Radar Recognition in the Wild: Enhancing Radar Emitter Recognition through Auto-Correlation Model-Agnostic Meta Learning
ICASSP 2024 - 2024 IEEE International Conference on Acoustics, Speech and Signal Processing (ICASSP)(2024)
摘要
In Electronic Support Measure (ESM) systems, the recognition of radar emitters stands as a pivotal yet intricate task. The complex electromagnetic environments, however, often hinders the collection of clean radar signal data, and results in data with different noise levels. Consequently, formulating a robust recognition model with limited data becomes a big challenge, further compounded by the demand for generalizability across scenarios with different noise levels. While Model-Agnostic Meta Learning (MAML) has proven its effectiveness in solving few-shot learning problems in computer vision, its application in radar signal processing has remained unexplored deeply. This paper pioneers the incorporation of MAML and autocorrelation into radar signal processing. To fit MAML to radar signals, we introduce a novel loss function, termed AC-Loss, designed to facilitate learning effective signal representation by retaining the periodicity of the radar pulses which is the key feature for recognizing different Pulse Repetition Intervals (PRIs). This proposed Autocorrelation Model-Agnostic Meta Learning (AC-MAML) enhances its recognition capabilities while using only a sparse number of signal samples in both source and target domains. Empirical results show the superiority of AC-MAML, achieving an impressive average recognition accuracy of 90.4% across seven diverse target domain scenarios.
更多查看译文
关键词
Radar Emitter Recognition,Model-Agnostic Meta-Learning,Auto-Correlation,Domain Adaptation,Few-shot Learning
AI 理解论文
溯源树
样例
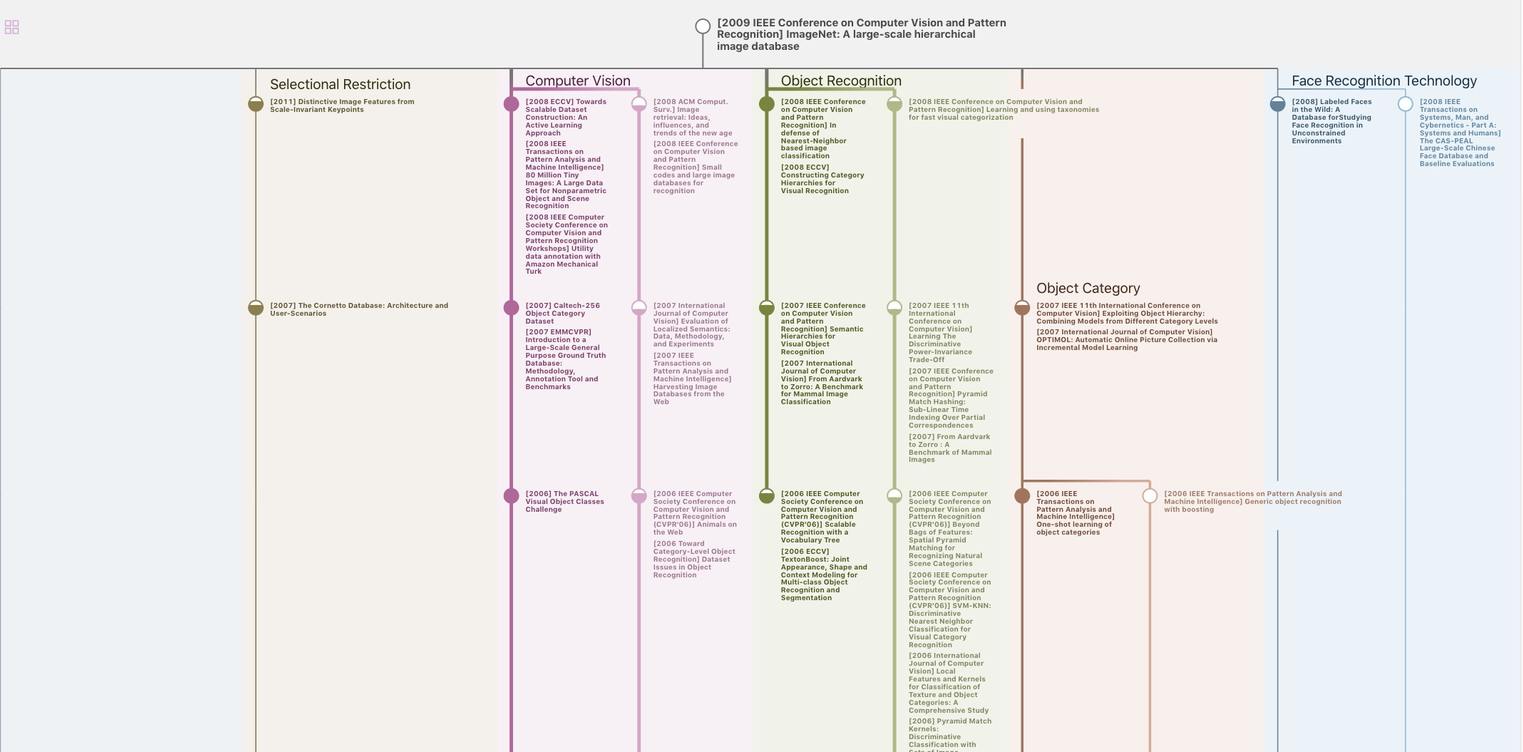
生成溯源树,研究论文发展脉络
Chat Paper
正在生成论文摘要