Communication-Efficient Decentralized Dynamic Kernel Learning
ICASSP 2024 - 2024 IEEE International Conference on Acoustics, Speech and Signal Processing (ICASSP)(2024)
摘要
This paper studies the decentralized dynamic kernel learning problem where each agent in the network receives continuous streaming local data and works collaboratively to learn a non-linear function "on the fly" in a dynamic environment. We utilize the random feature (RF) mapping method to circumvent the curse of dimensionality issue in conventional kernel methods and reformulate the dynamic kernel learning problem as a dynamic parameter optimization problem, which is then efficiently solved by the Decentralized Dynamic Kernel Learning via ADMM (DDKL) framework. To further improve communication efficiency, we incorporate the quantization and censoring strategies in the communication stage and develop the Quantized and Communication-censored DDKL (QC-DDKL) algorithm. We theoretically prove that QC-DDKL can achieve the optimal sublinear regret $\mathcal{O}(\sqrt T )$ over T time slots. Simulation results also corroborate the learning effectiveness and the communication efficiency of the proposed method.
更多查看译文
关键词
Decentralized dynamic kernel learning,RF mapping,ADMM,communication censoring,quantization
AI 理解论文
溯源树
样例
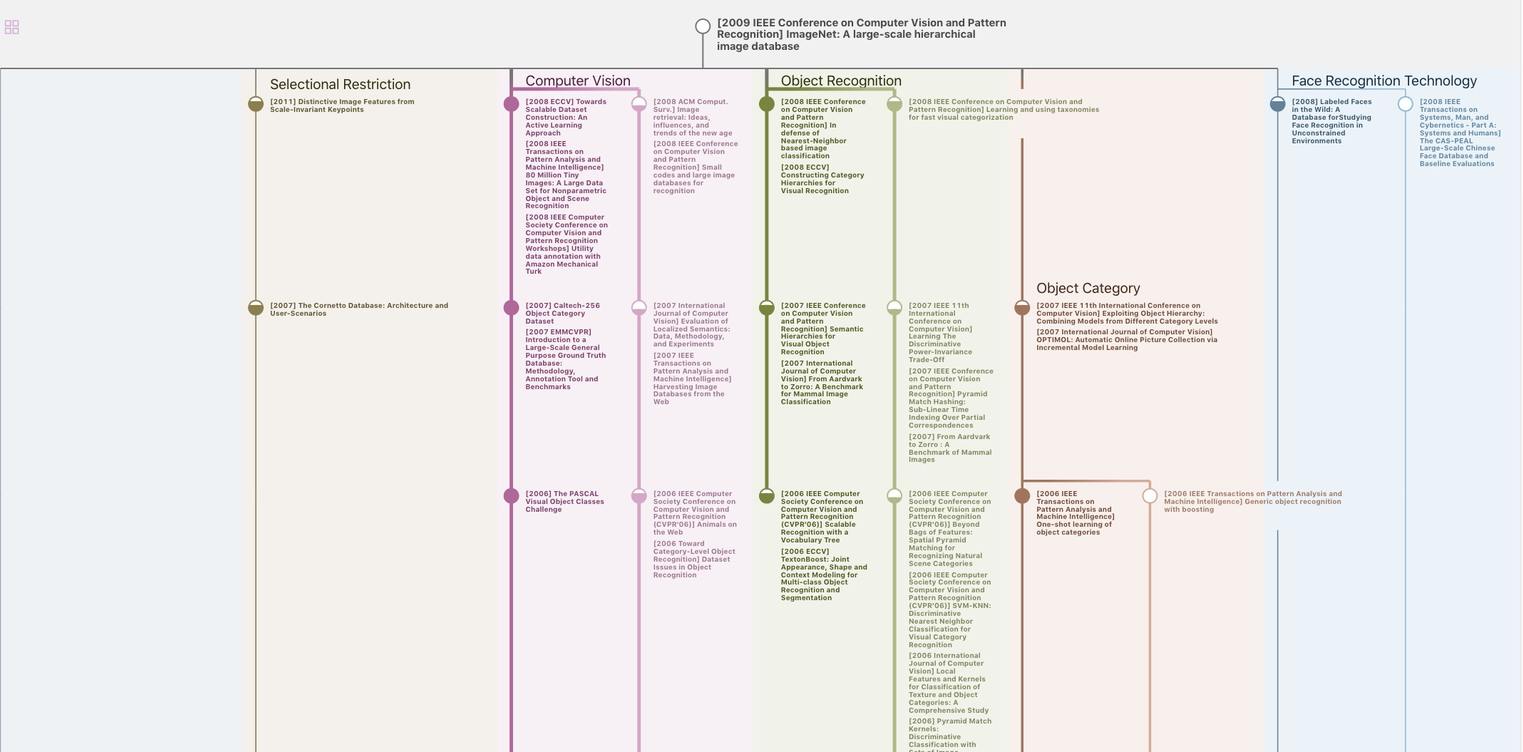
生成溯源树,研究论文发展脉络
Chat Paper
正在生成论文摘要