A Novel Medical Image Fusion Framework Integrating Multi-scale Encoder-Decoder with Discrete Wavelet Decomposition
ICASSP 2024 - 2024 IEEE International Conference on Acoustics, Speech and Signal Processing (ICASSP)(2024)
摘要
In recent years, many fusion algorithms based on multi-scale transform or neural networks have been proposed to improve medical image fusion (MIF) performance. However, there is still enormous potential to explore the combination of different fusion theories. In this paper, we propose a novel MIF framework to integrate powerful feature representation abilities of the deep learning model and accurate frequency decomposition characteristics of discrete wavelet transform (DWT). Firstly, a multi-scale encoder-decoder network is well-trained to extract feature information in different scales and achieve efficient image reconstruction. In particular, DWT is introduced into each scale to decompose the extracted features into high- and low-frequency sub-bands for information preservation during down-sampling. An elaborate feature fusion process is designed to achieve multi-scale fusion while merging different frequency sub-bands. Experiment results on benchmark datasets demonstrate that the proposed fusion framework outperforms current state-of-the-art methods with comparable time complexity in both objective and subjective evaluation.
更多查看译文
关键词
medical image fusion,discrete wavelet transform,multi-scale encoder-decoder,high- and low-frequency sub-bands,feature fusion
AI 理解论文
溯源树
样例
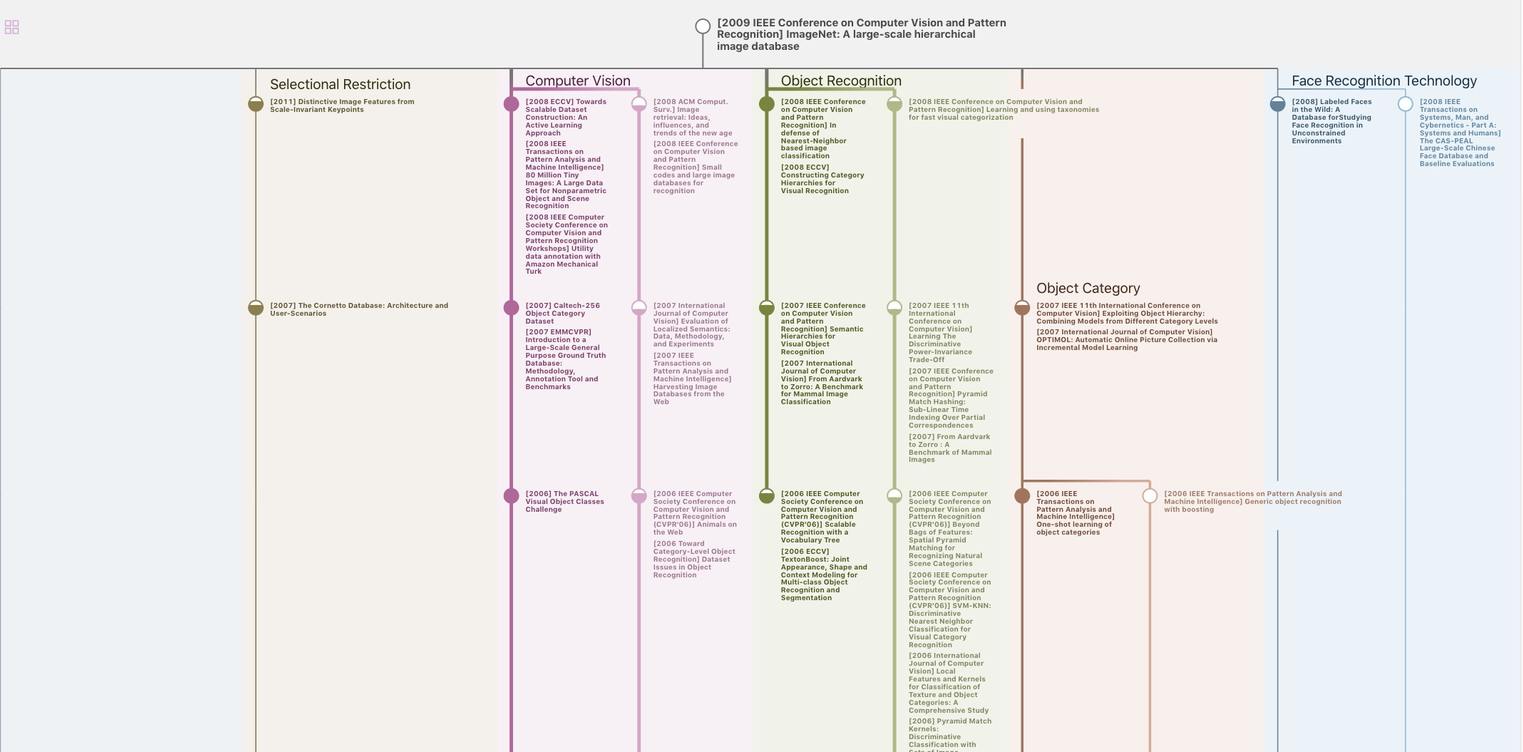
生成溯源树,研究论文发展脉络
Chat Paper
正在生成论文摘要