Power-Aware Task-Based Learning of Neuromorphic ADCs
ICASSP 2024 - 2024 IEEE International Conference on Acoustics, Speech and Signal Processing (ICASSP)(2024)
摘要
Analog-to-digital converters (ADCs) are key components in digital signal processing systems. Traditional ADCs are designed to accurately represent analog signals. Emerging technologies, such as neuromorphic ADCs, allow tuning the ADC mapping on the device, possibly adapting it to the system task or power considerations. In this work, we study such task-based acquisition using neuromorphic ADCs while jointly accounting for power minimization as well as a generic classification task. We propose a physically compliant model based on resistive successive approximation register ADCs, integrated with memristor components, that can be adjusted to modify the quantization regions. We propose a data-driven algorithm that jointly tunes the neuromorphic ADC along with the digital and analog processing. Our numerical studies demonstrate the efficacy of our design compared to traditional uniform ADCs, simultaneously improving accuracy by up to 1.5× while reducing power consumption by as much as 75%.
更多查看译文
关键词
neuromorphic,task-based ADCs,memristors,deep learning,power efficiency
AI 理解论文
溯源树
样例
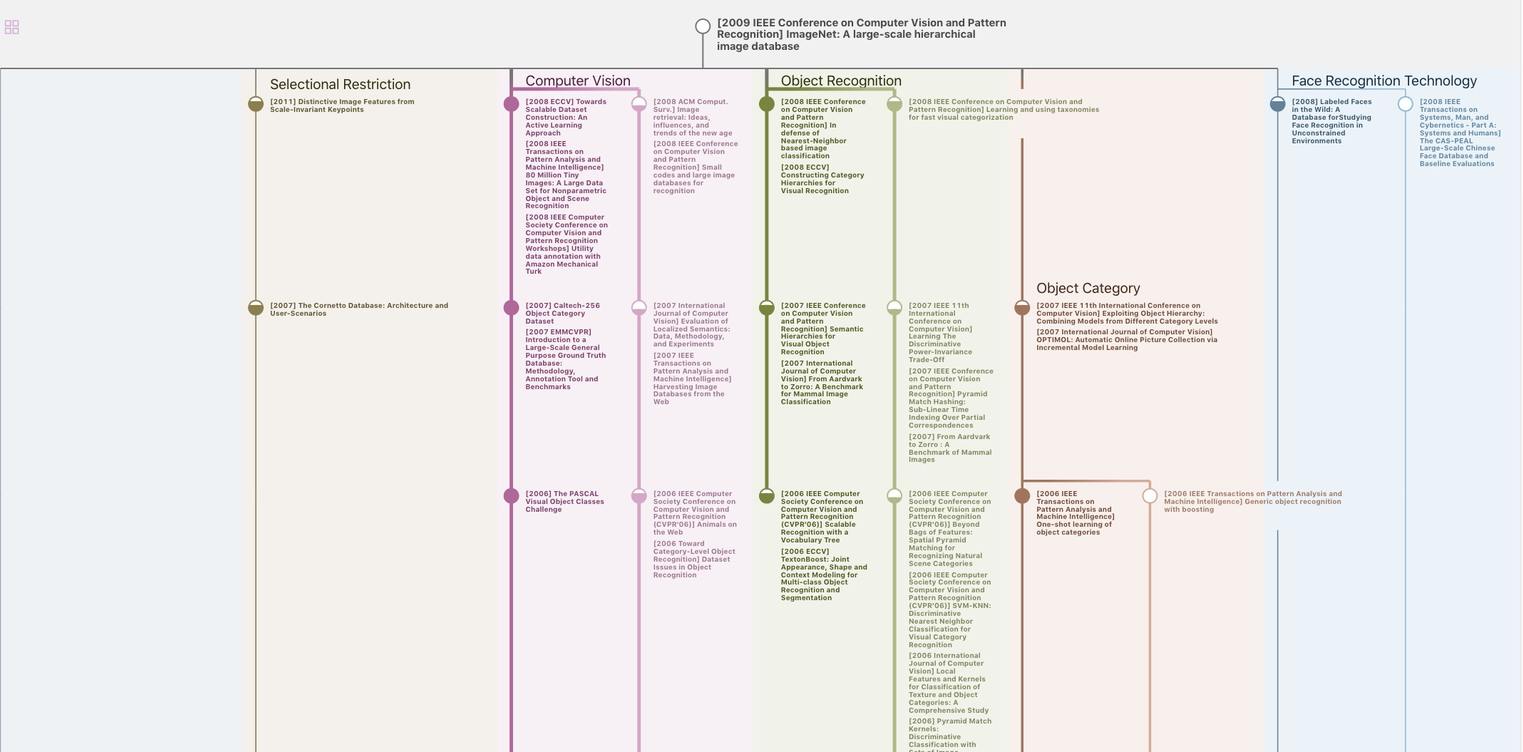
生成溯源树,研究论文发展脉络
Chat Paper
正在生成论文摘要