A Lightweight Small Object Detection Method Based On Multi-Layer Coordination Federated Intelligence for Coal Mine IoVT
IEEE Internet of Things Journal(2024)
摘要
Video surveillance as an important function of internet of video things (IoVT) system has been widely used in coal mine monitoring for coal mine safety with excellent results, however, there are still many shortcomings: 1) Existing coal mine IoVT systems have limited detection accuracy for small-sized objects; 2) Coal mine video surveillance systems generally adopt centralized cloud computing, transmission of massive data causes high latency, which seriously affects the response speed of object detection function; 3) The concept drift caused by the data stream seriously affect the detection effect of the offline algorithm. To address the above issues, we propose a small object detection method based federated intelligence to assist coal mine IoVT for object detection. First, we design a lightweight neural network Rep-ShuffleNet to improve YOLOv8, the state-of-the-art YOLO algorithm, to maintain high detection accuracy while dramatically increasing the inference speed, and with the advantage of lightweight, it can be deployed to embedded devices for low-latency edge computing; Moreover, we design a federated learning-based MLC-FL algorithm for local algorithms’ automatic and efficient optimization by asynchronous communication and data interaction reduction strategy. The experimental results show that with the assistance of federated intelligence model optimization strategies, the lightweight YOLOv8 has excellent detection performance (mAP: 94.6%, APsmall: 86.7%, FPS: 21.6), thus to assist coal mine IoVT to realize accurate and real-time underground small object detection.
更多查看译文
关键词
Internet of Video Things,Edge computing,Federated learning,Small object detection,Lightweight model
AI 理解论文
溯源树
样例
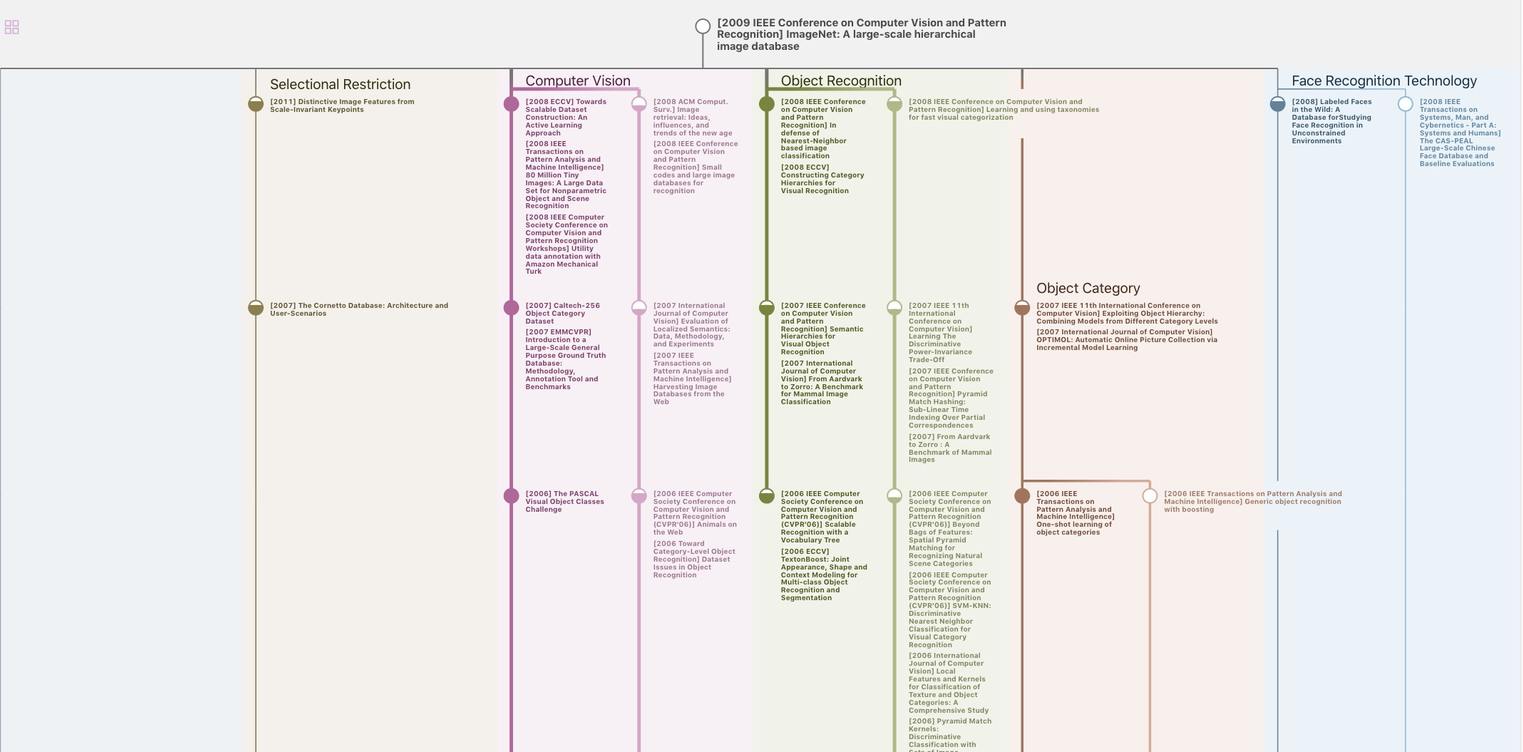
生成溯源树,研究论文发展脉络
Chat Paper
正在生成论文摘要