Learnable Statistical Moments Pooling for Automatic Modulation Classification
ICASSP 2024 - 2024 IEEE International Conference on Acoustics, Speech and Signal Processing (ICASSP)(2024)
摘要
We introduce a differentiable statistical moment aggregation layer, enabling networks to learn the optimal method of statistical moment pooling for automatic modulation classification. Statistical pooling, a cornerstone of convolutional networks, consolidates activations into fixed-length representations. Traditionally, this entails mean, variance, and higher-ordered statistics pooling defined as fixed hyperparameters. By enabling the statistics layer to become differentiable, networks are able to optimize the method of statistical aggregations, transcending predefined hyperparameters. With our approach, the statistical moment order is differentiable. Our results demonstrate learned statistical moments are able to outperform fixed-moments—improving modulation classification performance of a time-domain signal.
1
更多查看译文
关键词
Statistical moments,automatic modulation classification,deep learning,learnable pooling
AI 理解论文
溯源树
样例
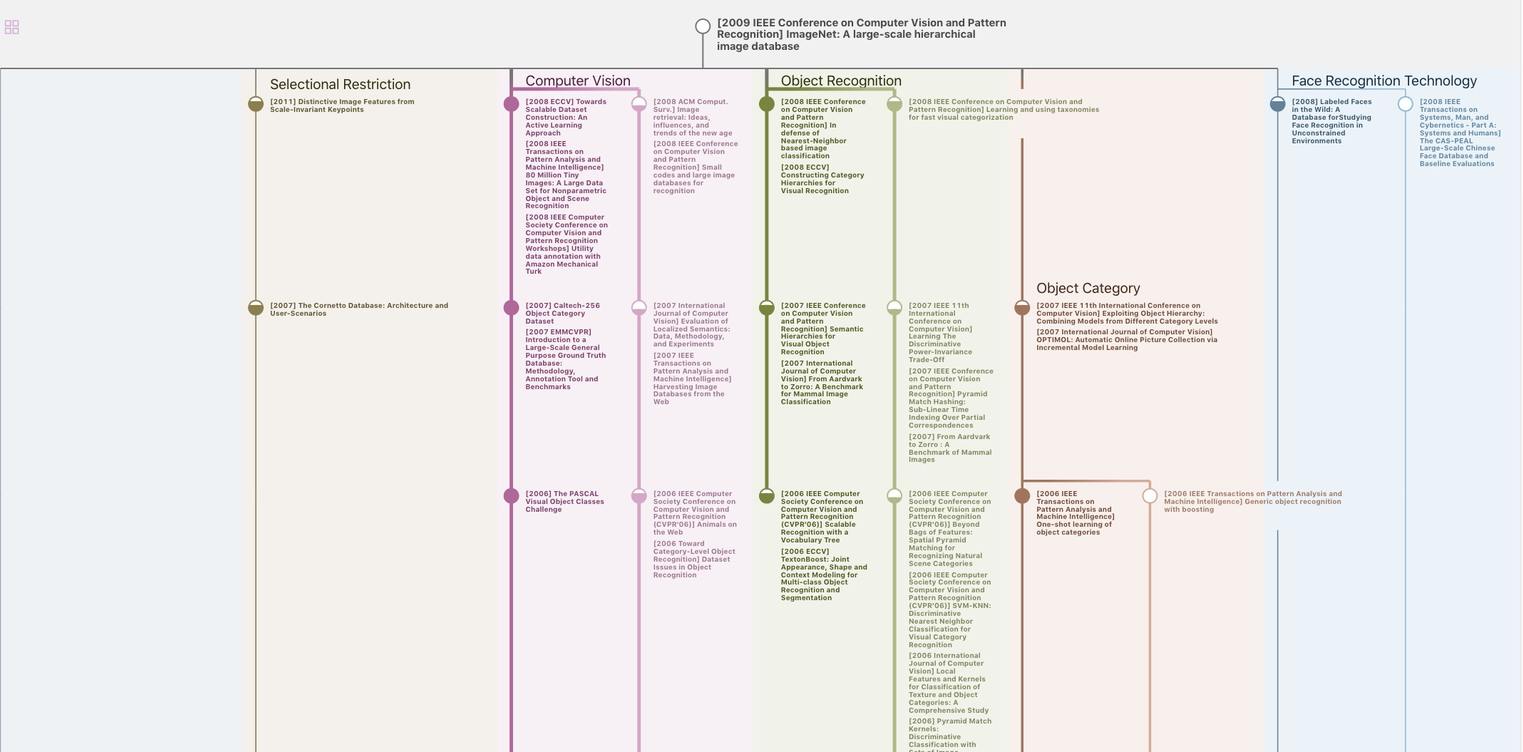
生成溯源树,研究论文发展脉络
Chat Paper
正在生成论文摘要