-Based Machine Learning Techniques for Channel Estimation with Fluid Antennas
ICASSP 2024 - 2024 IEEE International Conference on Acoustics, Speech and Signal Processing (ICASSP)(2024)
摘要
Fluid antennas (FAs) enable the reconfigurability of systems by moving between predefined locations, known as "ports". This is a promising concept for sixth generation (6G) mobile communications. To make full advantage of FAs, each port needs to estimate the channel, which requires enormous estimation overhead. We study how machine learning techniques can recover the complete channel from measurements at a few predefined ports. The stability of correlation between ports is crucial to the robustness of the network. However, the correlation varies in practice as the propagation environment changes. To address this challenge, we design dedicated sub-networks for different correlation conditions instead of training a single network for all correlation conditions. We also propose a hard selection approach for these sub-networks, which dynamically adjusting the number of ports that need to be estimated for different correlation conditions. Simulation results show that compared with a single neural network, the proposed hard selection approach achieves comparable performance while saving 17% of the estimation overhead.
更多查看译文
关键词
Fluid antenna,6G,Channel estimation,Machine learning
AI 理解论文
溯源树
样例
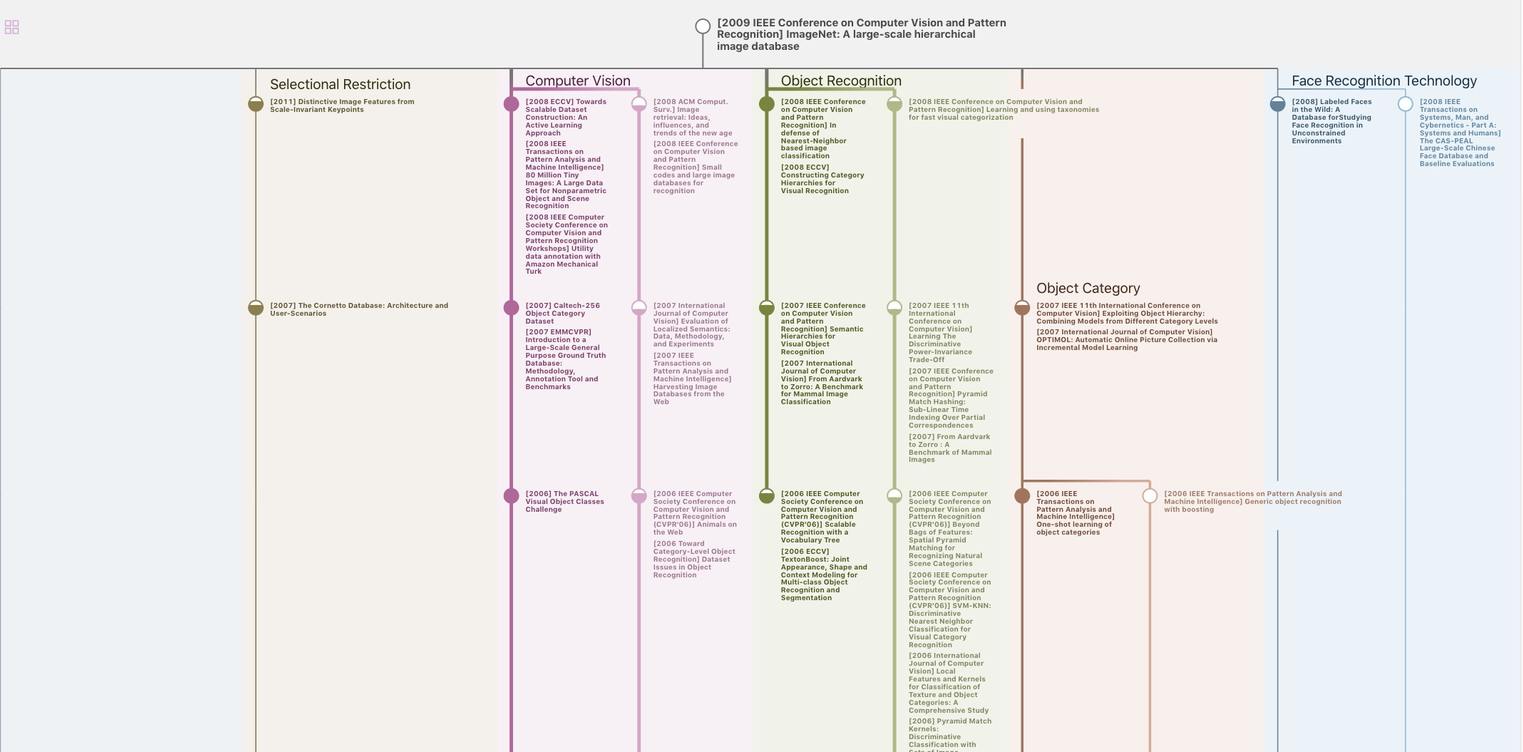
生成溯源树,研究论文发展脉络
Chat Paper
正在生成论文摘要