Progressive Image Synthesis from Semantics to Details with Denoising Diffusion GAN
ICASSP 2024 - 2024 IEEE International Conference on Acoustics, Speech and Signal Processing (ICASSP)(2024)
摘要
Although denoising diffusion probabilistic models (DDPMs) have shown remarkable progress in image generation, they typically face two main challenges: the time-expensive sampling process and the semantically meaningless latent space, which are often addressed separately in previous works. In particular, the latest representative work Denoising Diffusion GAN reduces the sampling steps to as few as two but ignores the semantics of the latent space. To address the two challenges simultaneously, we propose a two-stage framework to make the latent space of Denoising Diffusion GAN more semantically meaningful while enjoying its efficiency. Extensive results on three benchmark datasets demonstrate that our proposed diffusion model achieves competitive results with only two sampling steps in unconditional image generation. More importantly, the latent space of our diffusion model trained for unconditional image generation is shown to be semantically meaningful, which can be exploited on various downstream tasks (e.g., attribute editing) without further training.
更多查看译文
关键词
Diffusion model,GAN,Latent space,Semantics
AI 理解论文
溯源树
样例
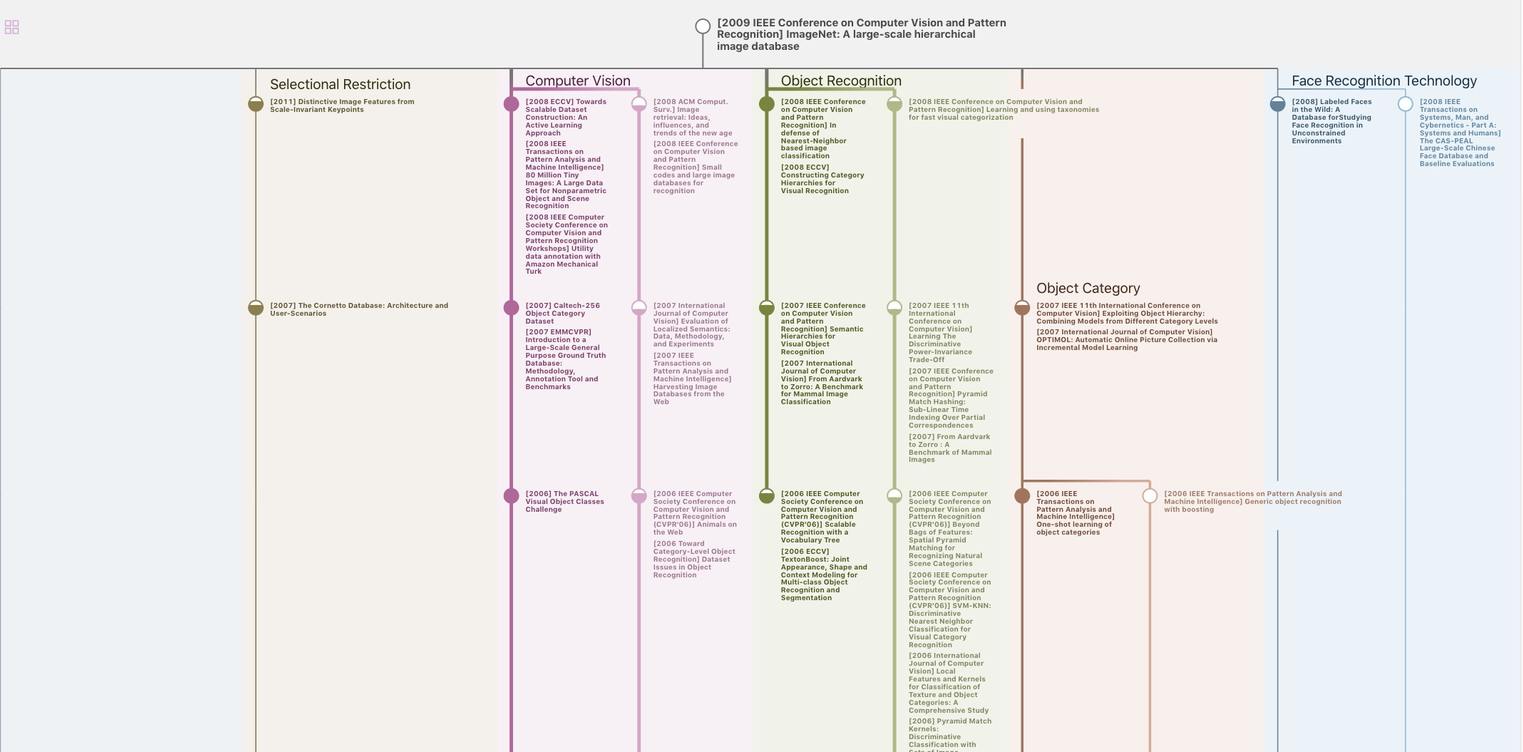
生成溯源树,研究论文发展脉络
Chat Paper
正在生成论文摘要