Geom-SAC: Geometric multi-discrete soft actor critic with applications in de novo drug design
IEEE Access(2024)
摘要
Finding new molecules with desirable properties has high computational and overhead costs. Much research has focused on generating candidate molecules in one- and two-dimensional spaces, which has produced some favorable results. However, extending these approaches to molecules in three-dimensional space would be far more useful because the representation of molecules is more realistic, although three-dimensional methods have much higher computational costs. In this work, we developed a geometric deep reinforcement learning agent that generates and optimizes molecules that could interact with a biochemical target. The agent can be used for generating molecules from scratch or for lead optimization when it enhances the properties of a given molecule, whether by enhancing its drug-likeness or increasing its activity toward the target via implicit learning. Thus, the agent works with molecules in three-dimensional space without high computational costs.
更多查看译文
关键词
Molecule generation,molecule optimization,geometric deep learning,reinforcement learning,de novo drug design
AI 理解论文
溯源树
样例
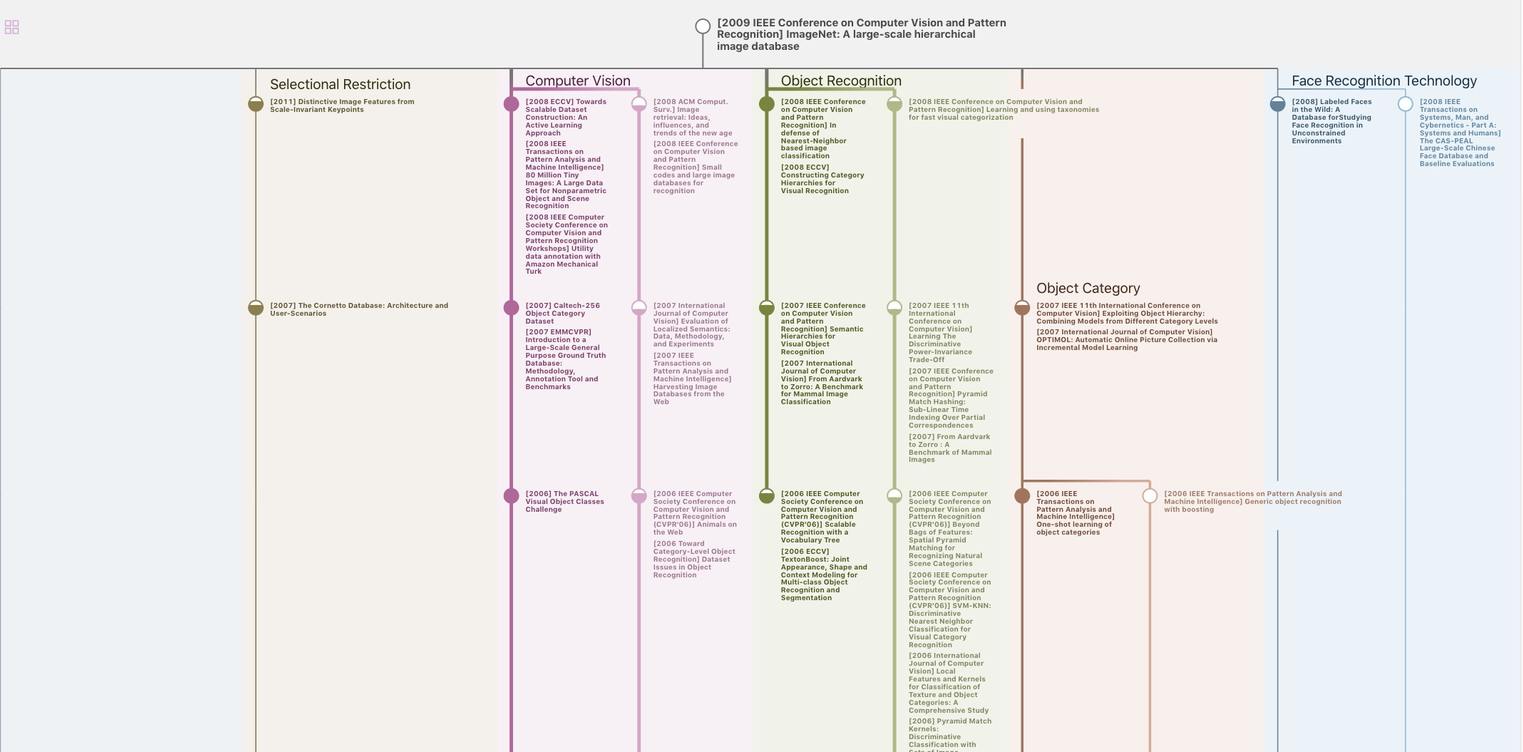
生成溯源树,研究论文发展脉络
Chat Paper
正在生成论文摘要