Phase-Space-Guided Deep Learning For Time Series Forecasting
ICASSP 2024 - 2024 IEEE International Conference on Acoustics, Speech and Signal Processing (ICASSP)(2024)
摘要
Time series forecasting is crucial, yet the challenge of escalating errors in chaotic data and natural phenomena prediction endures. Existing methods for recursive strategies face difficulties in Multi-Input Multi-Output scenarios. A unified learning framework addressing error growth alongside these models is lacking, despite advanced neural networks. While dynamical system theory has inspired research in time series forecasting, these approaches struggle to estimate and mitigate error growth adequately. To address these gaps, we introduce Phase-Space-Guided Forecasting (PSGF), rooted in dynamical system theory. PSGF transforms data into high-dimensional phase space, quantifies error growth rates, and incorporates them into the neural network via Error Growth Awareness Loss (EGAL). PSGF enhances the utilization of dynamical constraints, reducing the need for additional feature engineering or hyperparameter tuning. Experimental results on chaotic systems and real-world climate data demonstrate PSGF’s significant accuracy improvements on diverse deep learning models.
更多查看译文
关键词
Time series forecasting,error growth,phase space,Lyapunov exponent,loss function
AI 理解论文
溯源树
样例
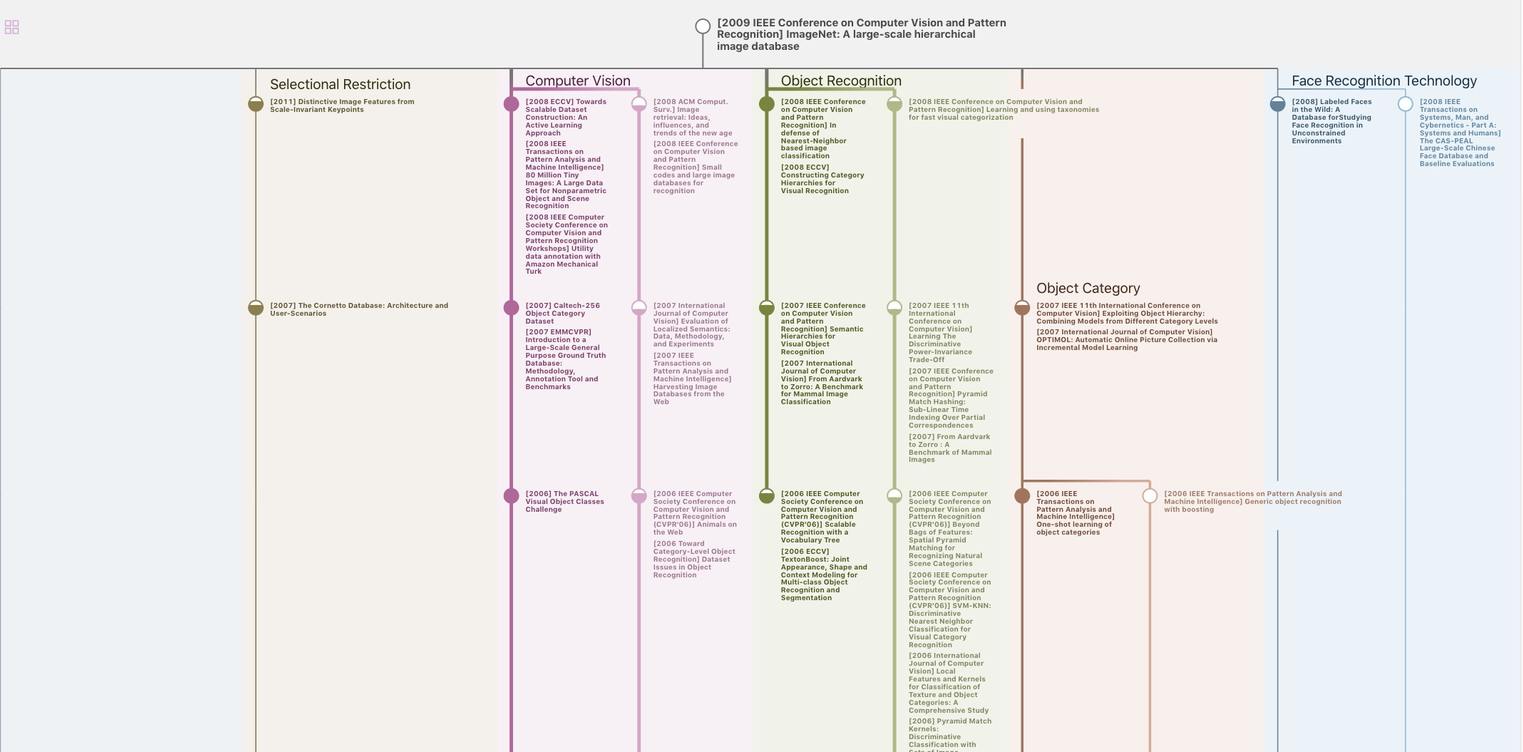
生成溯源树,研究论文发展脉络
Chat Paper
正在生成论文摘要