Small-Footprint Convolutional Neural Network with Reduced Feature Map for Voice Activity Detection
ICASSP 2024 - 2024 IEEE International Conference on Acoustics, Speech and Signal Processing (ICASSP)(2024)
摘要
By using Voice Activity Detection (VAD) as a preprocessing step, hardware-efficient implementations are possible for speech applications that need to run continuously in severely resource-constrained environments. For this purpose, we propose TinyVAD, which is a new convolutional neural network (CNN) model that executes extremely efficiently with a small memory footprint. TinyVAD uses an input pixel matrix partitioning method, termed patchify, to downscale the resolution of the input spectrogram. The hidden layers use a sequence of special convolutional structures with bypass links, referred to as CSPTiny layers. The proposed model is evaluated and compared with previous VAD methods using a diverse set of noisy environmental datasets. TinyVAD executes 3.13 times faster, utilizes only 12.5% as many multiplications, and requires only 13.0% as many parameters when compared to the previous state-of-the-art.
更多查看译文
关键词
voice activity detection,convolutional neural network,resource efficiency,low latency
AI 理解论文
溯源树
样例
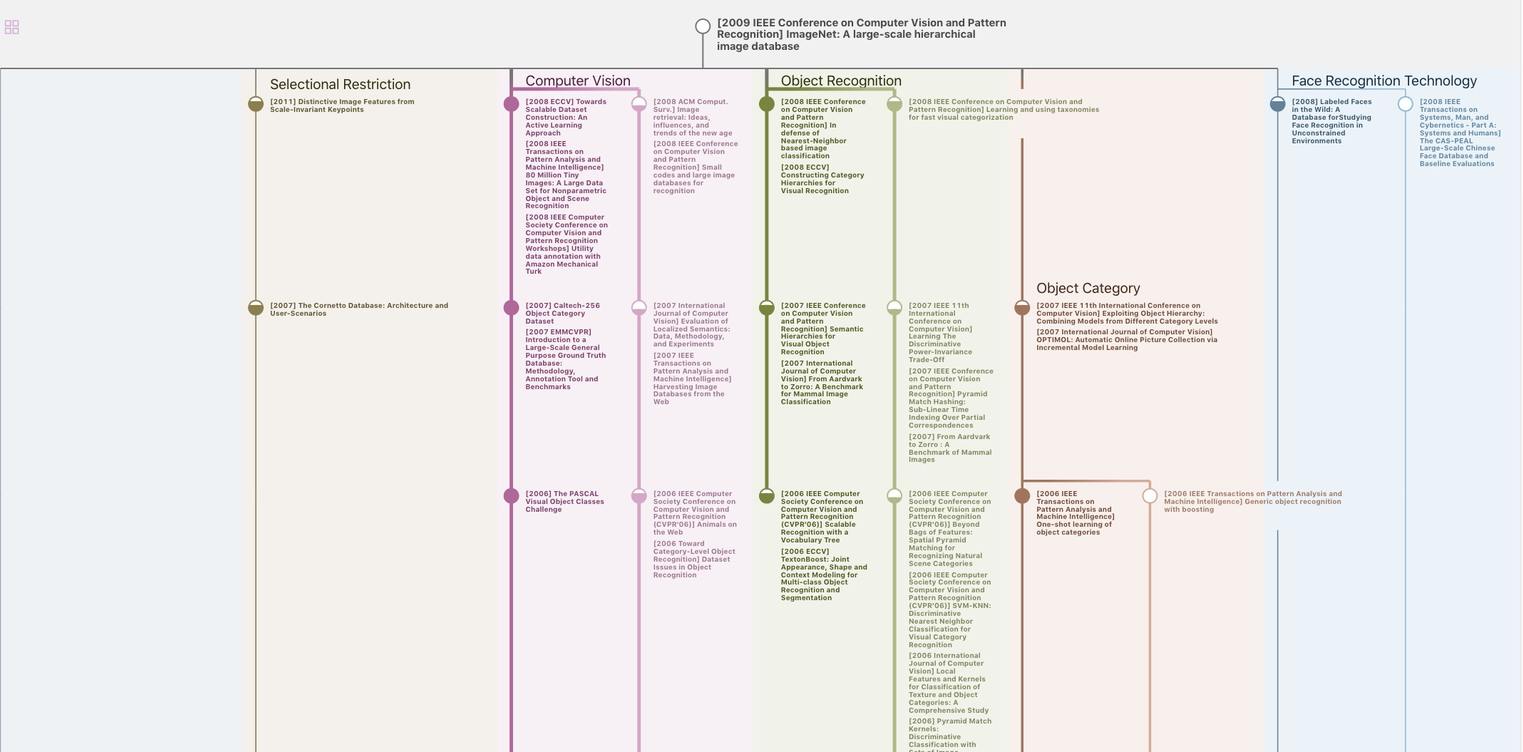
生成溯源树,研究论文发展脉络
Chat Paper
正在生成论文摘要