Rate-Quality Based Rate Control Model for Neural Video Compression
ICASSP 2024 - 2024 IEEE International Conference on Acoustics, Speech and Signal Processing (ICASSP)(2024)
摘要
Rate control (RC) is crucial in achieving stable and smooth bitrate variation in video compression and transmission. Existing RC methods for neural video compression (NVC) have made strong assumptions on solving bit allocation parameters using a pre-defined model, leading to high bit-rate errors (BRE). In response, the study introduces a simple yet effective one-pass RC strategy tailored for NVC frameworks in a plug-in fashion. This strategy consists of two key components: the NVC rate-adaptive model and the associated RC approach. The former model constructs the basis of the latter approach. The proposed RC approach employs a progressive online updating technique for parameter estimation to achieve a lower BRE and maintain the original quality structure of frameworks. Experimental results demonstrate that our approach achieves an impressive RC performance on standard test sequences, outperforming the conventional optimal R-λ RC model with lower BRE and better rate-distortion (R-D) performances. Furthermore, we extend our method to three representative NVC frameworks, consistently showcasing its effectiveness in achieving lower BRE with only moderated R-D performance degradation.
更多查看译文
关键词
Rate control,Neural video compression
AI 理解论文
溯源树
样例
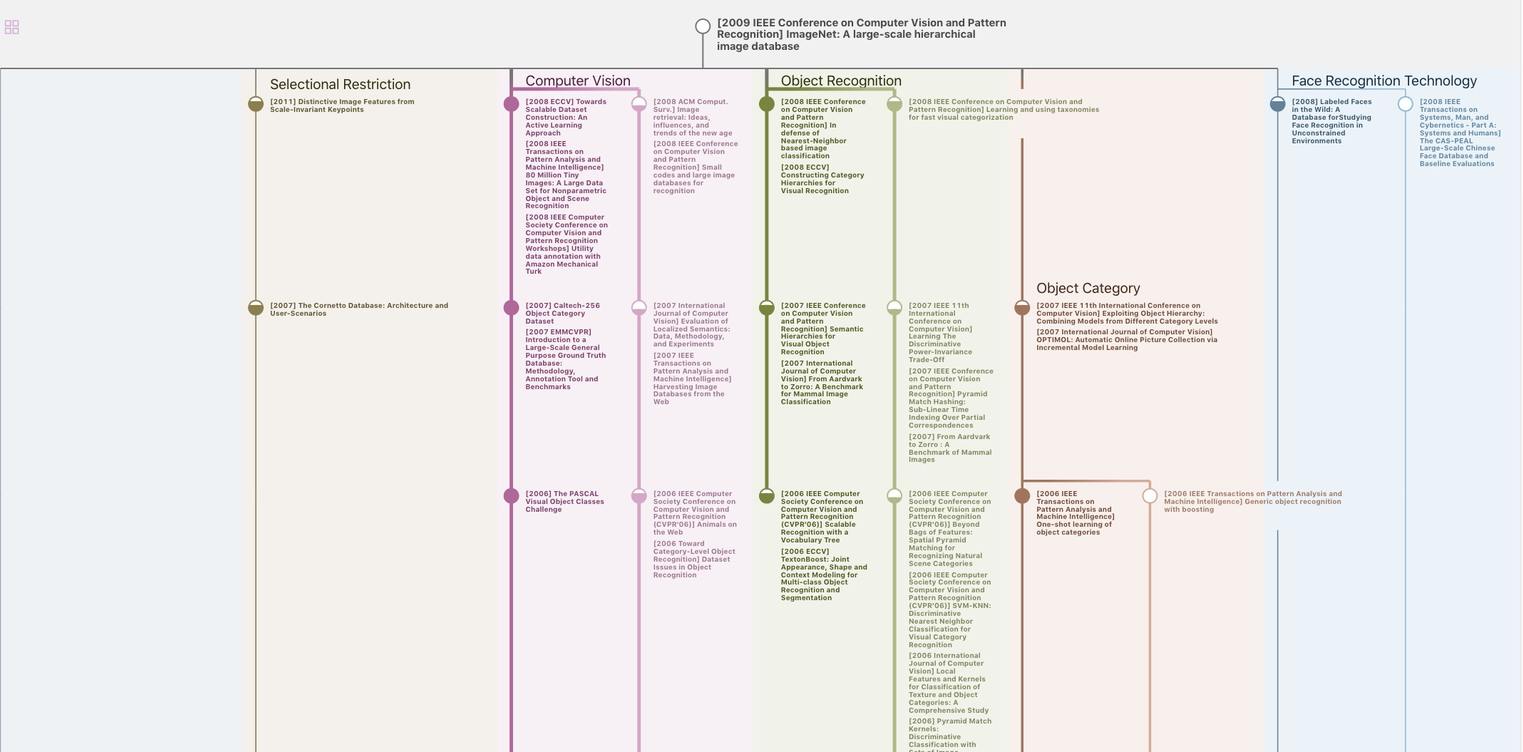
生成溯源树,研究论文发展脉络
Chat Paper
正在生成论文摘要