Diffusion Denoising as a Certified Defense against Clean-label Poisoning
arxiv(2024)
摘要
We present a certified defense to clean-label poisoning attacks. These
attacks work by injecting a small number of poisoning samples (e.g., 1
contain p-norm bounded adversarial perturbations into the training data to
induce a targeted misclassification of a test-time input. Inspired by the
adversarial robustness achieved by denoised smoothing, we show how an
off-the-shelf diffusion model can sanitize the tampered training data. We
extensively test our defense against seven clean-label poisoning attacks and
reduce their attack success to 0-16
time accuracy. We compare our defense with existing countermeasures against
clean-label poisoning, showing that the defense reduces the attack success the
most and offers the best model utility. Our results highlight the need for
future work on developing stronger clean-label attacks and using our certified
yet practical defense as a strong baseline to evaluate these attacks.
更多查看译文
AI 理解论文
溯源树
样例
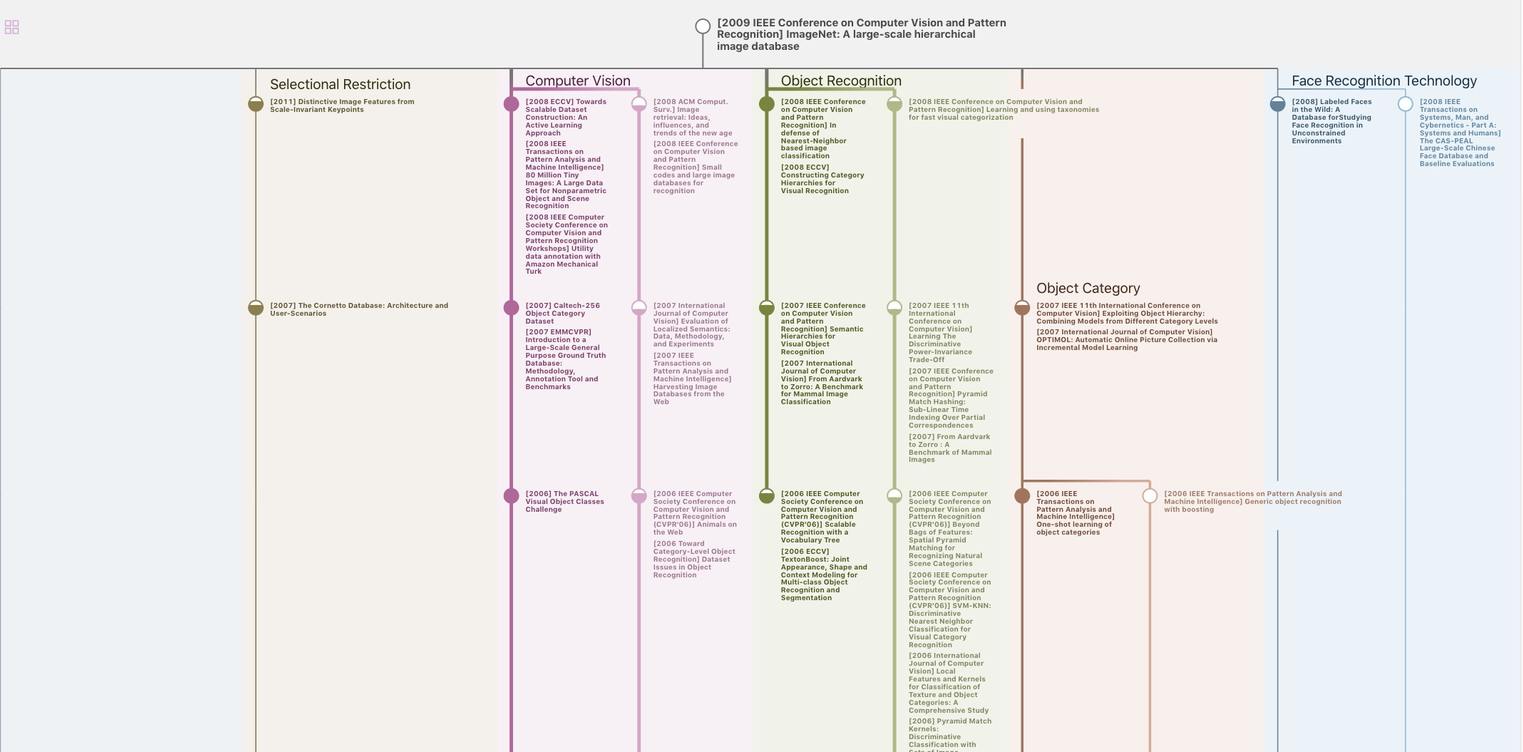
生成溯源树,研究论文发展脉络
Chat Paper
正在生成论文摘要