Training Ultra-Low-Latency Spiking Neural Networks from Scratch
ICASSP 2024 - 2024 IEEE International Conference on Acoustics, Speech and Signal Processing (ICASSP)(2024)
摘要
Spiking Neural networks (SNN) have emerged as an attractive spatio-temporal computing paradigm for a wide range of low-power vision tasks. However, state-of-the-art (SOTA) SNN models either incur multiple time steps which hinder their deployment in real-time use cases or increase the training complexity significantly. To mitigate this concern, we present a training framework (from scratch) for SNNs with ultra-low (down to 1) time steps that leverages the Hoyer regularizer. We calculate the threshold for each BANN layer as the Hoyer extremum of a clipped version of its activation map. The clipping value is determined through training using gradient descent with our Hoyer regularizer. We evaluate the efficacy of our training framework on large-scale vision tasks, including traditional and event-based image recognition and object detection. Our experiments demonstrate up to 34× increase in compute efficiency with a marginal accuracy/mAP drop compared to non-spiking networks. Finally, we implement our framework in the Lava-DL library, thereby enabling the deployment of our SNN models in the Loihi neuromorphic chip.
更多查看译文
关键词
spiking neural networks,Hoyer regularizer,extremum,threshold,Lava-DL
AI 理解论文
溯源树
样例
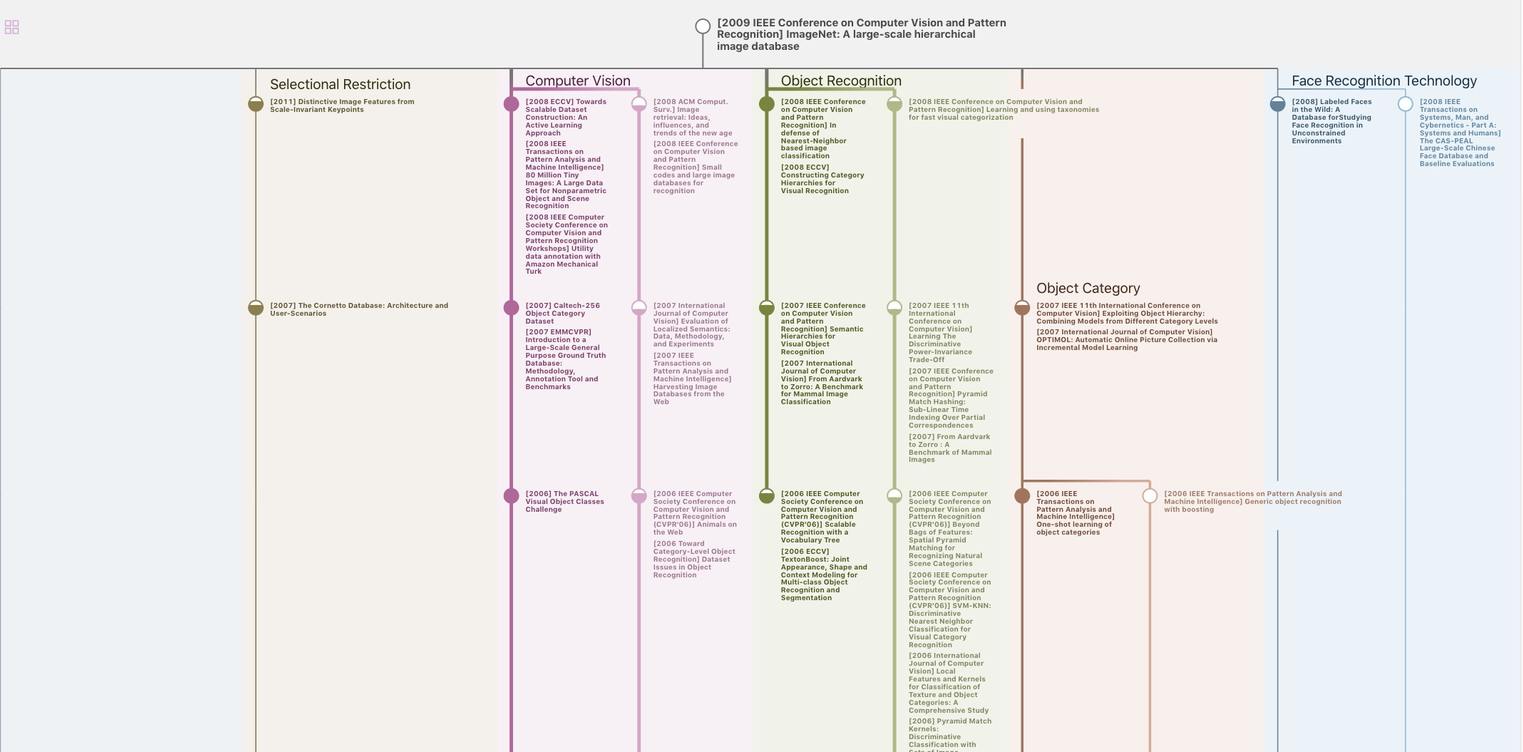
生成溯源树,研究论文发展脉络
Chat Paper
正在生成论文摘要