One-Epoch Training with Single Test Sample in Test Time for Better Generalization of Cough-Based Covid-19 Detection Model
ICASSP 2024 - 2024 IEEE International Conference on Acoustics, Speech and Signal Processing (ICASSP)(2024)
摘要
The outbreak of COVID-19 has raised researchers’ attention to audio-based rapid disease detection. Most of the previous studies have obtained competitive detection performance. However, these results are usually obtained by testing data from the same source offline. When making cross-dataset testing, the performance may deteriorate dramatically due to inconsistent data distribution between different datasets. In addition, in practical application, the model has to make a prediction for the current test audio without any prior information, which requires good model generalization under limited training data. To address the above issues, we adopt a test-time training framework to achieve a cough-based COVID-19 detection model with better generalizability. In the model development stage, resnet18 serves as the backbone network and a self-supervised learning branch is added as an auxiliary task. In testing stage, the model parameters are first fine-tuned by the self-supervised branch with the single test audio as input, and then the classification head outputs predictions. The proposed method is validated on three open-source datasets using a variety of hyperparameters. In cross-dataset testing, AUC and UAR increase by 3.65% and 3% on average absolutely, respectively. The results show that the proposed framework is applicable to improve the model performance in practical application.
更多查看译文
关键词
Cough,COVID-19 detection,Test-time training,Self-supervised learning
AI 理解论文
溯源树
样例
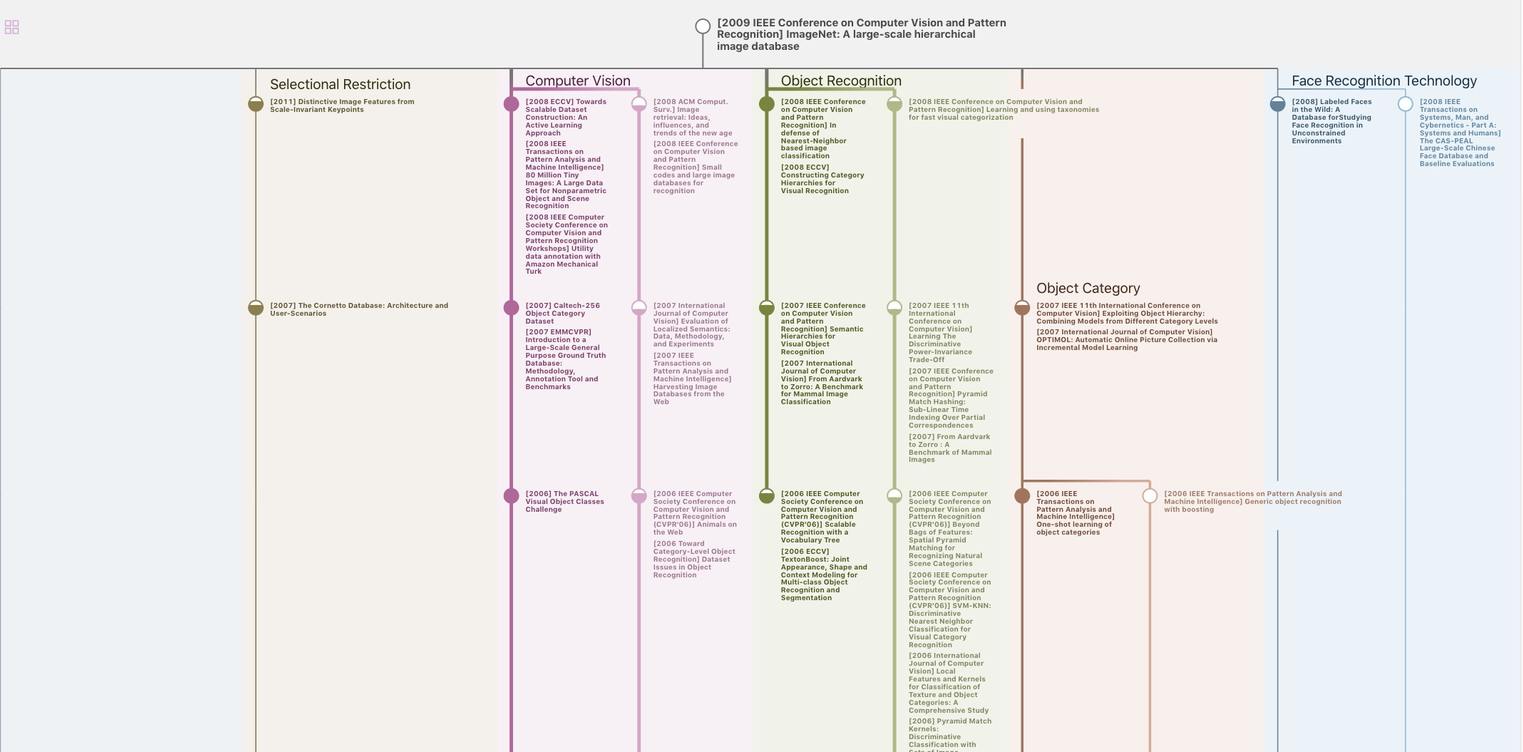
生成溯源树,研究论文发展脉络
Chat Paper
正在生成论文摘要