Incomplete Multi-View Clustering Via Inference and Evaluation
ICASSP 2024 - 2024 IEEE International Conference on Acoustics, Speech and Signal Processing (ICASSP)(2024)
摘要
Multi-view clustering aims to improve the clustering performance by leveraging information from multiple views. Most existing works assume that all views are complete. However, samples in real-world scenarios cannot be always observed in all views, leading to the challenging problem of Incomplete Multi-View Clustering (IMVC). Although some attempts are made recently, they still suffer from the following two limitations: (1) they usually adopt shallow models, which are unable to sufficiently explore the consistency and complementary of multiple views; (2) they lack of a suitable measurement to evaluate the quality of the recovered data during the learning process. To address the aforementioned limitations, we introduce a novel Incomplete Multi-View Clustering via Inference and Evaluation (IMVC-IE). Specifically, IMVC-IE adopts the contrastive learning strategy on features of different views to excavate the underlying information from existing samples firstly. Subsequently, massive alternative simulated data are inferred for missing views and a novel evaluation strategy is presented to obtain the proper data for missing views completion. Extensive experiments are conducted and verify the effectiveness of our method.
更多查看译文
关键词
Incomplete multi-view clustering,missing data inference,data evaluation
AI 理解论文
溯源树
样例
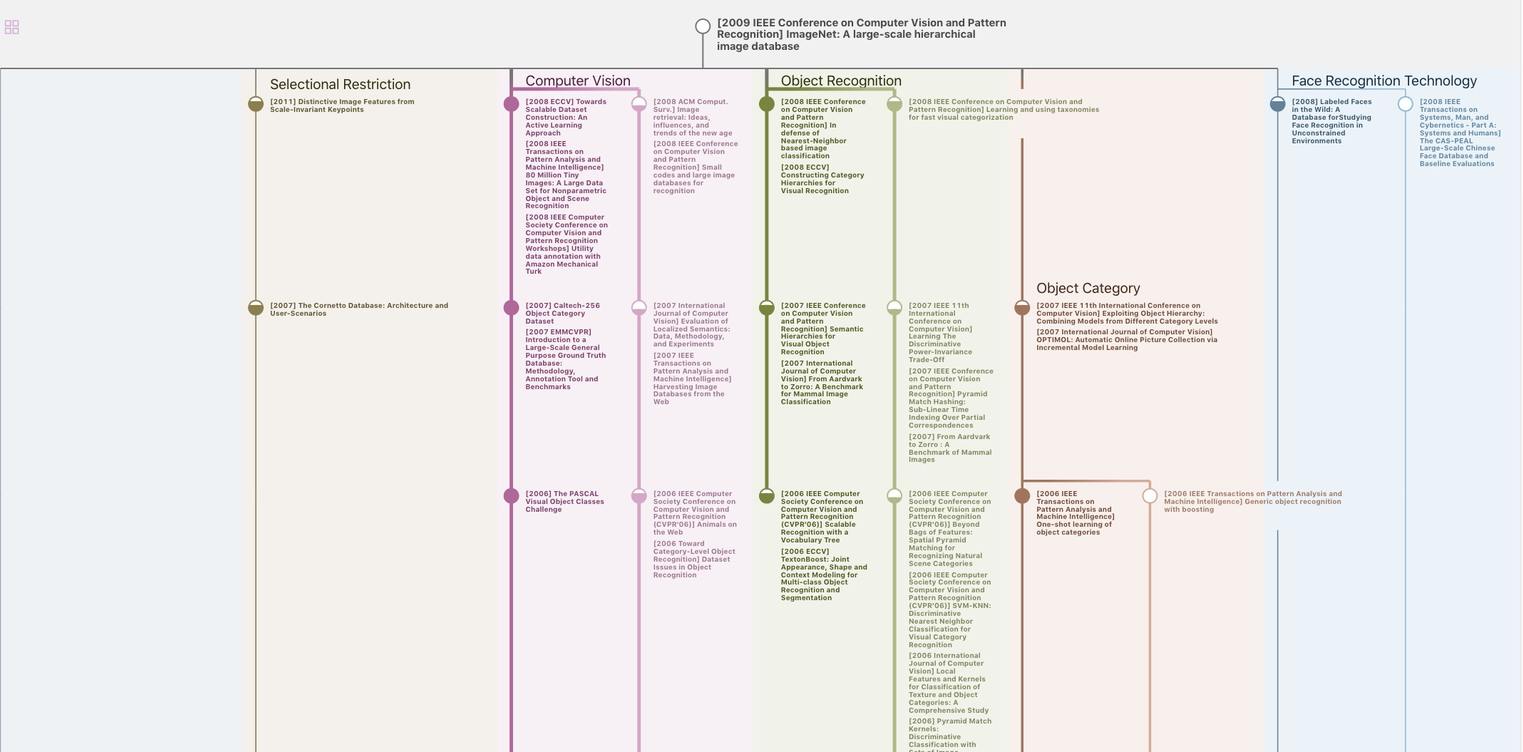
生成溯源树,研究论文发展脉络
Chat Paper
正在生成论文摘要