A Counterfactual Inspired Framework For Quantifying Edge Effects On Gnns Fairness
ICASSP 2024 - 2024 IEEE International Conference on Acoustics, Speech and Signal Processing (ICASSP)(2024)
摘要
Graph Neural Networks (GNNs) play a pivotal role in graph representation learning, addressing challenges across diverse applications. Despite their significance, data-driven GNNs often overlook biases, raising fairness concerns. Inspired by counterfactuals, we inquire, ’In graph data, how does removing an edge affect model fairness?’ Existing edge fairness measures lack interpretability. Our focus is on exploring how each edge influences model fairness. We introduce the Movement of Edge Weight (MEW) framework, establishing an interpretable estimation of the influence of each edge on model fairness. Specifically, we improve the interpretability of Probabilistic Distribution Disparity (PDD) as a fairness metric when removing edges by extending the chain rule. To achieve effective model debiasing, we propose deleting the top k training edges with the most significant impact on model bias. Experimental results affirm the effectiveness of our method, demonstrating excellent performance.
更多查看译文
关键词
Fairness,Graph Neural Networks,Counterfactual
AI 理解论文
溯源树
样例
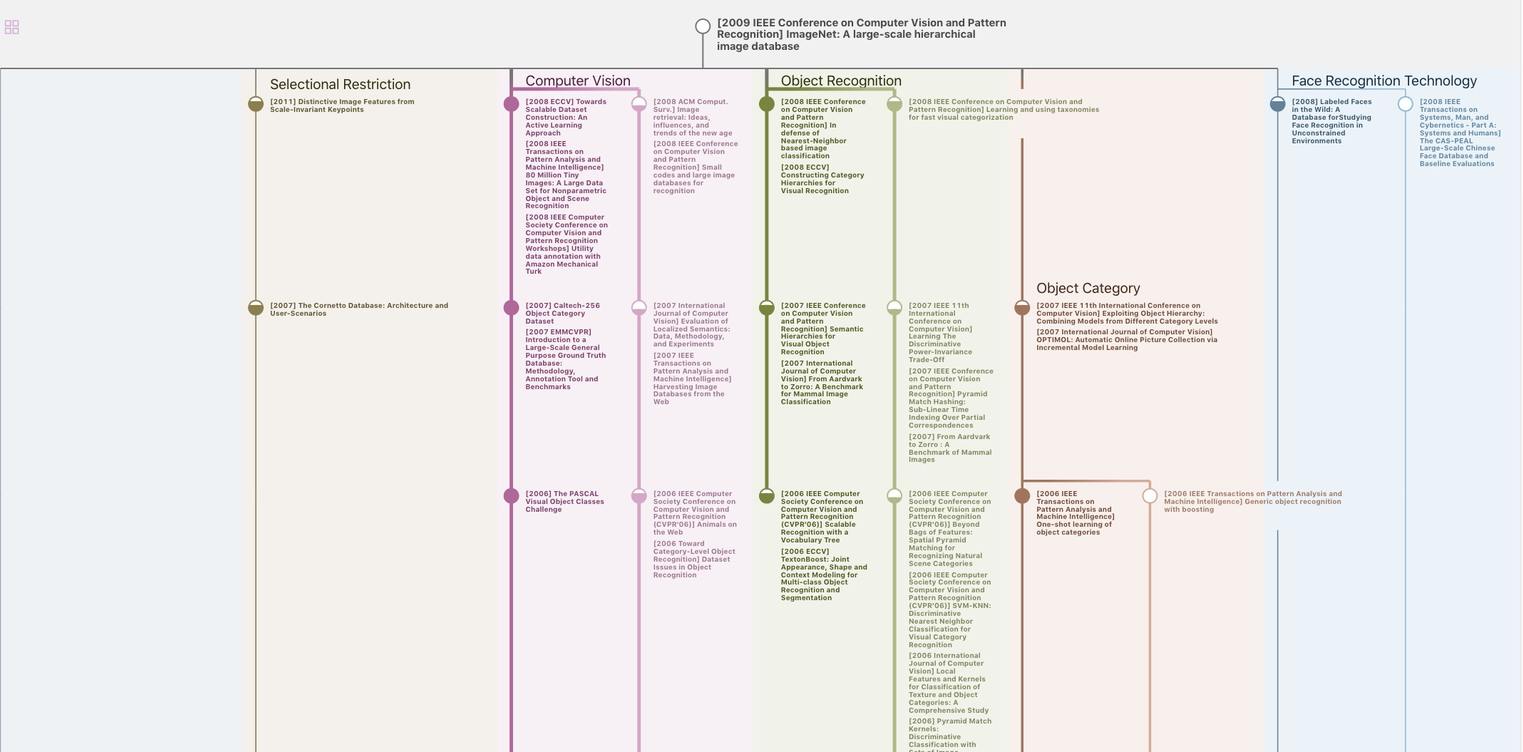
生成溯源树,研究论文发展脉络
Chat Paper
正在生成论文摘要