Unsupervised Pitch-Timbre Disentanglement of Musical Instruments Using a Jacobian Disentangled Sequential Autoencoder
ICASSP 2024 - 2024 IEEE International Conference on Acoustics, Speech and Signal Processing (ICASSP)(2024)
摘要
Disentangled representation learning seeks to align individual dimensions or separate groups of coordinates of latent factors with attributes of observed data such that perturbing certain latent factors uniquely changes particular attributes. A main challenge in unsupervised disentanglement using autoencoders is that strong regularisation, while necessary for consistent disentanglement, comes at the expense of accurate data reconstruction. To address this, we introduce a teacher-student framework that incorporates a variational sequential autoencoder and a Jacobian constraint that regularises the variation of observations relative to latent factors. In real-world audio recordings of musical instruments, our approach outperforms a state-of-the-art method in both sampling quality and unsupervised pitch-timbre disentanglement.
更多查看译文
关键词
Disentangled representation,unsupervised learning,variational autoencoder,music instrument
AI 理解论文
溯源树
样例
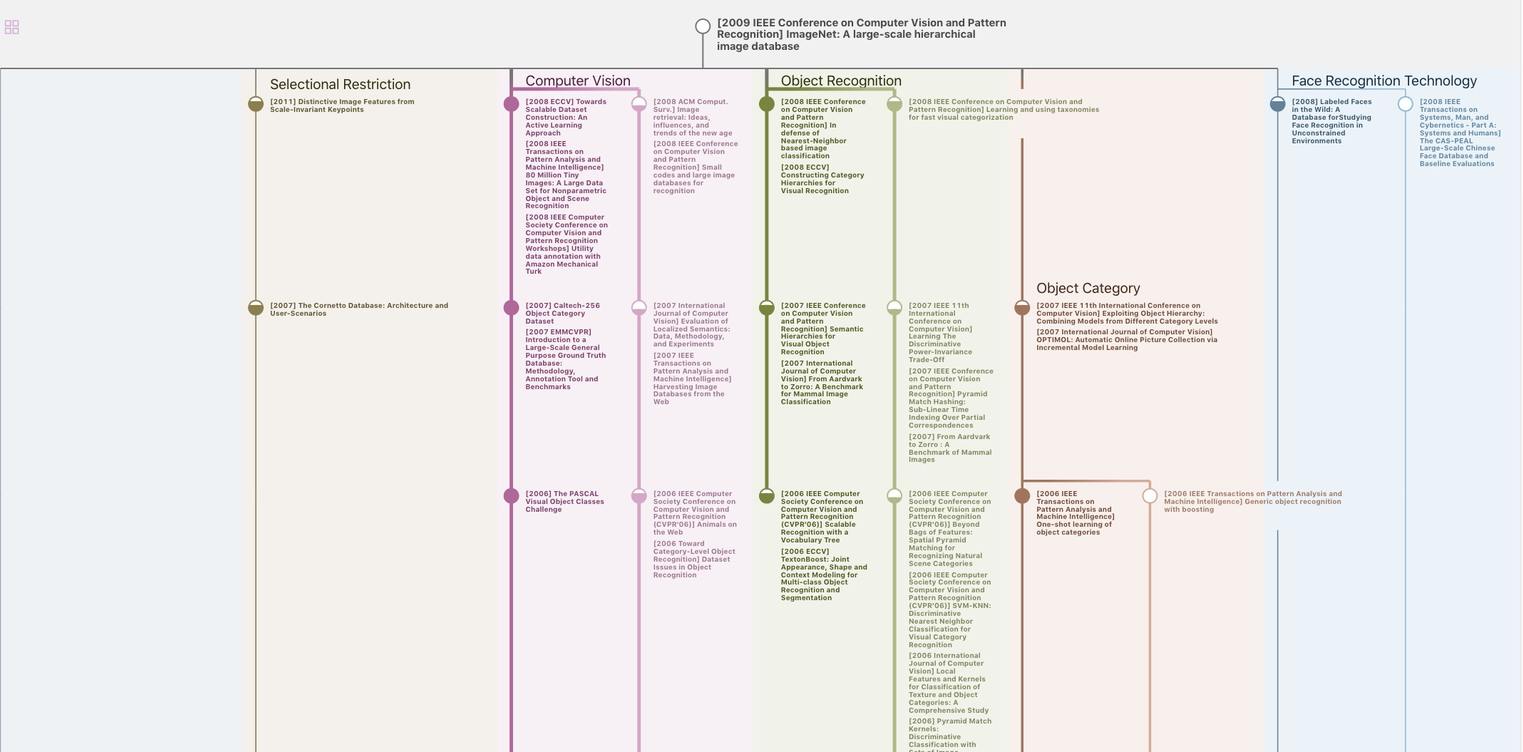
生成溯源树,研究论文发展脉络
Chat Paper
正在生成论文摘要