Modeling Quasi-Periodic Dependency via Self-Supervised Pre-Training for Respiratory Sound Classification
ICASSP 2024 - 2024 IEEE International Conference on Acoustics, Speech and Signal Processing (ICASSP)(2024)
摘要
Despite the success of self-supervised respiratory sound classification methods, they do not consider that respiratory sounds are quasi-periodic signals with repetitive patterns in successive breaths, which is vital for distinguishing respiratory sounds from non-quasi-periodic sounds like noises. Therefore, the existing methods may achieve limited improvement due to ignoring the quasi-periodic dependency. To this end, considering that the segments containing the same respiratory sound pattern should be similar in a sample, we extract the segment-wise representations and evaluate the similarity between the periodic-dependent representations via a sparse self-relation matrix. By defining a periodic consistency loss, we push the sparse self-relation matrixes of two clips of the same sample closer, encouraging a larger similarity between the representations. In this manner, the method can focus more on the respiratory sound-related quasi-periodic patterns that repeatedly recur in the periodic-dependent segments. Taking HF_Lung_V1 and COVID-19 Sounds as pre-training sets, the method exceeds the baseline by 7.67% on the ICBHI 2017 classification task.
更多查看译文
关键词
Respiratory sound classification,self-supervised learning,quasi-periodic dependency
AI 理解论文
溯源树
样例
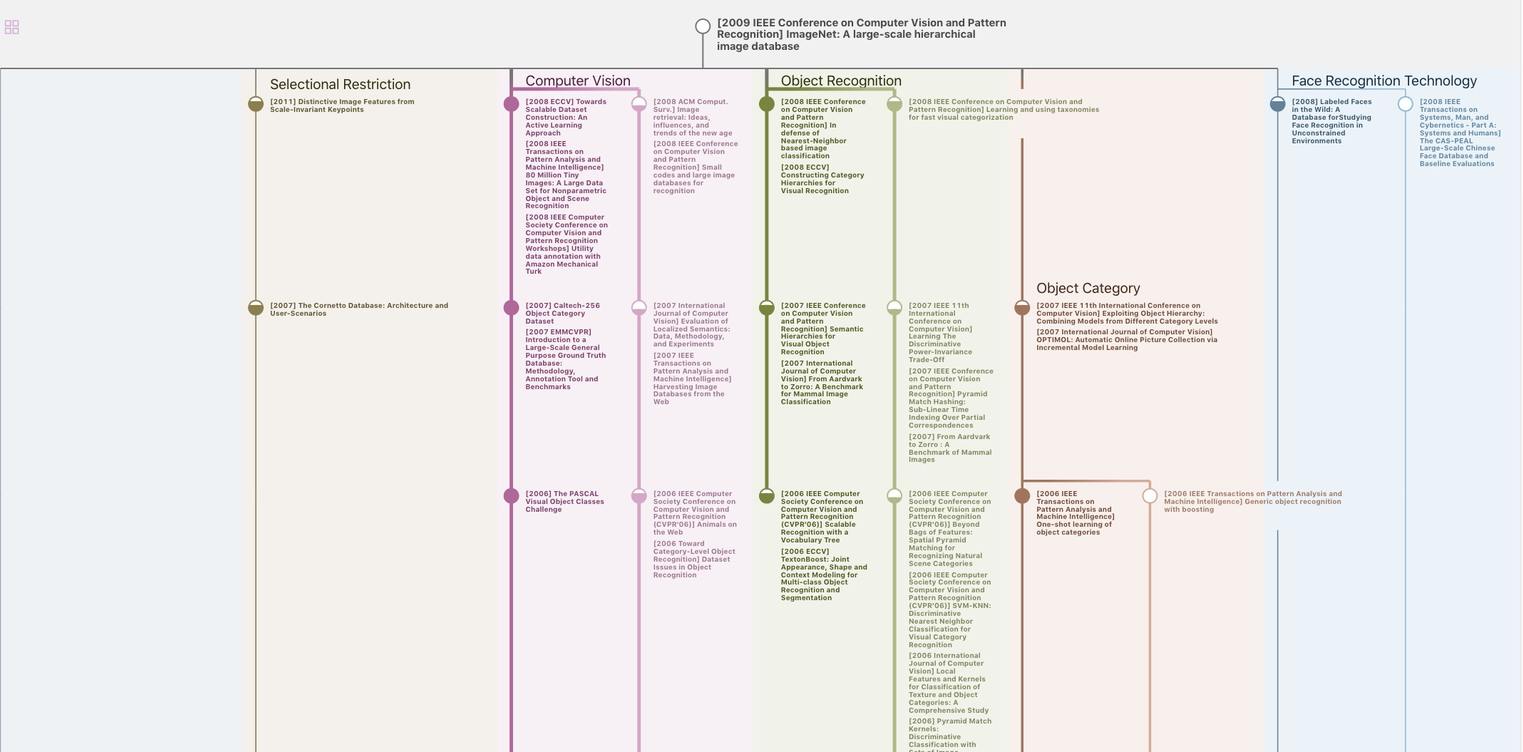
生成溯源树,研究论文发展脉络
Chat Paper
正在生成论文摘要