Analysis of High-Order Brain Networks Resolved in Time and Frequency Using CP Decomposition
ICASSP 2024 - 2024 IEEE International Conference on Acoustics, Speech and Signal Processing (ICASSP)(2024)
摘要
To capture different aspects of a complex system, the modeling approach should be able to take these effectively into consideration. Two aspects of the human brain we are quite interested in are its interconnected nature and its dynamism. One modeling approach that can capture these two aspects is based on networks that change with time and go beyond pairwise interactions. Partly because of the size of these temporal high-order networks, analyzing and visualizing them is quite a challenge. In this work, we propose a pipeline based on canonical polyadic (CP) decomposition to analyze high-order networks that are resolved in both time and frequency estimated from resting-state functional magnetic resonance imaging (fMRI) data. We show that we can combine different subjects' information into a common frame of reference for comparison. We also show that different factors provide different patterns that are easy to visualize and interpret. To the best of our knowledge, this is the first work that has proposed a pipeline for analyzing different subjects' brain networks while also incorporating temporal and spectral information about high-order interactions.
更多查看译文
关键词
fMRI,tensor decomposition,canonical polyadic decomposition,high-order networks,Time-frequency analysis
AI 理解论文
溯源树
样例
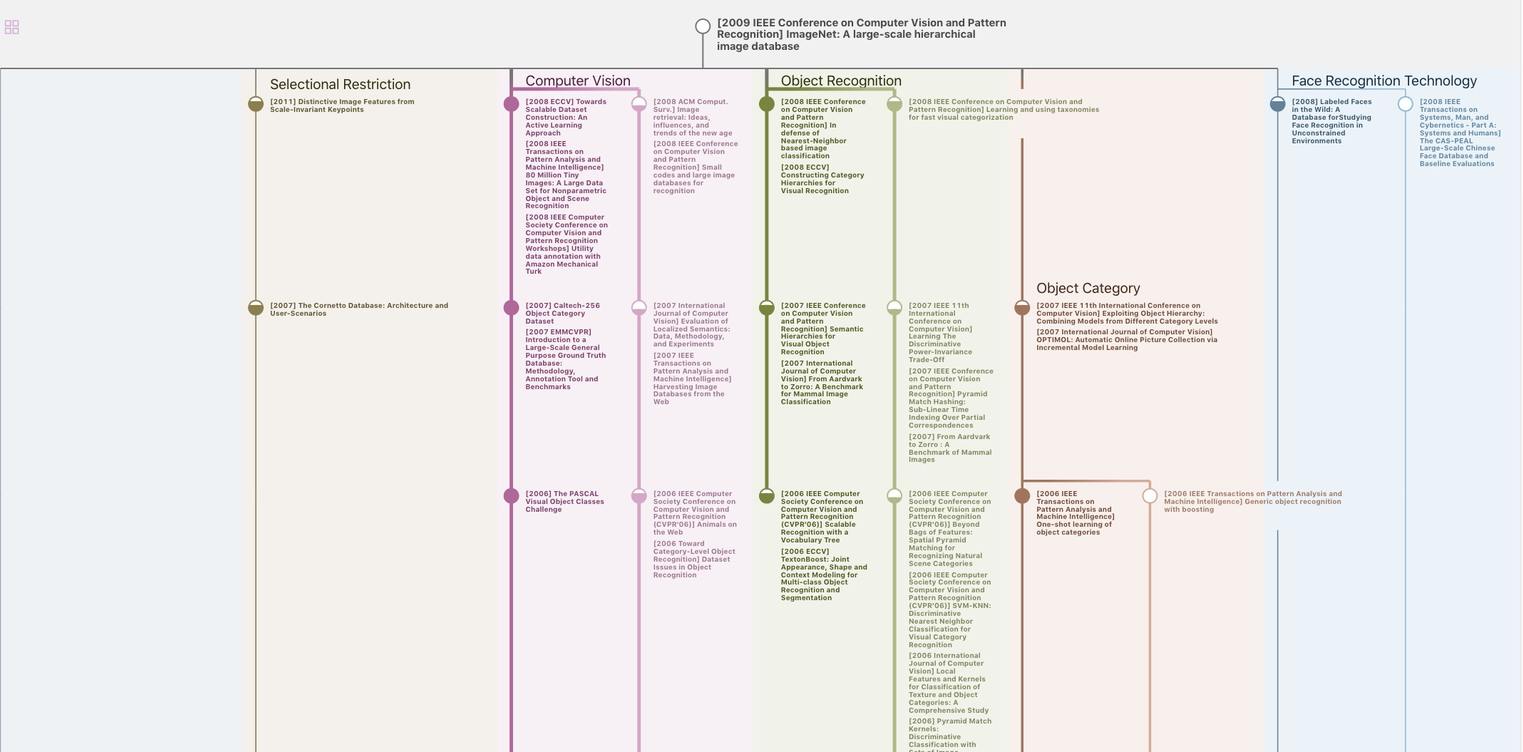
生成溯源树,研究论文发展脉络
Chat Paper
正在生成论文摘要