Low Redundant Attention Network for Efficient Image Super-Resolution
ICASSP 2024 - 2024 IEEE International Conference on Acoustics, Speech and Signal Processing (ICASSP)(2024)
摘要
Transformer-based models have demonstrated impressive performance in image super-resolution (SR), but they come with a high computational overhead. In this paper, we present a low redundant attention network (LRAN) for efficient image SR. We observe that there is significant similarity in attention maps across heads and blocks, leading to computational redundancy. First, to mitigate the redundancy in attention maps among heads, we introduce a multi-element mechanism in the self-attention computation. This mechanism allows for the incorporation of various types of self-attention, thus increasing inter-head diversity. Second, to address this redundancy in attention maps among blocks, we propose the hamburger architecture, which introduces enhanced local perception units to capture local information. Moreover, this architecture incorporates a single self-attention layer between several efficient MLP layers. Extensive experiments demonstrate that LRAN outperforms the latest models in lightweight SR, achieving a better trade-off between SR quality and latency. For instance, LRAN surpasses SwinIR-light by 0.25dB PSNR in ×4 SR on Urban100, while running ×5 faster.
更多查看译文
关键词
Super-resolution,Transformer,Self-attention
AI 理解论文
溯源树
样例
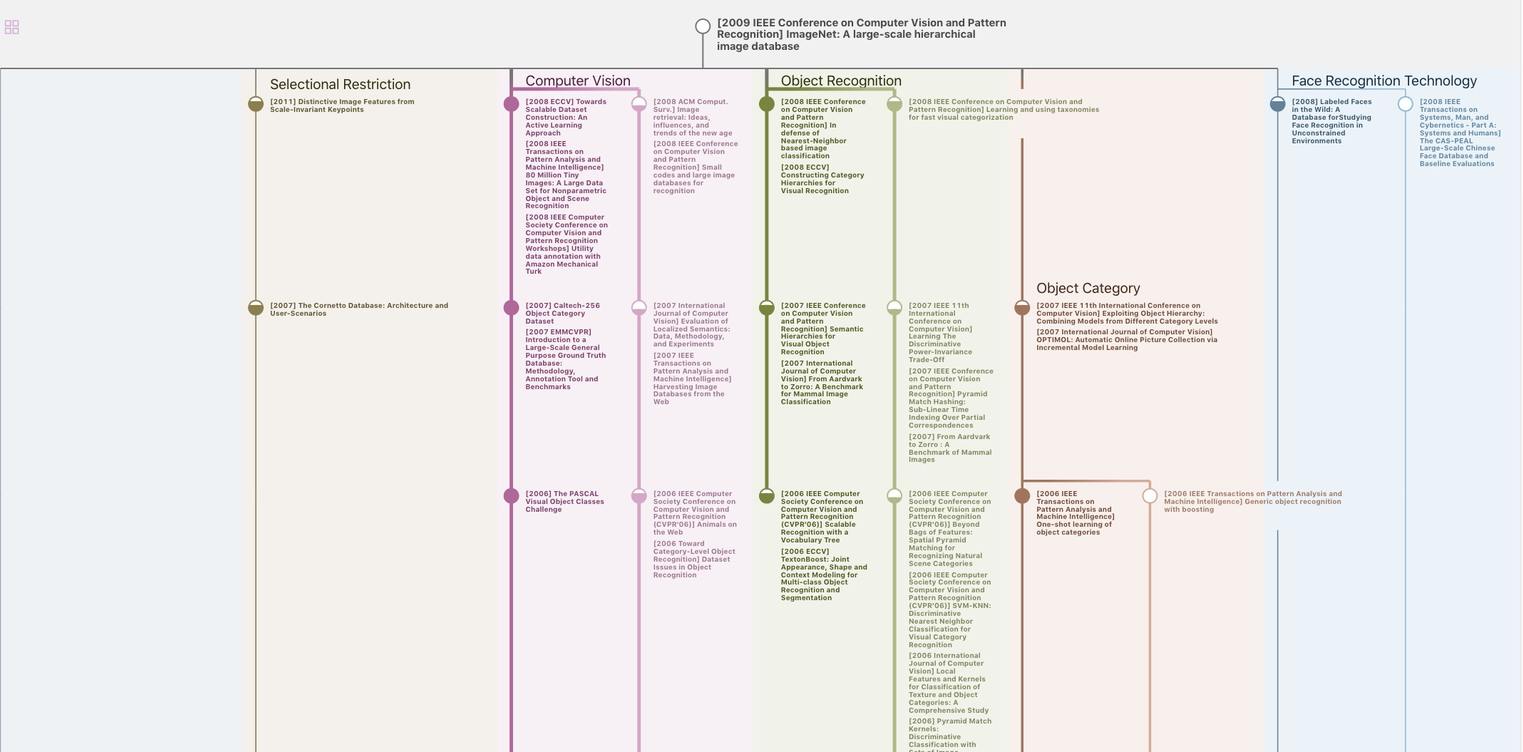
生成溯源树,研究论文发展脉络
Chat Paper
正在生成论文摘要