Enabling Reliable Water-Air Direct Optical Wireless Communication for Uncrewed Vehicular Networks: A Deep Reinforcement Learning Approach
IEEE Transactions on Vehicular Technology(2024)
摘要
The next generation wireless networks demand full coverage from the sky to the deep ocean, in which the water-air cross-boundary communication is a critical component in a coordinated uncrewed vehicular network that connects both uncrewed aerial vehicles (UAVs) and autonomous underwater vehicles (AUVs). In such a network, optical wireless communication (OWC) is an emerging and promising technology to implement direct high-speed wireless communication through the water-to-air interface. However, due to the high directionality of optical beams and the dynamics of the harsh oceanic environments, it faces significant challenges to achieve reliable and robust seamless cross-boundary communication, as the waves cause beam deflection and the mobility of the transceivers makes the link worse or even results in link disconnections. To deal with these challenges, in this paper, we investigate both static and mobile application scenarios to enable reliable water-air direct optical communications via the deep reinforcement learning (DRL) approaches. In the static scenario, we propose an active alignment method and derive the optimal beamwidth to satisfy the requirement of bit error rate (BER) and improve the link availability. To cope with the oceanic environment-induced channel dynamics, in the mobile scenario, we design a DRL-based UAV control strategy by incorporating the extended Kalman filter (EKF) prediction technique to enhance reliable communication between the mobile transceivers. The numerical simulations demonstrate that the proposed schemes achieve impressive performance in terms of energy consumption and reliable communications under both the static and mobile scenarios.
更多查看译文
关键词
Optical Wireless Communication,Uncrewed Aerial Vehicles,Markov Decision Process,Extended Kalman Filter,Deep Reinforcement Learning
AI 理解论文
溯源树
样例
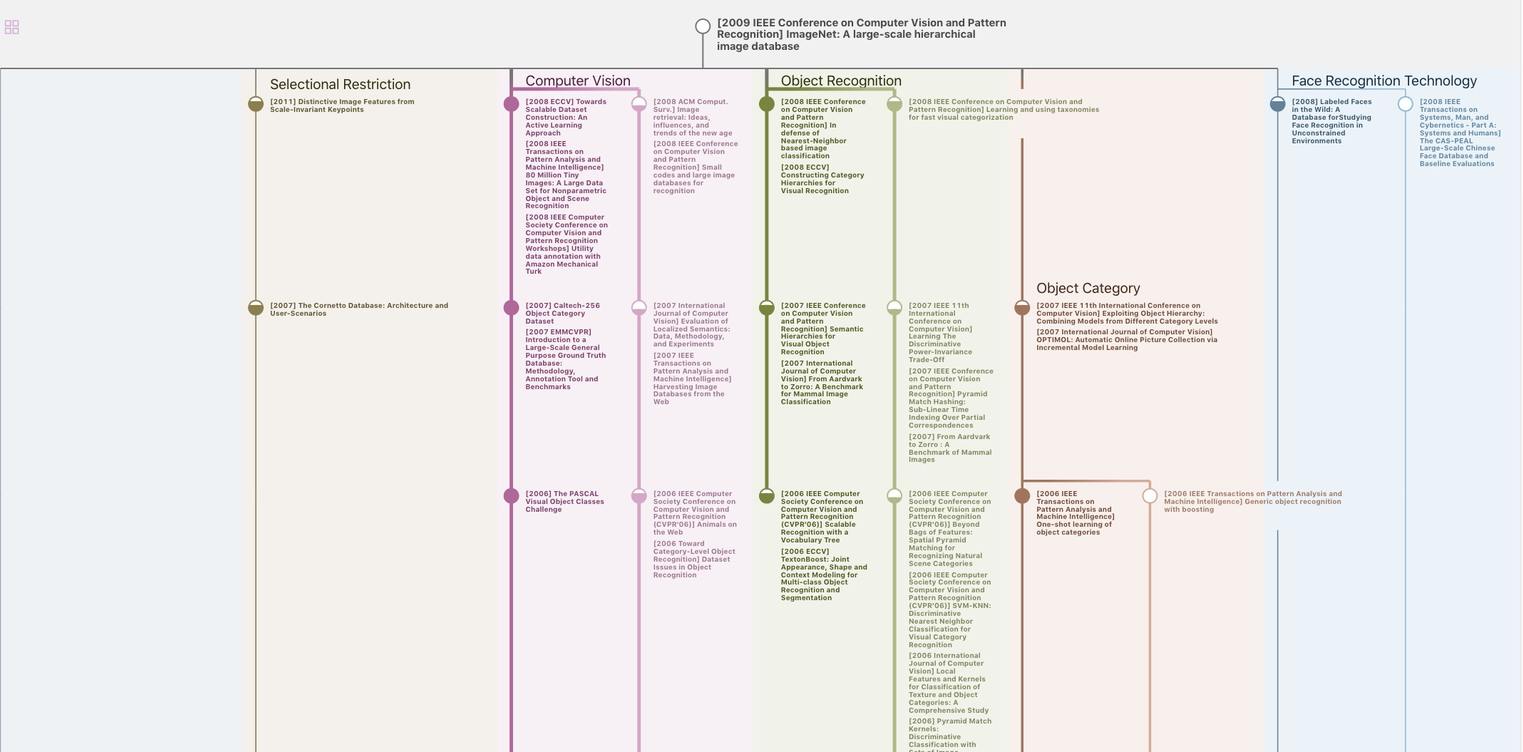
生成溯源树,研究论文发展脉络
Chat Paper
正在生成论文摘要