Image Coding for Analytics via Adversarially Augmented Adaptation
ICASSP 2024 - 2024 IEEE International Conference on Acoustics, Speech and Signal Processing (ICASSP)(2024)
摘要
Image Coding for Machine (ICM) aims to compress an image so that the reconstructed one can meet the requirements of both human vision and machine vision. Existing methods apply the constraint from the downstream models to improve machine analytics performance while compromising the visual quality. This paper proposes a novel adversarially augmented adaptation route that achieves a better trade-off between the utility of the human and machine perspectives by making slight changes to the image manifold. In detail, a targeted adversarial attack is employed to generate subtle image perturbations that are nearly imperceptible to humans but significantly improve machine analytic performance. These perturbed images would be subsequently employed as ground truth to guide training/fine-tuning of an end-to-end image compression network. Note that, our method is a plug-and-play framework that does not rely on any change in existing architecture or loss functions. Extensive experimental results demonstrate the superiority of the proposed scheme over conventional ICM frameworks and the effectiveness of our design.
更多查看译文
关键词
Image coding for machine,Machine vision,Targeted adversarial attack,Machine vision coding
AI 理解论文
溯源树
样例
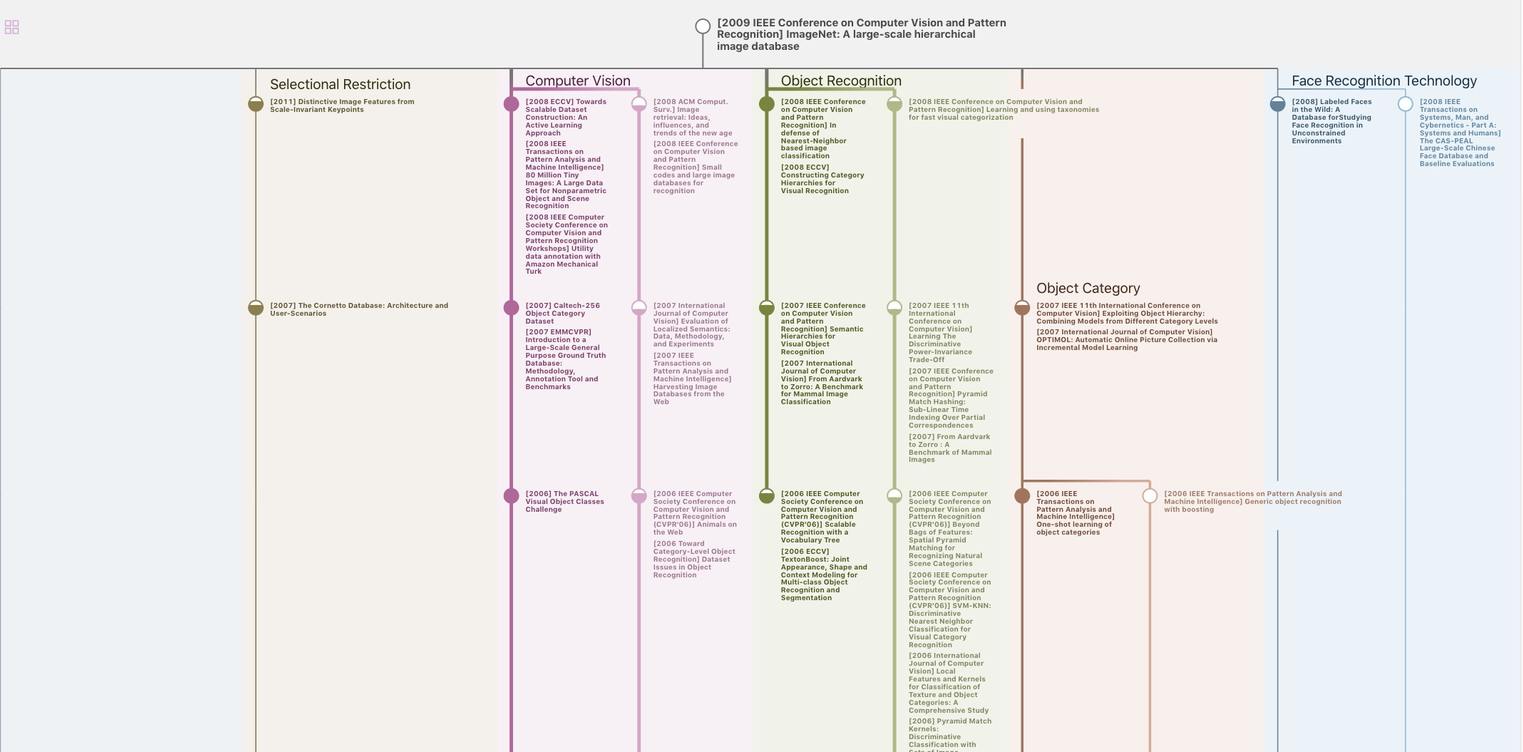
生成溯源树,研究论文发展脉络
Chat Paper
正在生成论文摘要