Digital Shadow Sensor Framework for Smart Agriculture: Time Series Prediction Through Data Segmentation and Clustering.
Consumer Communications and Networking Conference(2024)
摘要
In IoT-based smart agriculture systems, the acquisition of high-quality sensing data plays a key role in enabling informed decisions by farmers. However, the deployment of wireless sensors in remote agricultural areas often entails facing frequent network disconnections. Furthermore, the necessity for uninterrupted monitoring is in contrast with the energy-saving requirements of battery-operated IoT devices. In this paper, we propose a solution that decouples the connection between IoT sensors and the cloud by introducing an intermediary software stratum acting as an edge data proxy. More specifically, our framework assigns a Digital Shadow to each IoT device, endowed with the capability to predict forthcoming sensor values during devices' low-power modes or network disconnections. Three main contributions are provided in this paper. First, we present the architecture and operations of our framework, enabling the orchestration of phases of sensor readings and data forecasting and the consequential adjustment of the device sampling frequency. Second, we introduce a novel time series forecasting approach that aims at identifying diverse patterns in the sensor time series and at instantiating distinct Machine Learning (ML) models for each individual pattern. Third, we validate our framework through real-world soil moisture datasets. The experimental results showcase the efficacy of our approach in delivering accurate forecasts, outperforming single-model and context-aware methodologies.
更多查看译文
关键词
Smart agriculture,Internet of Things (IoT),Digital Shadow,Software Architecture,Machine Learning
AI 理解论文
溯源树
样例
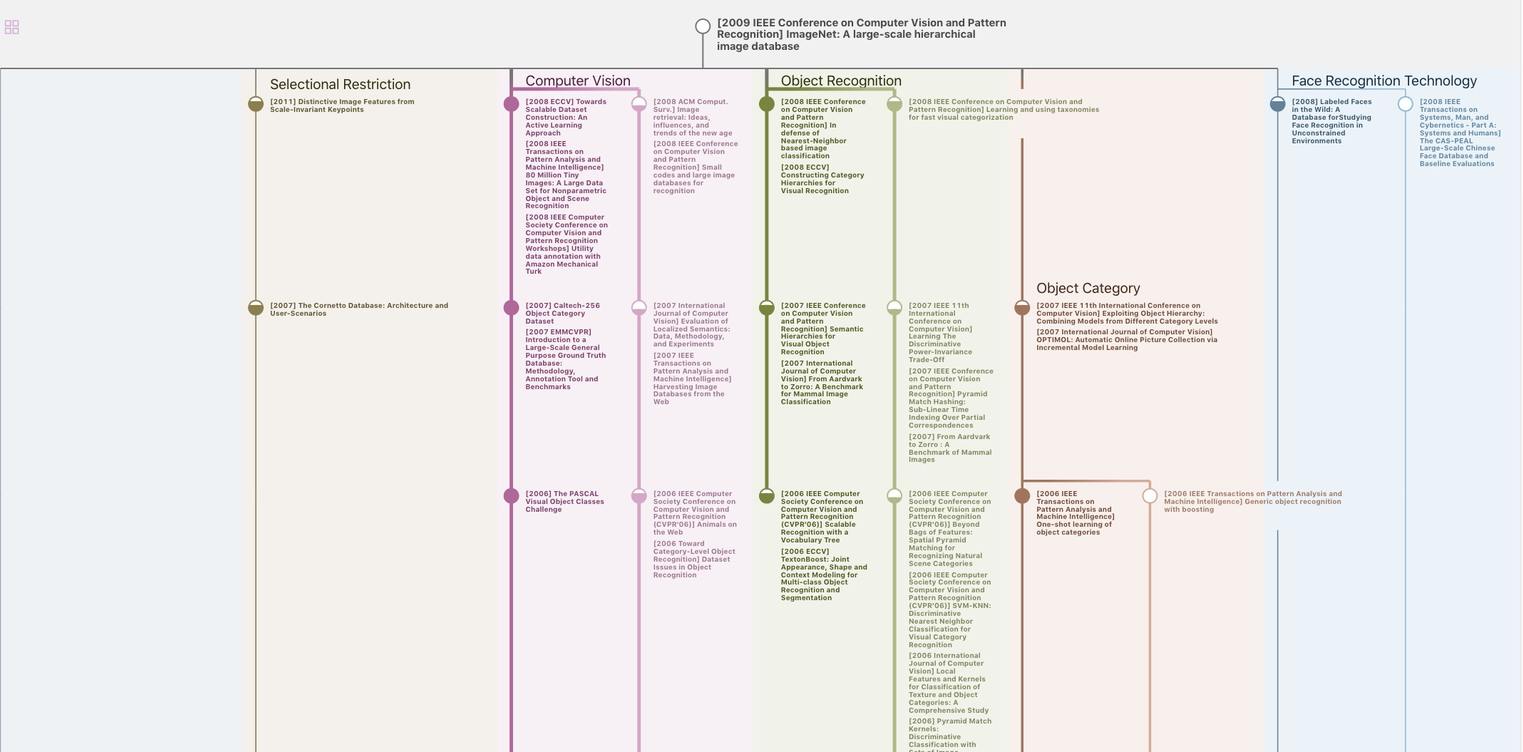
生成溯源树,研究论文发展脉络
Chat Paper
正在生成论文摘要