Transformer-Inspired Lightweight Model for Efficient Time Series Forecasting
ICASSP 2024 - 2024 IEEE International Conference on Acoustics, Speech and Signal Processing (ICASSP)(2024)
摘要
Accuracy and efficiency are pivotal considerations in the field of time series forecasting. Through the integration of meticulously designed temporal components, the Transformer-based models have significantly enhanced the accuracy of time series prediction. However, due to the utilization of attention mechanism, these models suffer heightened complexity and limited practicality. To address the complexity challenge and develop a more practical and lightweight model, we propose the integration of the core designs of Transformer-based models into the framework of CNNs. This integration entails the assimilation of low-level features from shallower layers into the prediction process, effectively addressing the challenge of inadequate resolution within deep feature maps. Furthermore, we leverage depthwise convolution to capture finer temporal details and adopt a channel-sharing prediction strategy to reduce the parameter count of the model. Empirical results, derived from experiments conducted on seven distinct datasets, substantiate the superior performance of our stream-lined model. Notably, it outperforms competing models in terms of both accuracy and model complexity.
更多查看译文
关键词
Multi-scale features,Depthwise convolution,Lightweight model,Time series forecasting
AI 理解论文
溯源树
样例
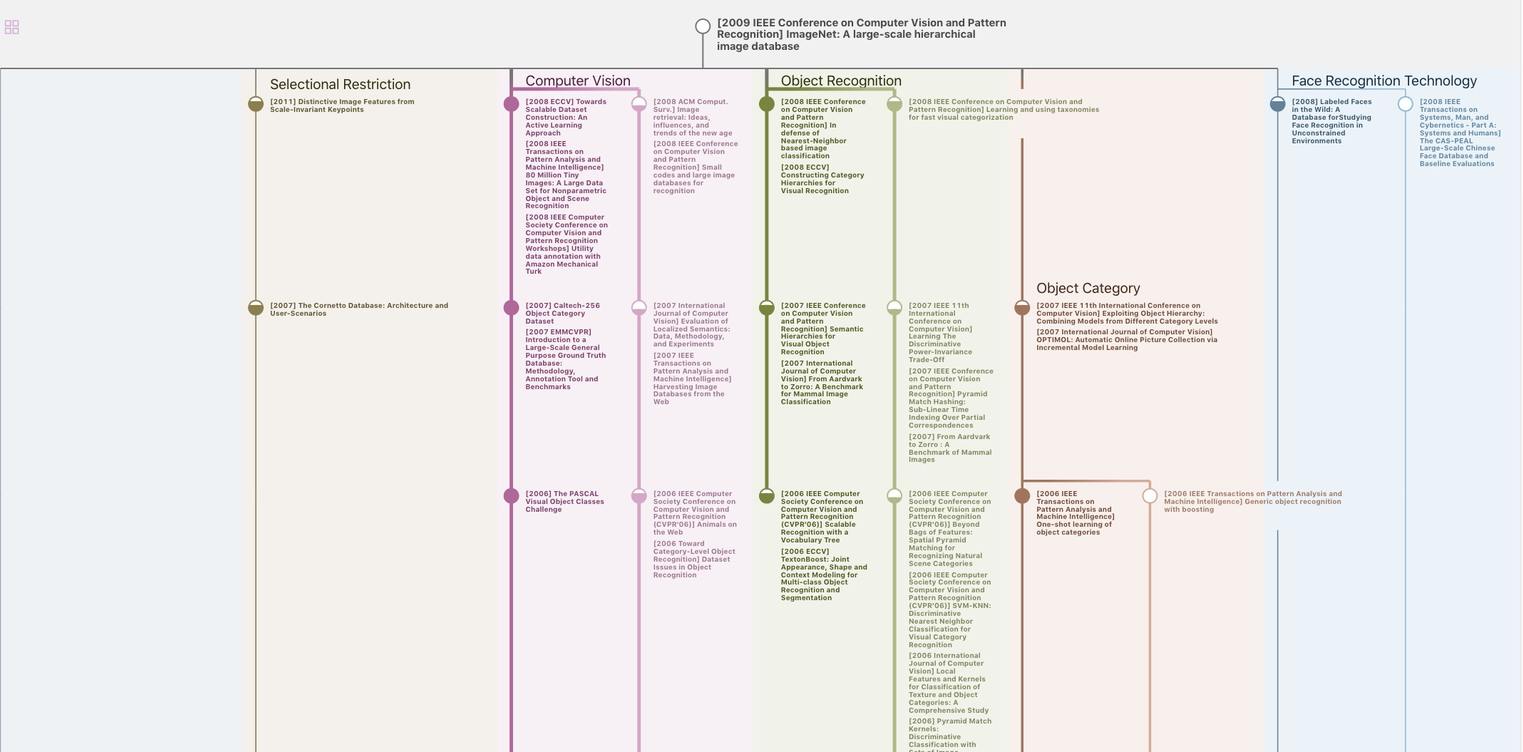
生成溯源树,研究论文发展脉络
Chat Paper
正在生成论文摘要