Selecting N-Lowest Scores for Training MOS Prediction Models
ICASSP 2024 - 2024 IEEE International Conference on Acoustics, Speech and Signal Processing (ICASSP)(2024)
摘要
The automatic speech quality assessment (SQA) has been extensively studied to predict the speech quality without time-consuming questionnaires. Recently, neural-based SQA models have been actively developed for speech samples produced by text-to-speech or voice conversion, with a primary focus on training mean opinion score (MOS) prediction models. The quality of each speech sample may not be consistent across the entire duration, and it remains unclear which segments of the speech receive the primary focus from humans when assigning subjective evaluation for MOS calculation. We hypothesize that when humans rate speech, they tend to assign more weight to low-quality speech segments, and the variance in ratings for each sample is mainly due to accidental assignment of higher scores when overlooking the poor quality speech segments. Motivated by the hypothesis, we analyze the VCC2018 and BVCC datasets. Based on the hypothesis, we propose the more reliable representative value N
low
-MOS, the mean of the N-lowest opinion scores. Our experiments show that LCC and SRCC improve compared to regular MOS when employing N
low
-MOS to MOSNet training. This result suggests that N
low
-MOS is a more intrinsic representative value of subjective speech quality and makes MOSNet a better comparator of VC models.
更多查看译文
关键词
speech quality assessment,mean opinion score,subjective evaluation dataset,training sample selection,MOSNet
AI 理解论文
溯源树
样例
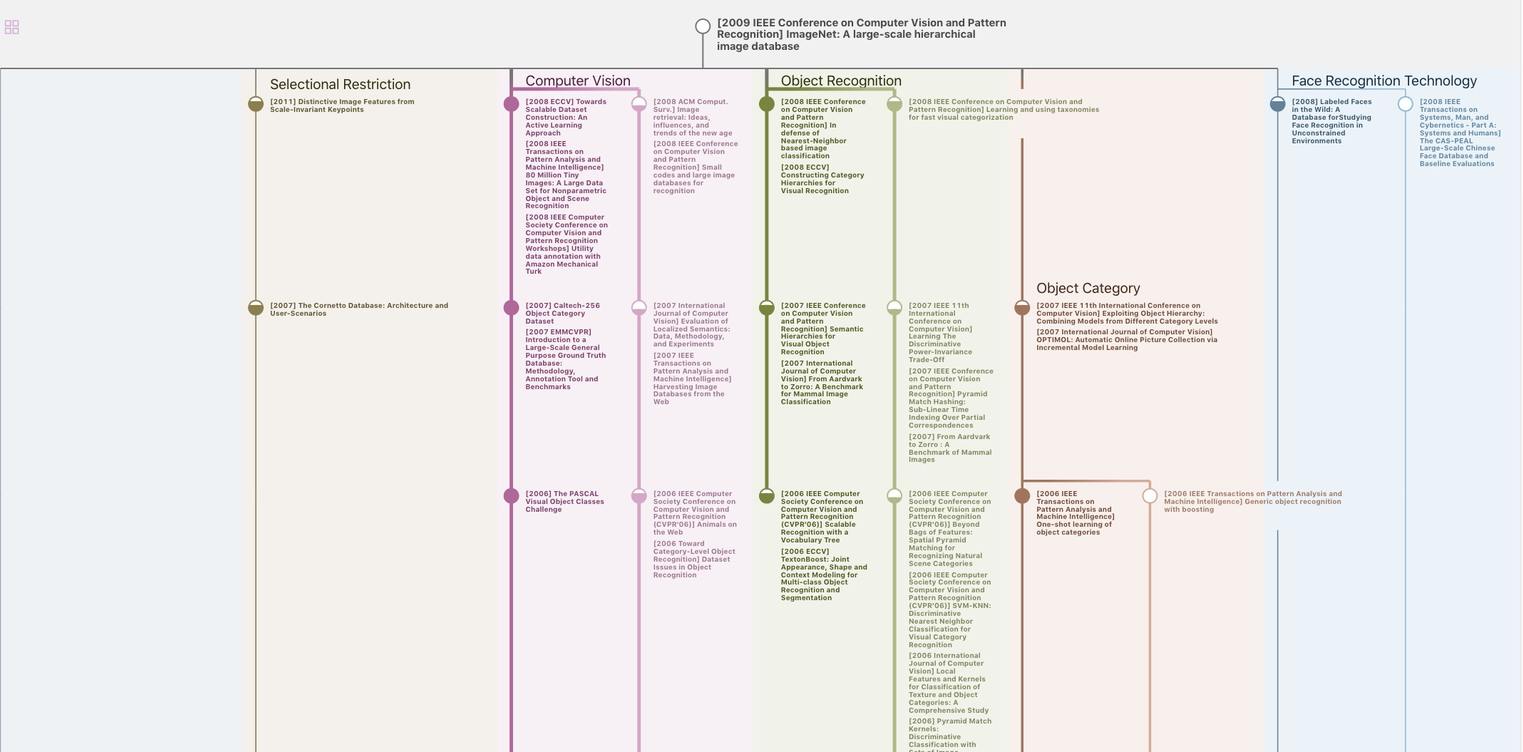
生成溯源树,研究论文发展脉络
Chat Paper
正在生成论文摘要