Machine Learning LSST 3x2pt analyses – forecasting the impact of systematics on cosmological constraints using neural networks
arxiv(2024)
摘要
Validating modeling choices through simulated analyses and quantifying the
impact of different systematic effects will form a major computational
bottleneck in the preparation for 3×2 analysis with Stage-IV surveys
such as Vera Rubin Observatory's Legacy Survey of Space and Time (LSST). We can
significantly reduce the computational requirements by using machine learning
based emulators, which allow us to run fast inference while maintaining the
full realism of the data analysis pipeline. In this paper, we use such an
emulator to run simulated 3×2 (cosmic shear, galaxy-galaxy lensing, and
galaxy clustering) analyses for mock LSST-Y1/Y3/Y6/Y10 surveys and study the
impact of various systematic effects (galaxy bias, intrinsic alignment,
baryonic physics, shear calibration and photo-z uncertainties). Closely
following the DESC Science Requirement Document (with several updates) our main
findings are: a) The largest contribution to the `systematic error
budget' of LSST 3×2 analysis comes from galaxy bias uncertainties, while
the contribution of baryonic and shear calibration uncertainties are
significantly less important. b) Tighter constraints on intrinsic
alignment and photo-z parameters can improve cosmological constraints
noticeably, which illustrates synergies of LSST and spectroscopic surveys. c) The scale cuts adopted in the DESC SRD may be too conservative and pushing
to smaller scales can increase cosmological information significantly. d)
We investigate the impact of photo-z outliers on 3×2 pt analysis and
find that we need to determine the outlier fraction to within 5-10% accuracy
to ensure robust cosmological analysis. We caution that these findings depend
on analysis choices (parameterizations, priors, scale cuts) and can change for
different settings.
更多查看译文
AI 理解论文
溯源树
样例
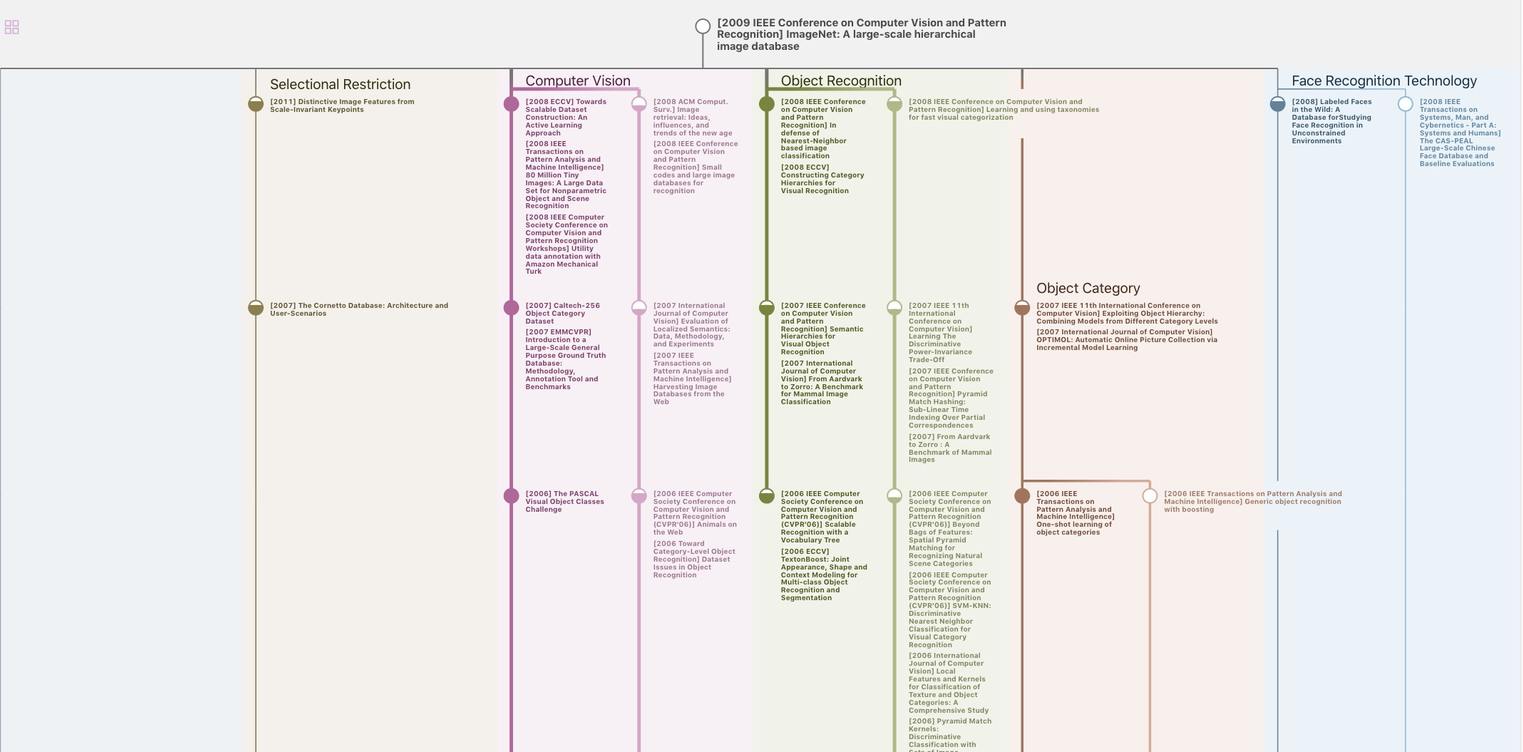
生成溯源树,研究论文发展脉络
Chat Paper
正在生成论文摘要