Data-Driven Energy Profiling for Resource-Efficient 5G Vertical Services.
Consumer Communications and Networking Conference(2024)
摘要
Energy efficiency is a major concern in the development of future mobile networks. Besides the infrastructure, a significant challenge is the power consumption of the user equipment, as it directly affects the quality of experience. We have conducted comprehensive laboratory measurements on the latest commercial 5G devices to assess power consumption, considering different frequencies, bandwidths and duplex patterns. A key result is the non-linear increase in power consumption with uplink transmit power. An empirical model with machine learning methods is proposed to enable quantitative analysis of the power consumption based on the communication behavior. By applying this model to an extensive data set, spatiotemporal predictions of real-world user equipment power consumption were performed. Depending on the deployment location and communication behavior, battery life can be as much as five times lower. These results can be utilized to perform energy-aware scheduling and deployment site selection to enhance the energy footprint of mobile platforms and Internet of Things devices.
更多查看译文
关键词
Machine Learning,Energy Efficiency,Power Consumption,Empirical Model,Internet Of Things,Transmission Power,Quality Of Experience,Mobile Network,Communication Behaviors,Internet Of Things Devices,User Equipment,Increased Power Consumption,Deployment Location,Root Mean Square Error,Energy Consumption,Data Rate,Frequency Band,Data Transfer,Application Programming Interface,Time Division Duplex,Power Consumption Of Devices,Datapath,Power Control,5G Networks,User Datagram Protocol,Mobile Network Operators,Path Loss,Rise In Consumption,Uplink Data
AI 理解论文
溯源树
样例
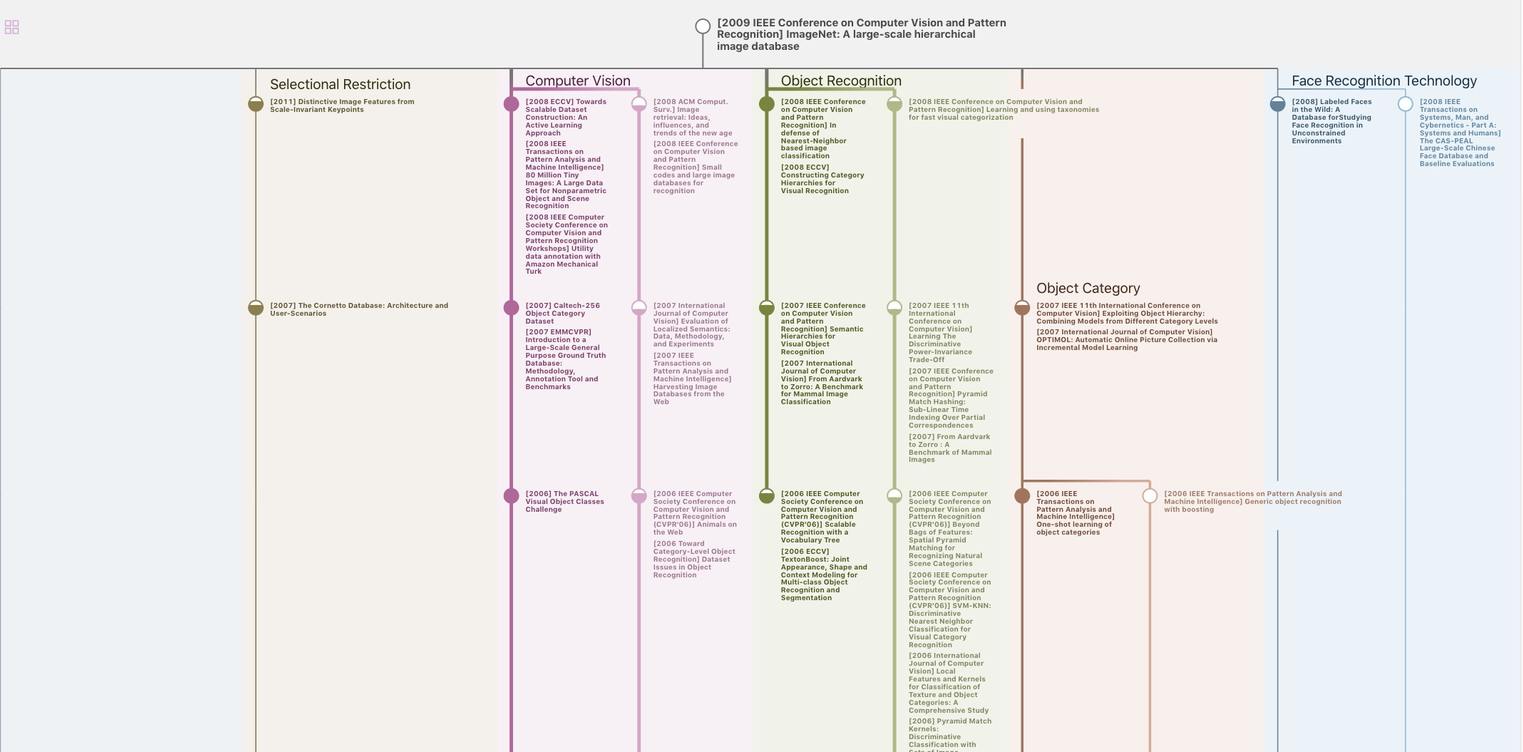
生成溯源树,研究论文发展脉络
Chat Paper
正在生成论文摘要