Towards a community-wide effort for benchmarking in subsurface hydrological inversion: benchmarking cases, high-fidelity reference solutions, procedure and a first comparison
crossref(2024)
摘要
Abstract. Inversion in subsurface hydrology refers to estimating spatial distributions of (typically hydraulic) properties, often associated with quantified uncertainty. Many methods are available, each characterized by a set of assumptions, approximations, and numerical implementations. Only a few intercomparison studies have been performed (in the remote past) amongst different approaches (e.g., Zimmerman et al., 1998; Hendricks Franssen et al., 2009). These intercomparisons guarantee broad participation to push forward research efforts of the entire subsurface hydrological inversion community. However, in past studies until now, comparisons were made among approximate methods without firm reference solutions. Without reference solutions, one can only compare competing best estimates and their associated uncertainties in an intercomparison sense, and absolute statements on accuracy are unreachable. Our current initiative defines benchmarking scenarios for groundwater model inversion. These are targeted for community-wide use as test cases in intercomparison scenarios. Here, we develop five synthetic, open-source benchmarking scenarios for the inversion of hydraulic conductivity from pressure data. We also provide highly accurate reference solutions produced with massive high-performance computing and with a high-fidelity MCMC-type solution algorithm. Our high-end reference solutions are publicly available, as well as the benchmarking scenarios, the reference algorithm, and suggested benchmarking metrics. Thus, in comparison studies, one can test against high-fidelity reference solutions rather than discussing different approximations. To demonstrate how to use these benchmarking scenarios, reference solutions, and suggested metrics, we provide a blueprint comparison of a specific ensemble Kalman filter version. We invite the community to use our benchmarking scenarios and reference solutions now and into the far future in a community-wide effort towards clean and conclusive benchmarking. For now, we aim at an article collection in an appropriate journal, where such clean comparison studies can be submitted together with an editorial summary that provides an overview.
更多查看译文
AI 理解论文
溯源树
样例
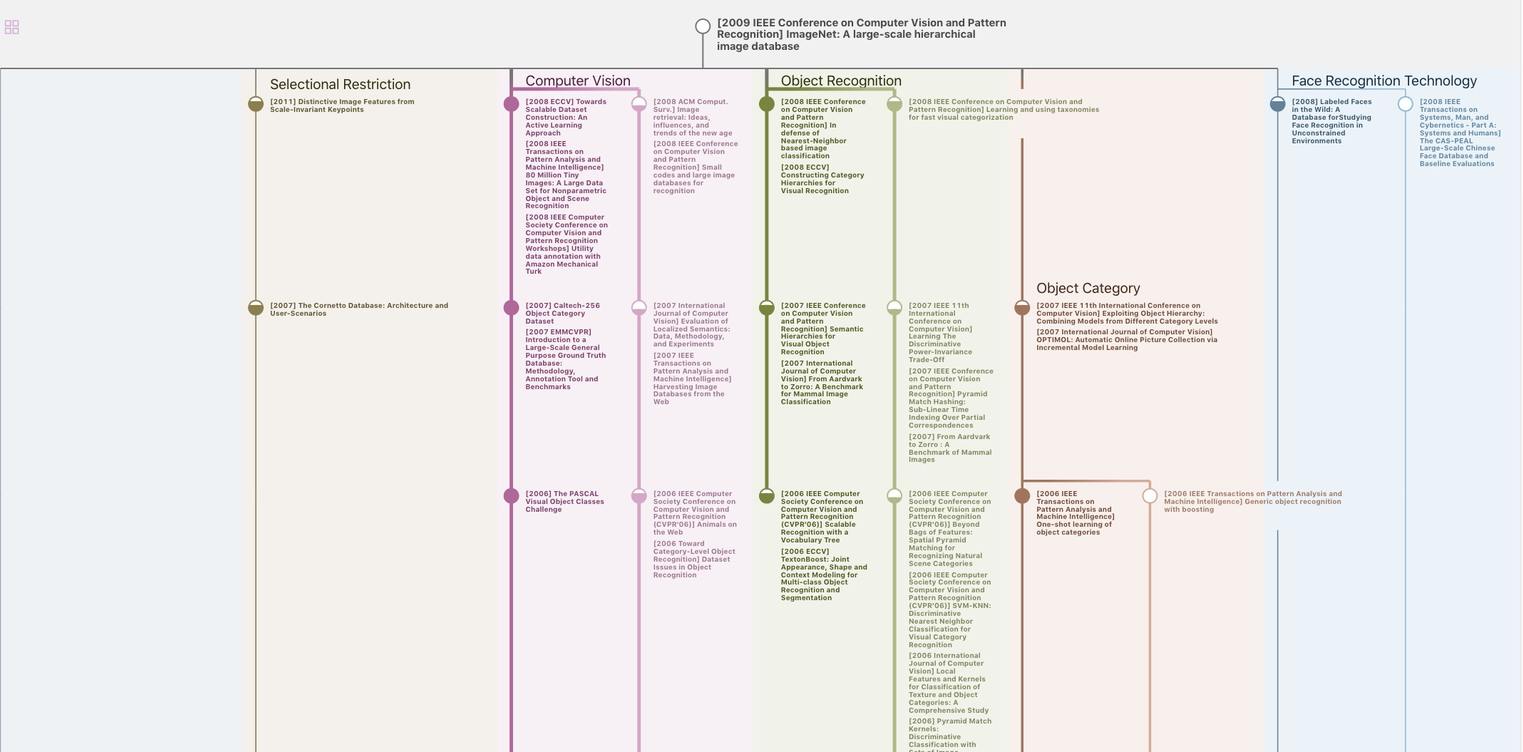
生成溯源树,研究论文发展脉络
Chat Paper
正在生成论文摘要