Efficient Point Cloud Attribute Compression Framework using Attribute-Guided Graph Fourier Transform
ICASSP 2024 - 2024 IEEE International Conference on Acoustics, Speech and Signal Processing (ICASSP)(2024)
摘要
The Graph Fourier Transform (GFT) has achieved remarkable success in point cloud attribute compression due to its adaptability in handling irregular signals. However, the conventional graph-based attribute compression method mostly relies on geometry information to construct the Laplace matrix. In the case of poor geometry-attribute correlation, this method cannot represent the attribute correlation well enough to bring efficient attribute compression performance. In this paper, we propose an efficient point cloud attribute compression scheme using attribute-guided graph Fourier transform. We utilize the attribute features to guide the block partitioning process and design a novel scanning method to efficiently represent attribute patterns, which enhances the efficiency of GFT without imposing extra storage overhead introduced by recording clustering results. Furthermore, a coding module of DC coefficients is proposed for leveraging inter-block correlation, and a tailored coding strategy of AC coefficients is designed based on the distribution of transform coefficients. Experimental results demonstrate that our method achieves better compression performance compared with the latest G-PCC version 22.
更多查看译文
关键词
Point Cloud,Attribute Compression,Graph Fourier Transform
AI 理解论文
溯源树
样例
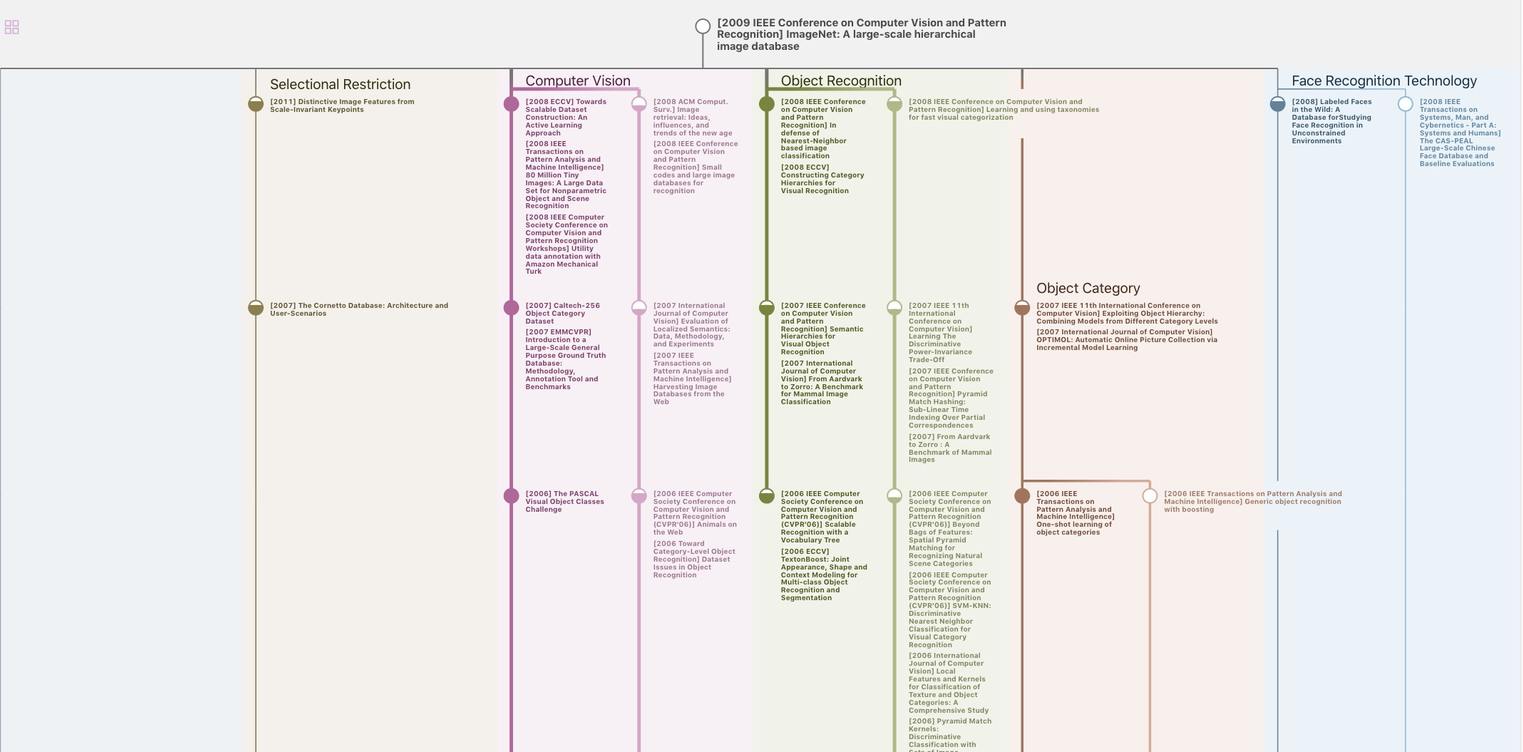
生成溯源树,研究论文发展脉络
Chat Paper
正在生成论文摘要