Joint Multi-Feature Information Entity Alignment for Cross-Lingual Temporal Knowledge Graph with BERT
IEEE Transactions on Big Data(2024)
摘要
Entity alignment is the most critical technology of knowledge fusion, which aims to identify and align entities that exist in different knowledge graphs (KGs) but represent the same real-world objects. Temporal knowledge graphs (TKGs) extend static triples into quadruples by introducing temporal information, which is more in line with the dynamic changes of the real world and is often used to enhance the performance of various applications. However, the existing entity alignment methods mainly focus on traditional KGs, ignoring the temporal information contained in TKGs may lead to misalignment between some similar entities. The latest method enhances the performance of entity alignment by learning temporal information embedding, but it does not make full use of the advantages of temporal information. In this paper, we propose a new Entity Alignment method for Cross-lingual TKG with BERT (EACTB). EACTB uses the BERT model of multi-language training to learn the semantic relevance of entity description. For temporal information, we propose a new and more efficient method to calculate the similarity of temporal information. EACTB uses graph convolution network (GCN) to embed structural information. In addition, iterative method is used to deal with the problem of insufficient training datasets. Experimental results on three cross-lingual TKGs datasets show that EACTB is significantly superior to existing methods.
更多查看译文
关键词
Entity alignment,entity description,temporal knowledge graph
AI 理解论文
溯源树
样例
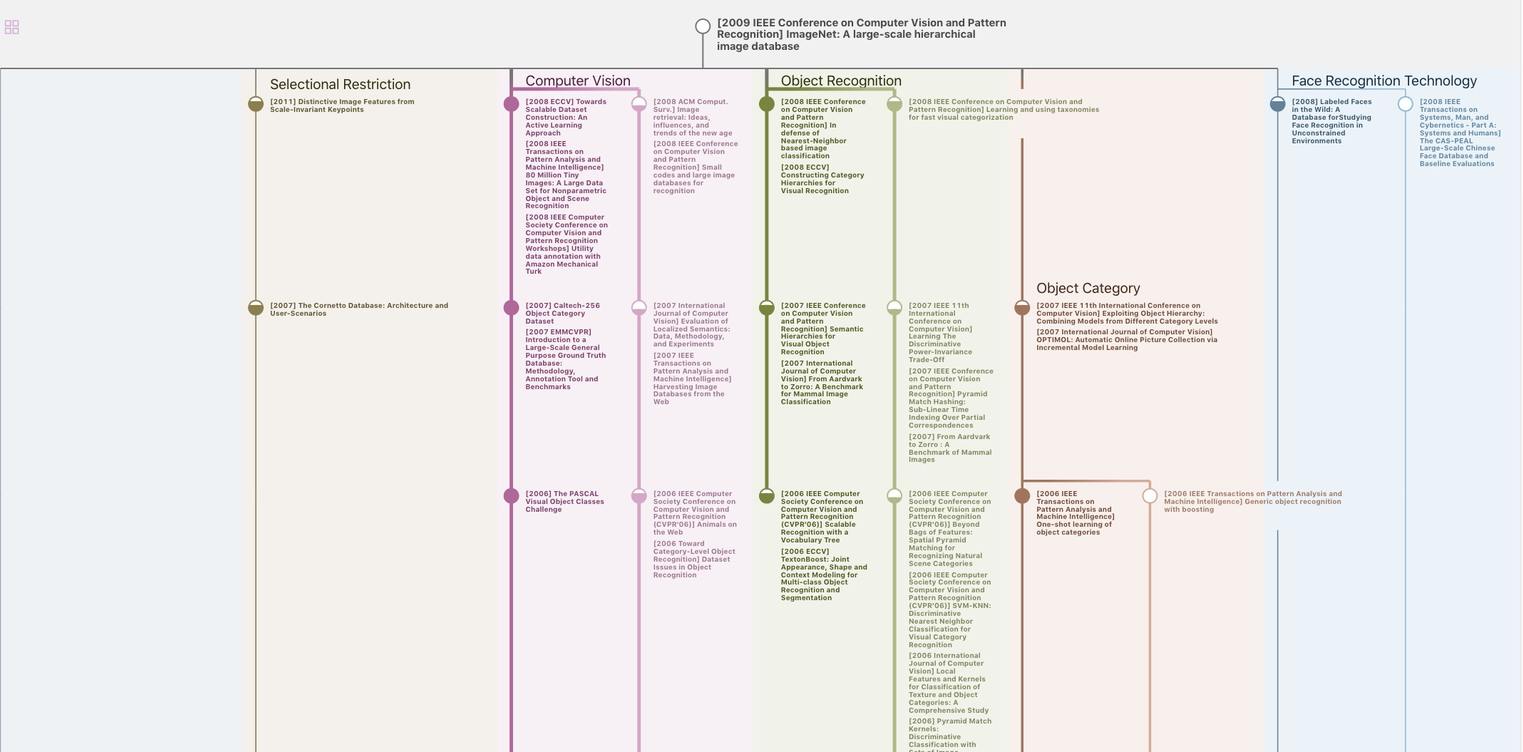
生成溯源树,研究论文发展脉络
Chat Paper
正在生成论文摘要