Constrained Risk-Sensitive Deep Reinforcement Learning for eMBB-URLLC Joint Scheduling
IEEE Transactions on Wireless Communications(2024)
摘要
In this work, we employ a constrained risk-sensitive deep reinforcement learning (CRS-DRL) approach for joint scheduling in a dynamic multiplexing scenario involving enhanced mobile broadband (eMBB) and ultra-reliable low-latency communications (URLLC). Our scheduling policy minimizes the adverse impact of URLLC puncturing on eMBB users while satisfying URLLC requirements. Conventional DRL-based algorithms for eMBB/URLLC scheduling prioritize maximizing the expected return. However, for URLLC mission-critical applications, it is crucial to explicitly avoid catastrophic scheduling failures associated with the long tail of the reward distribution. Therefore, robust management of such uncertainties and risks is imperative. Our proposed CRS-DRL algorithm incorporates the conditional Value-at-Risk (CVaR) as the risk criterion for optimization. A URLLC queuing mechanism is considered to decrease the URLLC drops and increase eMBB throughput compared to the instant scheduling policy. Our architecture is based on the actor-critic model but considers a transfer function to obtain feasible solutions of the unconstrained actor network, and the critic predicts the entire distribution over future returns instead of simply the expectation. Numerical results indicate that our CRS-DRL algorithm, under varying CVaR levels, achieves similar expected returns but reduces long-tail behavior for long-term rewards compared to the risk-neutral approach.
更多查看译文
关键词
eMBB,deep reinforcement learning,punctured scheduling,resource allocation,risk-sensitive,URLLC
AI 理解论文
溯源树
样例
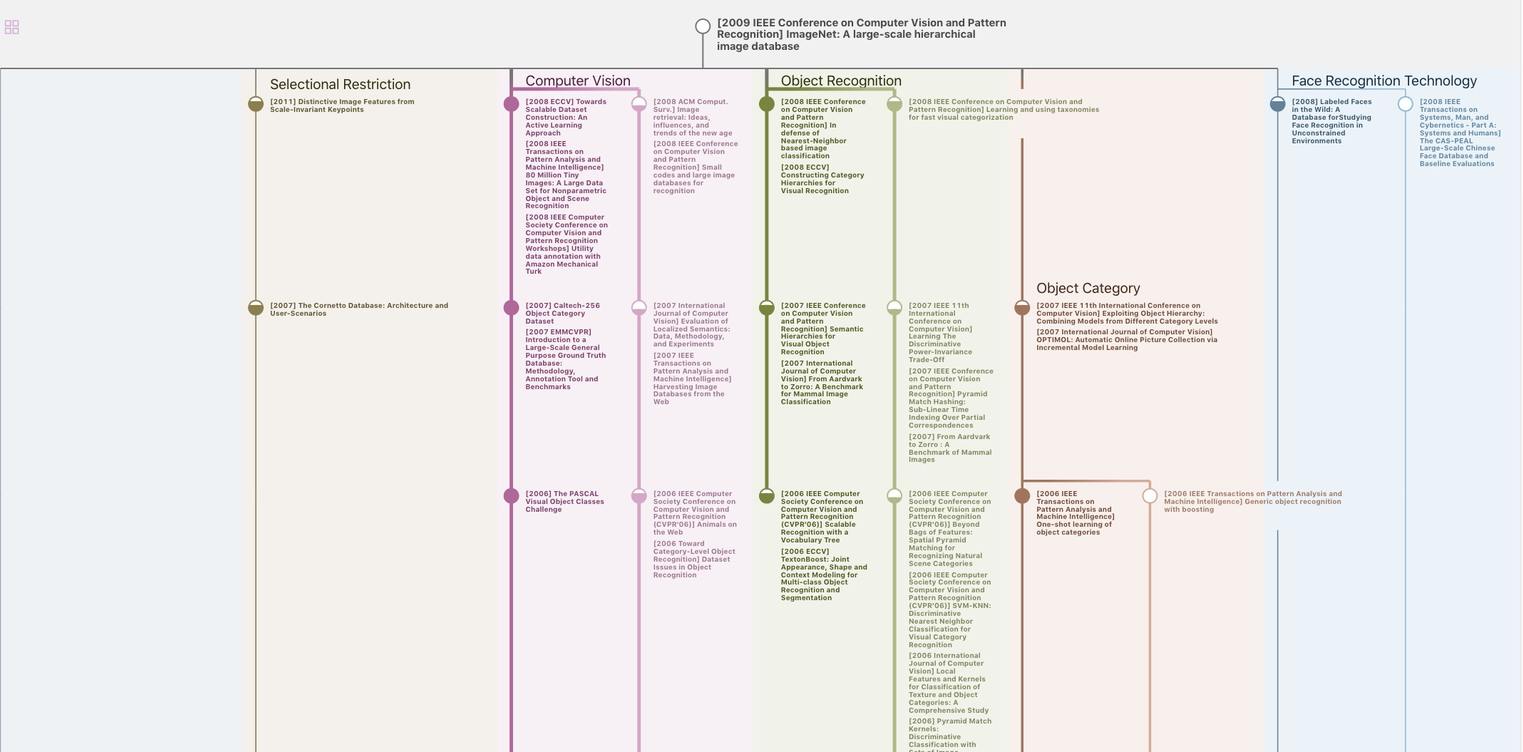
生成溯源树,研究论文发展脉络
Chat Paper
正在生成论文摘要