Leveraging Large Pretrained Models for Line-by-Line Spoken Program Recognition
ICASSP 2024 - 2024 IEEE International Conference on Acoustics, Speech and Signal Processing (ICASSP)(2024)
摘要
Spoken programming languages significantly differ from natural English due to the inherent variability in speech patterns among programmers and the wide range of programming constructs. In this paper, we employ Wav2Vec 2.0 to enhance the accuracy of transcribing spoken programming languages like Java. Adapting a model with just one hour of spoken programs that had prior exposure to a substantial amount of natural English-labeled data, we achieve a word error rate (WER) of 8.7%, surpassing the high 28.4% WER of a model trained solely on natural English. Decoding with a domain-specific N-gram model and subsequently rescoring the N-best list with a fine-tuned large language model tailored to the programming domain resulted in a WER of 5.5% on our test set.
更多查看译文
关键词
low resource speech recognition,large pre-trained models,voice programming,language modeling
AI 理解论文
溯源树
样例
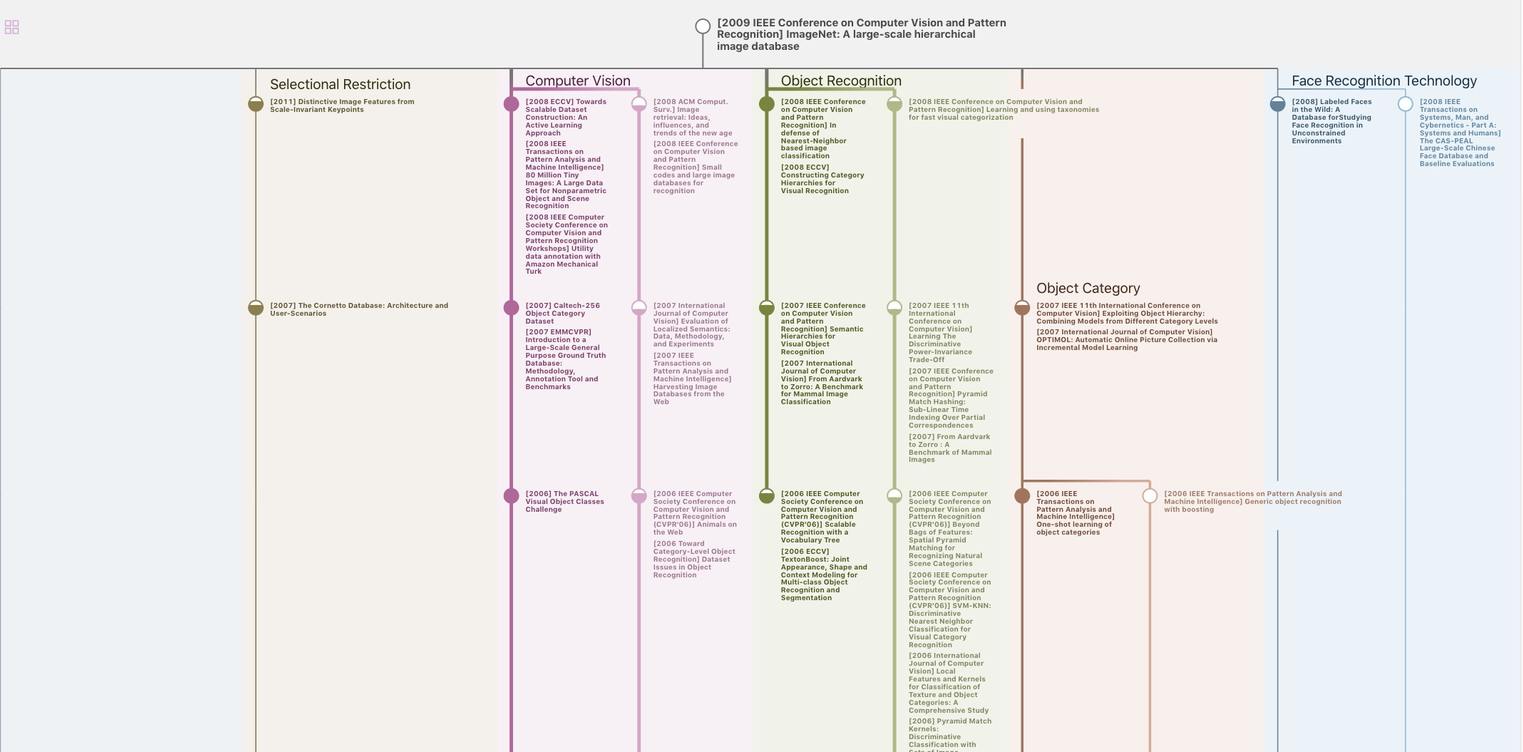
生成溯源树,研究论文发展脉络
Chat Paper
正在生成论文摘要