Parallel Multi-Objective Evolutionary Algorithm for Constrained Multi-Objective Optimization.
Arab Conference on Information Technology(2023)
摘要
Most real-world problems aim at achieving multiple objectives under a pool of constraints. Generally, the objectives of this problem category are contradictory. These problems are modeled as constrained multi-objective optimization problems (CMOPs). Solving a CMOP leads to finding an optimal solution, which trade-offs between the conflicting objectives respecting a set of constraints. Constrained multi-objective evolutionary algorithms (CMOEA) are a suitable class of based-evolutionary algorithms for finding a solution to CMOP problems. Finding an optimal solution for a large-scale problem with CMOEAs represents a time-consuming task, and the search process may lead to premature convergence. Exploiting parallel technologies is an omnipresent solution for improving the performance of CMOEAs without deteriorating the solutions' quality. In this paper, a paralleled version of a recent CMOEA algorithm named constrained multi-objective optimization evolutionary algorithms based on decomposition and directed mating (CMOEA/D-DMA), is proposed (PCMOEA/D-DMA) based on a multi-population mechanism and implemented under a synchronous master-slave parallel model. Based on the hypervolume metric and execution time, a well-known CMOP (mCDTLZ) is used for experimenting with the proposed PCMOEA/D-DMA and comparing it with the sequential CMOEA/D-DMA. Results show that PCMOEA/D-DMA outperforms the sequential CMOEA/D-DMA regarding execution time metric.
更多查看译文
关键词
Constrained multi-objective optimization problems,constrained MOEA,directed mating,archives of infeasible solutions,multi-population mechanism,parallelism,synchronous master-slave parallel model
AI 理解论文
溯源树
样例
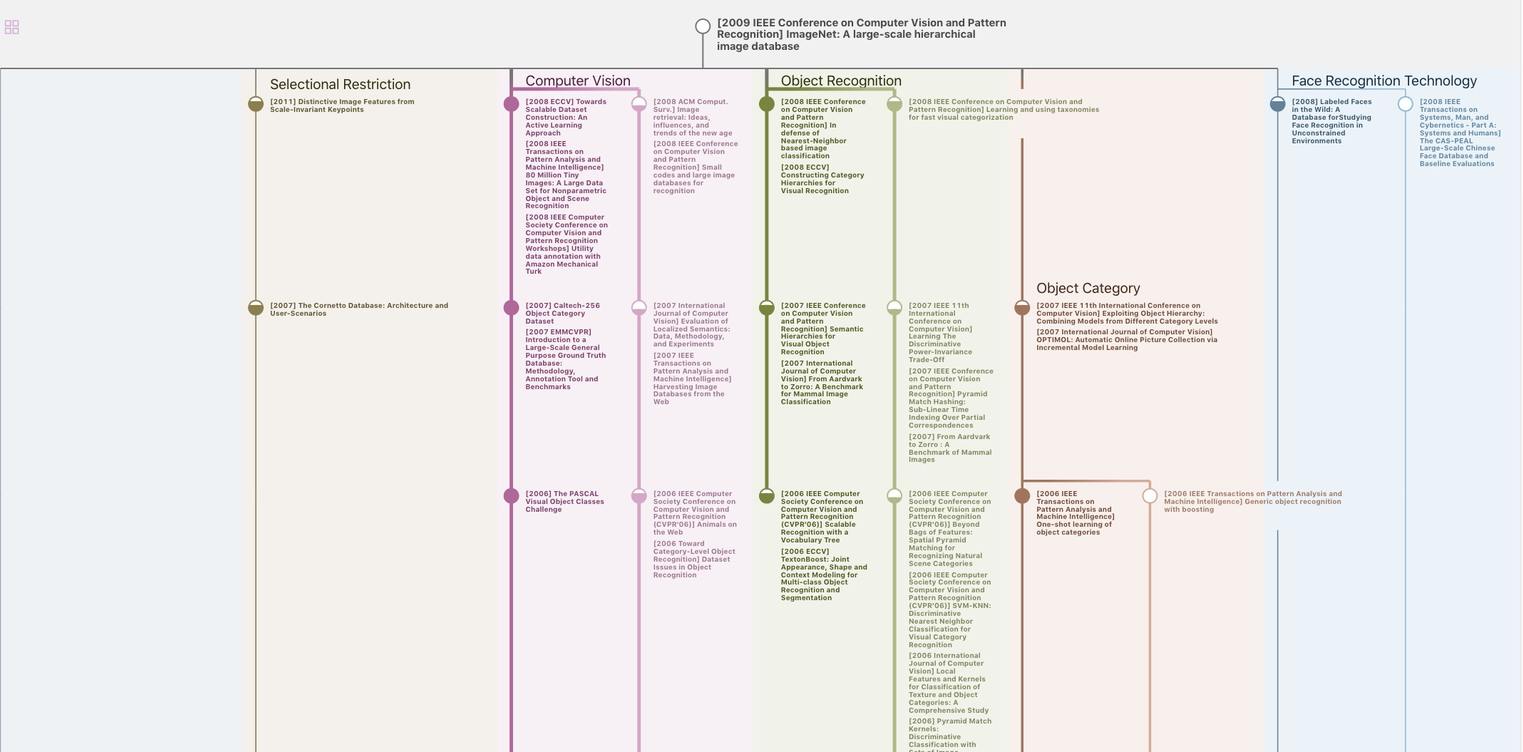
生成溯源树,研究论文发展脉络
Chat Paper
正在生成论文摘要