Adaptive Multi-View Joint Contrastive Learning on Graphs
ICASSP 2024 - 2024 IEEE International Conference on Acoustics, Speech and Signal Processing (ICASSP)(2024)
摘要
Recently, contrastive learning has shown promising results for representing graphs. Despite their success, several key issues have not been well addressed in existing studies: 1) Data noise and incompleteness are inevitable in the graph signal due to various factors. 2) Improper augmentation strategies may have negative effects on views construction and graph representation. In this study, we propose a novel joint contrastive learning model for graph representation named MVJCL. Specifically, a set of views were constructed with topology-level and node-level augmentation strategies. For each view, we execute two-layers GCNs to learn the node embeddings. Then, we propose a positive-negative-positive (pnp) contrastive learning task, which performs contrastive learning between the negative view and each positive view, so as alleviate the noise of supervision signal and exploit the most critical information. Extensive experiments on five real-world datasets demonstrate the effectiveness of MVJCL, where the maximum improvement can reach to 4.63%.
更多查看译文
关键词
Contrastive learning,self-supervised learning,graph augmentation,graph representation
AI 理解论文
溯源树
样例
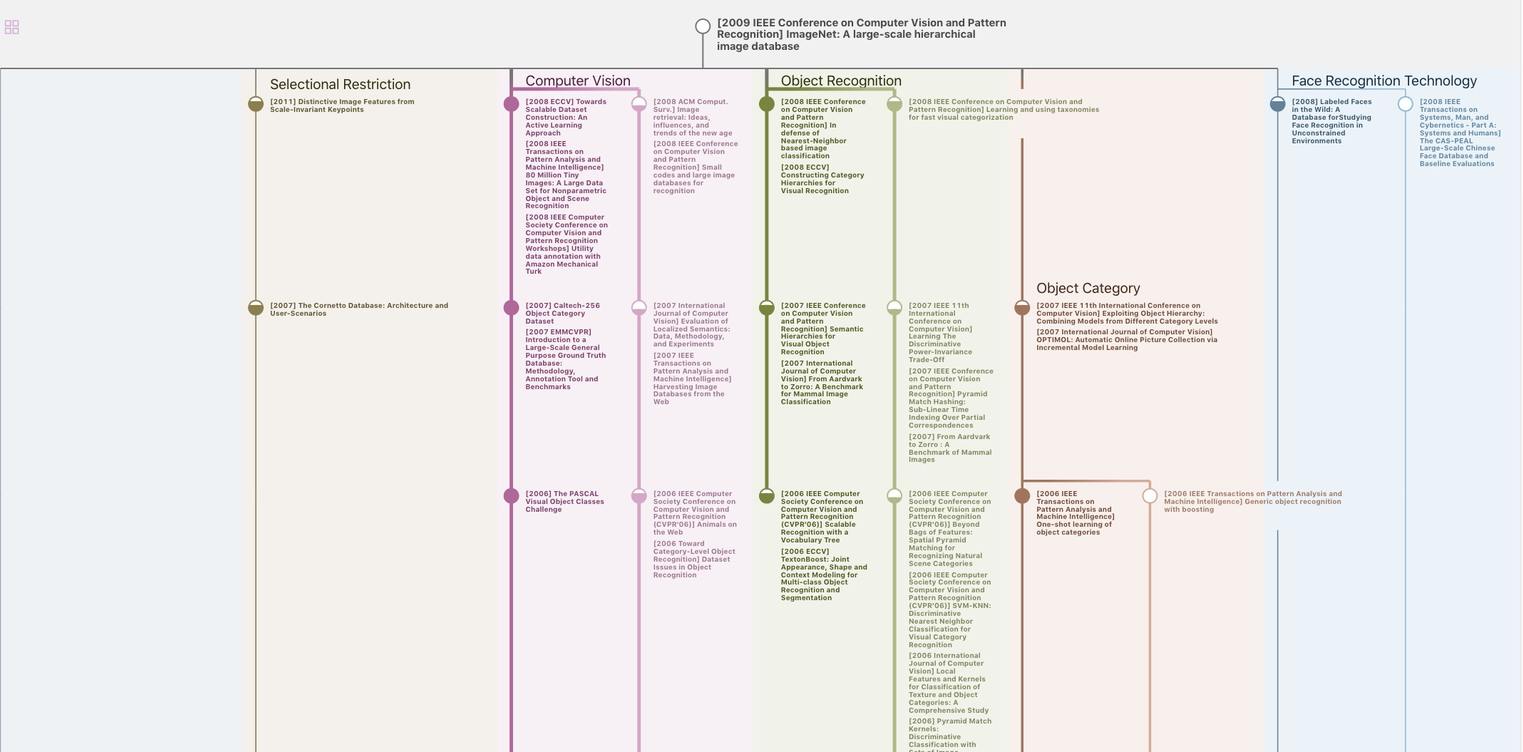
生成溯源树,研究论文发展脉络
Chat Paper
正在生成论文摘要