A Dual-Scale Transformer-Based Remaining Useful Life Prediction Model in Industrial Internet of Things
IEEE Internet of Things Journal(2024)
摘要
With recent advents of industrial Internet of Things (IIoT), the connectivity and data collection capabilities of industrial equipment have be significantly enhanced, yet bringing new challenges for the remaining useful life (RUL) prediction. To fulfill the RUL predicting demand in multivariate time series, this work proposes an encoder-decoder model termed as dual-scale transformer model (DSFormer), built upon the Transformer architecture. First, in the encoder part, a dual-attention module is designed for the weight feature extraction from both dimensions of the sensor and time series, aiming to compensate for the diverse impacts of different sensors on the prediction. Next, a temporal convolutional network (TCN) module is introduced to capture sequence features and alleviate the loss of positional information incurred by stacking blocks. Then, the feature decomposition module is integrated into the decoder for trend feature extraction from sequences, providing the model with additional sequence information. Finally, compared to existing models, the proposed method can obtain the superior performance in terms of the root mean square error (RMSE) and Score metrics on the FD001, FD002 and FD003 subsets of the C-MAPSS dataset, with an average improvement of 3.2% and 2.5% respectively. In particular, the ablation experiment further validates the effectiveness of proposed modules in handling multivariate time series and extracting features.
更多查看译文
关键词
Industrial Internet of Things (IIoT),multi-sensor data,remaining useful life (RUL),attention mechanism,Transformer
AI 理解论文
溯源树
样例
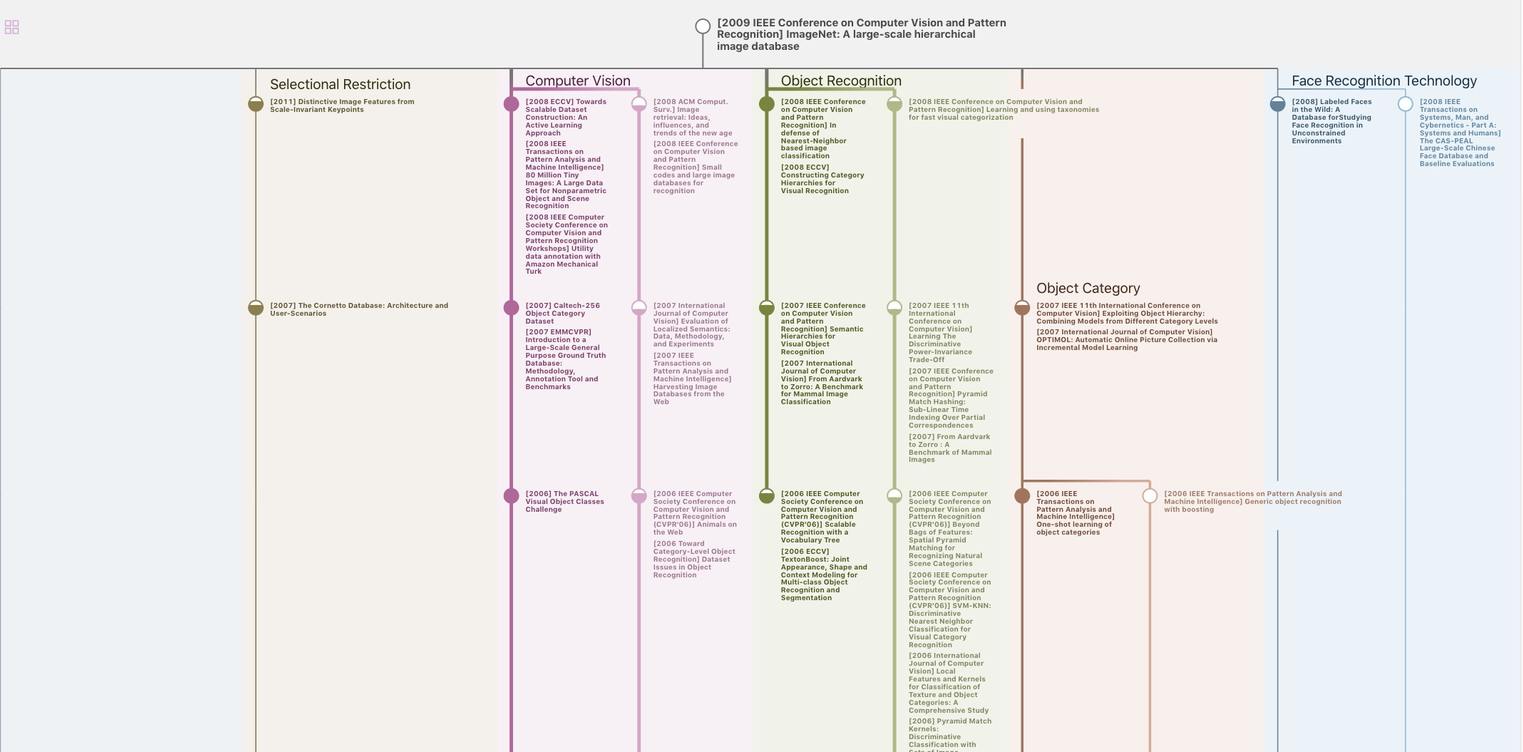
生成溯源树,研究论文发展脉络
Chat Paper
正在生成论文摘要