Bridging The Domain Gap Arising from Text Description Differences for Stable Text-To-Image Generation
ICASSP 2024 - 2024 IEEE International Conference on Acoustics, Speech and Signal Processing (ICASSP)(2024)
摘要
Generating high-quality images that conform to the semantics of captions has numerous potential applications. However, text-to-image generation is a challenging task due to its cross-modality nature. Current generative models are typically unstable, meaning that complex sentences can result in poor image quality. In this paper, we propose a novel model to bridge the domain gap arising from sentence complexity to achieve stable text-to-image generation. Our model includes two key modules, the attribute extraction module and the attribute fusion module. These modules can extract attributes from the captions and fuse them with image features to encourage the model to accurately understand the semantics. Our modules are plug-and-play and extensive experiments demonstrate that our approach outperforms the state-of-the-art GAN model. Our code and trained model are available at https://github.com/tantian21/stable-t2i-generation.
更多查看译文
关键词
Text-to-image generation,GAN,Diffusion
AI 理解论文
溯源树
样例
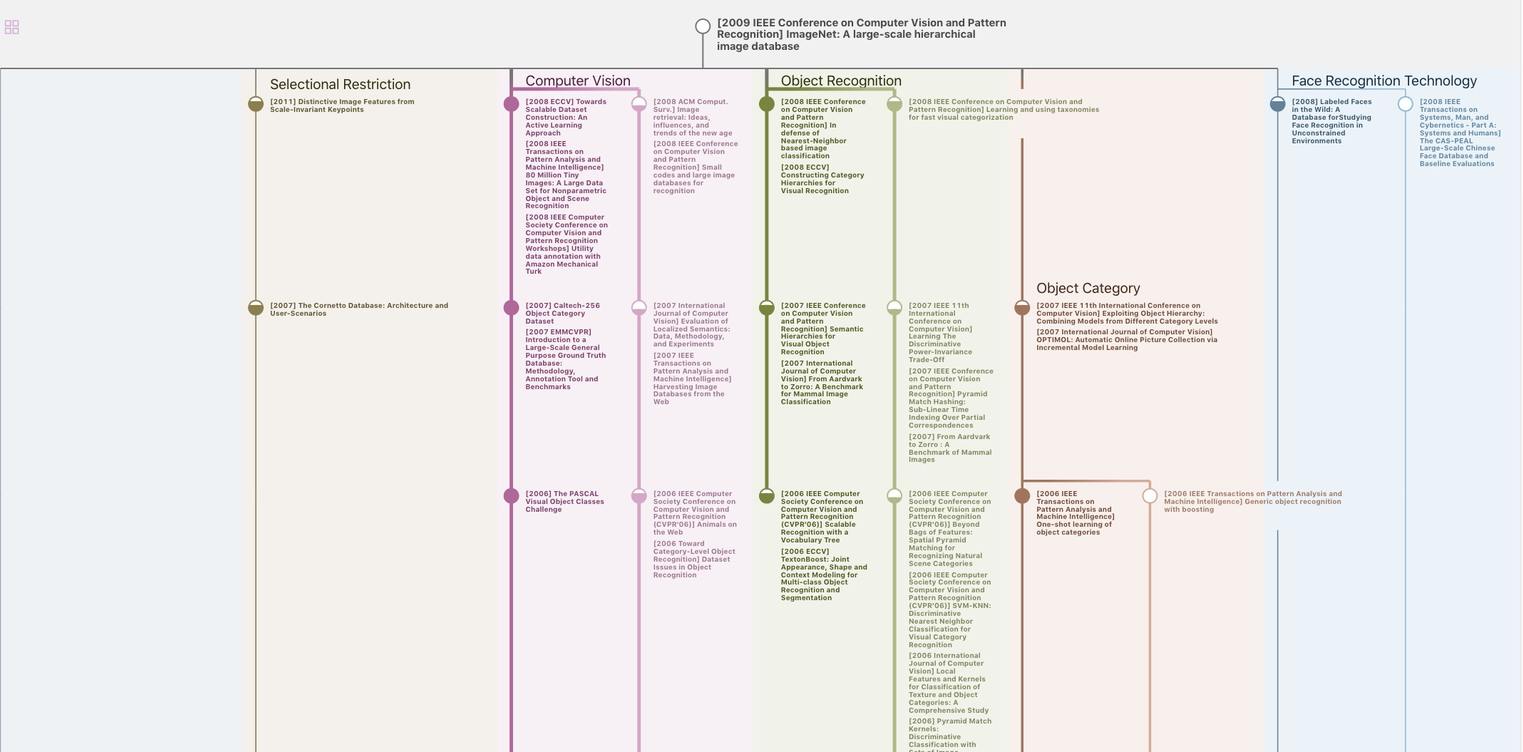
生成溯源树,研究论文发展脉络
Chat Paper
正在生成论文摘要