BiFA: Remote Sensing Image Change Detection With Bitemporal Feature Alignment.
IEEE Trans. Geosci. Remote. Sens.(2024)
摘要
Despite the success of deep learning-based change detection methods, their existing insufficiency in temporal (channel, spatial) and multi-scale alignment have rendered them insufficient capability in mitigating external factors (illumination changes and perspective differences, etc.) arising from different imaging conditions during change detection. In this paper, a Bi-temporal Feature Alignment (BiFA) model is proposed to produce a precise change detection map in a lightweight manner by reducing the impact of irrelevant factors. Specifically, for the temporal alignment, the Bi-temporal Interaction (BI) module is proposed to realize the alignment of the bi-temporal image channel level. Our intuition is introducing the bi-temporal interaction in the feature extraction stage may benefit suppressing the interference, such as illumination changes. Simultaneously, the Alignment module based on Differential Flow Field (ADFF) is proposed to explicitly estimate the offset of the bi-temporal image and realize their spatial level alignment to mitigate the inadequate registration resulting from different perspectives. Furthermore, for the multi-scale alignment, we introduce the Implicit Neural alignment Decoder (IND) to produce more refined prediction maps achieving precise alignment of multi-scale features by learning continuous image representations in coordinate space. Our BiFA outperforms other state-of-the-art methods on six datasets (such as the F1/IoU scores are improved by 2.70%/3.91%, 2.01%/2.94% on LEVIR+-CD and SYSU-CD, respectively) and displays greater robustness in cross-resolutions change detection. Our code is available at https://github.com/zmoka-zht/BiFA.
更多查看译文
关键词
Change detection (CD),high-resolution optical remote sensing image,feature alignment,bi-temporal interaction,flow field,implicit neural representation
AI 理解论文
溯源树
样例
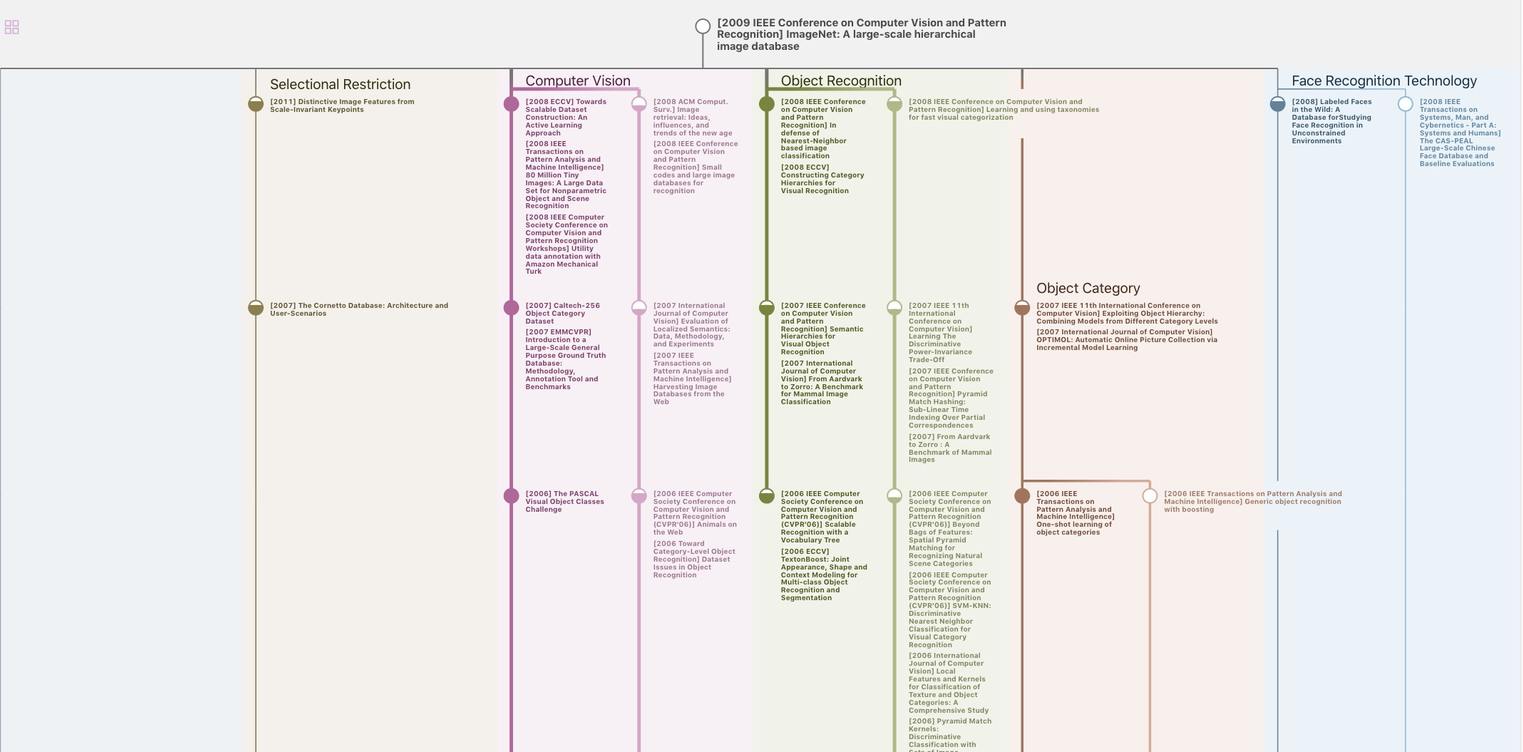
生成溯源树,研究论文发展脉络
Chat Paper
正在生成论文摘要