Learning Density Regulated and Multi-View Consistent Unsigned Distance Fields
ICASSP 2024 - 2024 IEEE International Conference on Acoustics, Speech and Signal Processing (ICASSP)(2024)
摘要
Learning unsigned distance fields (UDF) directly from raw point clouds as the implicit representation for surface reconstruction is a promising learning-based method for reconstructing open surfaces and supervision-free attributes. In most UDF methods, Chamfer Distance (CD), the commonly used metric in 3D domains, is reckoned as the preferable loss function for training neural networks that predict UDFs. However, CD intrinsically suffers from deficiencies like the insensitivity to point density distribution and the inclination to be diverged by outliers, which may severely hamper the reconstruction performance. In this regard, we propose DM-UDF, a method that learns density-regulated and multi-view consistent UDFs by revising CD loss with the dynamic three-phase loss function. Specifically, we adopt a carefully designed CD derivative called Density-aware Chamfer Distance (DCD) for detecting different density distributions to alleviate the distribution imbalance problem in the reconstructed surfaces. Further, to generate surfaces with fine-grained local details, a differentiable rendering view loss is also introduced into the hybrid design of our loss function, measuring the fidelity of projected images under different camera poses to maintain multi-view consistency. We conducted surface reconstruction tasks on both synthetic and real scan datasets and experimental results show that DM-UDF achieves state-of-the-art performance. Code is available at dm-udf.
更多查看译文
关键词
Unsigned distance fields,3D reconstruction,density distribution,multi-view consistency
AI 理解论文
溯源树
样例
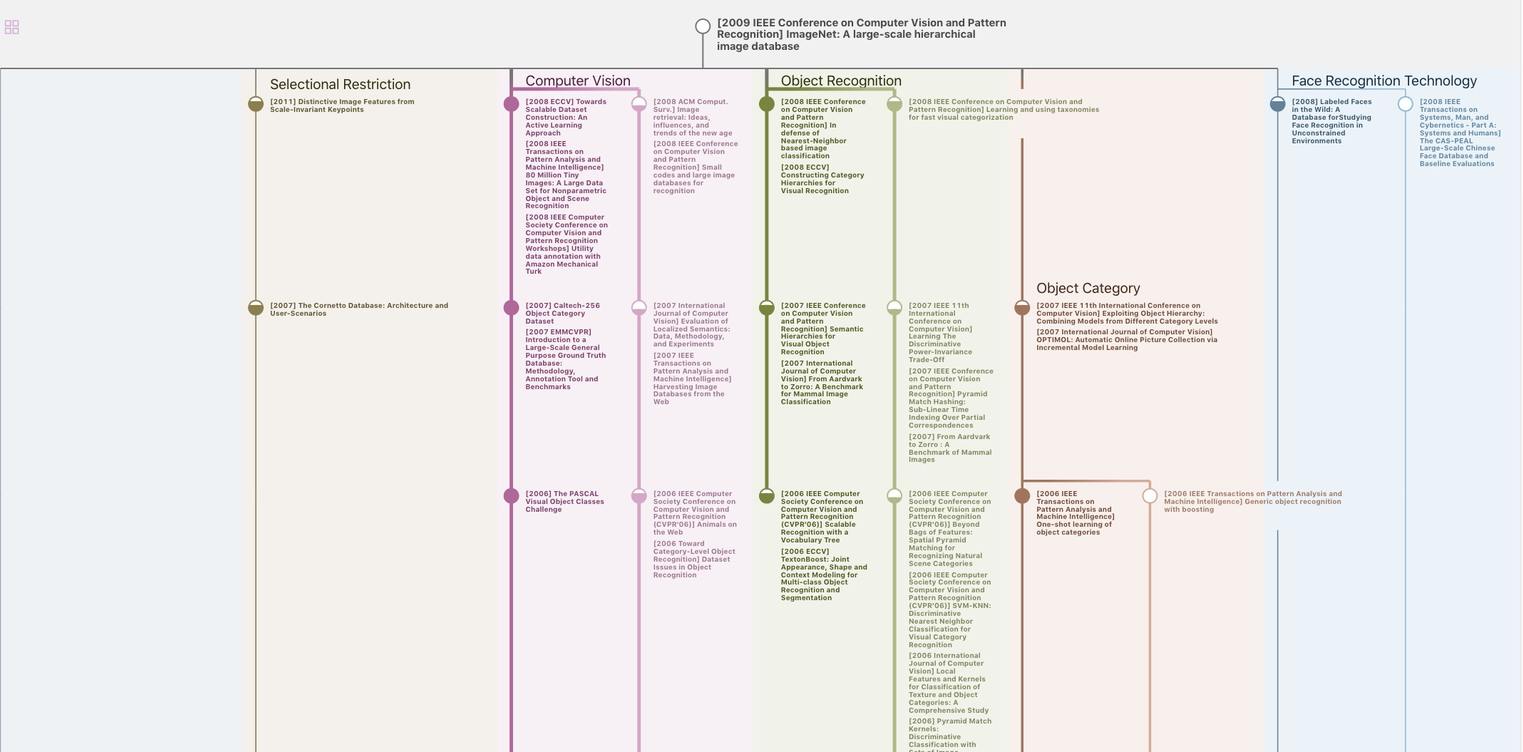
生成溯源树,研究论文发展脉络
Chat Paper
正在生成论文摘要