VTFR-AT: Adversarial Training With Visual Transformation and Feature Robustness
IEEE Transactions on Emerging Topics in Computational Intelligence(2024)
摘要
Research on the robustness of deep neural networks to adversarial samples has grown rapidly since studies have shown that deep learning is susceptible to adversarial perturbation noise. Adversarial training is widely regarded as the most powerful defence strategy against adversarial attacks out of many defence strategies. It has been shown that the adversarial vulnerability of models is due to the learned non-robust feature in the data. However, few methods have attempted to improve adversarial training by enhancing the critical information in the data, i.e., the important region of the object. Moreover, adversarial training is prone to overfitting the model due to the overuse of training set samples. In this paper, we propose a new adversarial training framework with visual transformation and feature robustness, named VTFR-AT. The visual transformation (VT) module enhances principal information in images, weakens background information, and eliminates nuisance noise by pre-processing images. The feature robustness (FR) loss function enhances the network feature extraction partly against perturbation by constraining the feature similarity of the network on similar images. Extensive experiments have shown that the VTFR framework can substantially promote the performance of models on adversarial samples and improve the adversarial robustness and generalization capabilities. As a plug-and-play module, the proposed framework can be easily combined with various existing adversarial training methods.
更多查看译文
关键词
Image classification,adversarial defence,adversarial training,network robustness
AI 理解论文
溯源树
样例
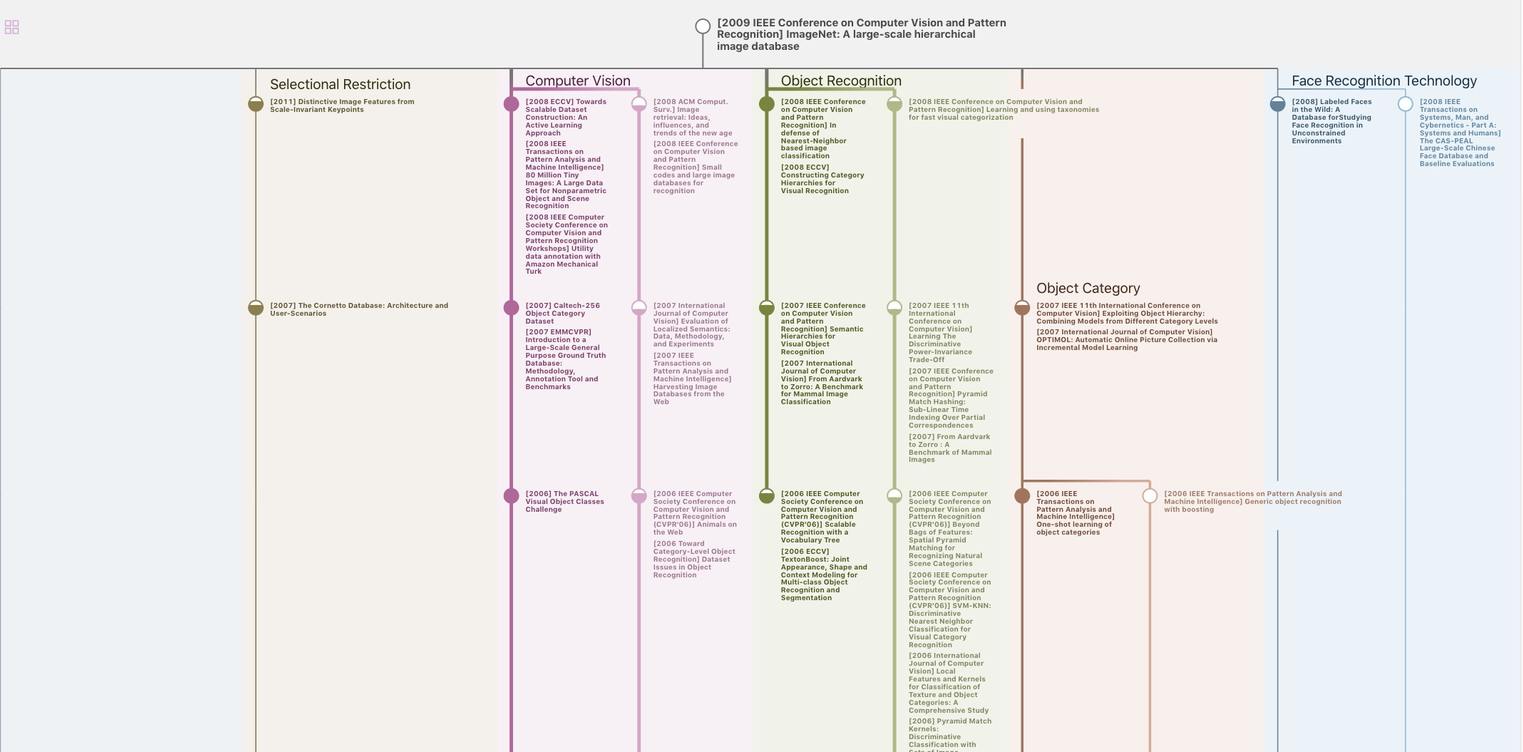
生成溯源树,研究论文发展脉络
Chat Paper
正在生成论文摘要