Enhanced KPI Anomaly Detection: An Unsupervised Hybrid Model with Dynamic Threshold
ICASSP 2024 - 2024 IEEE International Conference on Acoustics, Speech and Signal Processing (ICASSP)(2024)
摘要
Anomaly detection based on key performance indicator (KPI) is an important topic in the field of intelligent operation and maintenance. The problem of insufficient annotated samples is widespread in the industrial Internet, and it severely impairs the performance of data-driven anomaly detection. Previous methods tackled the problem mainly through unsupervised methods, which rely heavily on the ability of the algorithm to extract features. In this work, we propose an unsupervised hybrid model to address these issues. Technically, we capture the long-term dependencies of time series by stacked BiLSTM and use the self-attention mechanism to adaptively select the most noteworthy information in the time series data for global consideration. To effectively distinguish anomalies, we propose a dynamic threshold method that takes into account the context of the data being measured. By doing so, we aim to minimize false positives and missed positives, thus significantly enhancing the overall performance. Extensive experiments on various public benchmarks and real-world measured data demonstrate that our method offers advanced performance and practicality.
更多查看译文
关键词
Key Performance Indicator,Anomaly Detection,Artificial Intelligence for IT Operations
AI 理解论文
溯源树
样例
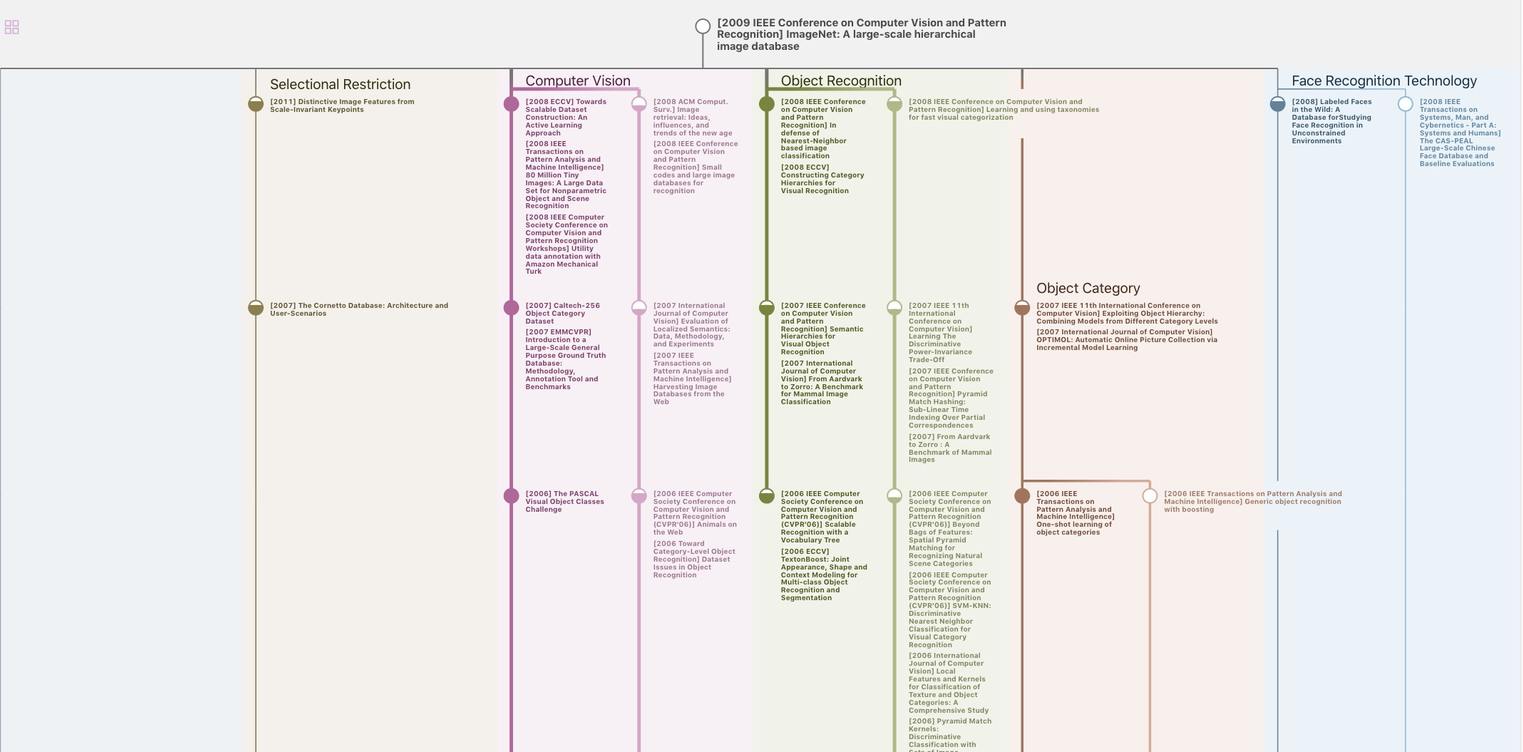
生成溯源树,研究论文发展脉络
Chat Paper
正在生成论文摘要