Multi-Level Augmentation Consistency Learning and Sample Selection for Semi-Supervised Domain Generalization
ICASSP 2024 - 2024 IEEE International Conference on Acoustics, Speech and Signal Processing (ICASSP)(2024)
摘要
Semi-supervised domain generalization (SSDG) aims to build a domain-generalized model using partially labeled data from source domains. Mainstream SSDG methods follow the augmentation consistency in FixMatch. However, the extraction of domain-invariant features may be challenging due to the absence of feature-based operations, further leading to overfitting of the classifier. To this end, we propose Multi-level Augmentation Consistency Learning (MACMatch), which improves the generalization of feature extractor and classifier through feature-based augmentation consistency. On the other hand, existing methods assume labeled data are class-balanced and domain-balanced, which is easily violated in practice. Based on this, we introduce Representativity and Diversity-based Sample Selection (RDSS), which models data as graphs to evaluate reasonable samples for labeling, relaxing the assumption for labeled data. Experiments on PACS and OfficeHome demonstrate that MACMatch outperforms state-of-the-art SSDG methods. Furthermore, MAC-Match with RDSS achieves competitive results without domain and class priori assumptions. Code is available at https://github.com/Y-J-Zhang/MACMatch-RDSS.
更多查看译文
关键词
Semi-supervised domain generalization,image classification,consistency regularization,sample selection
AI 理解论文
溯源树
样例
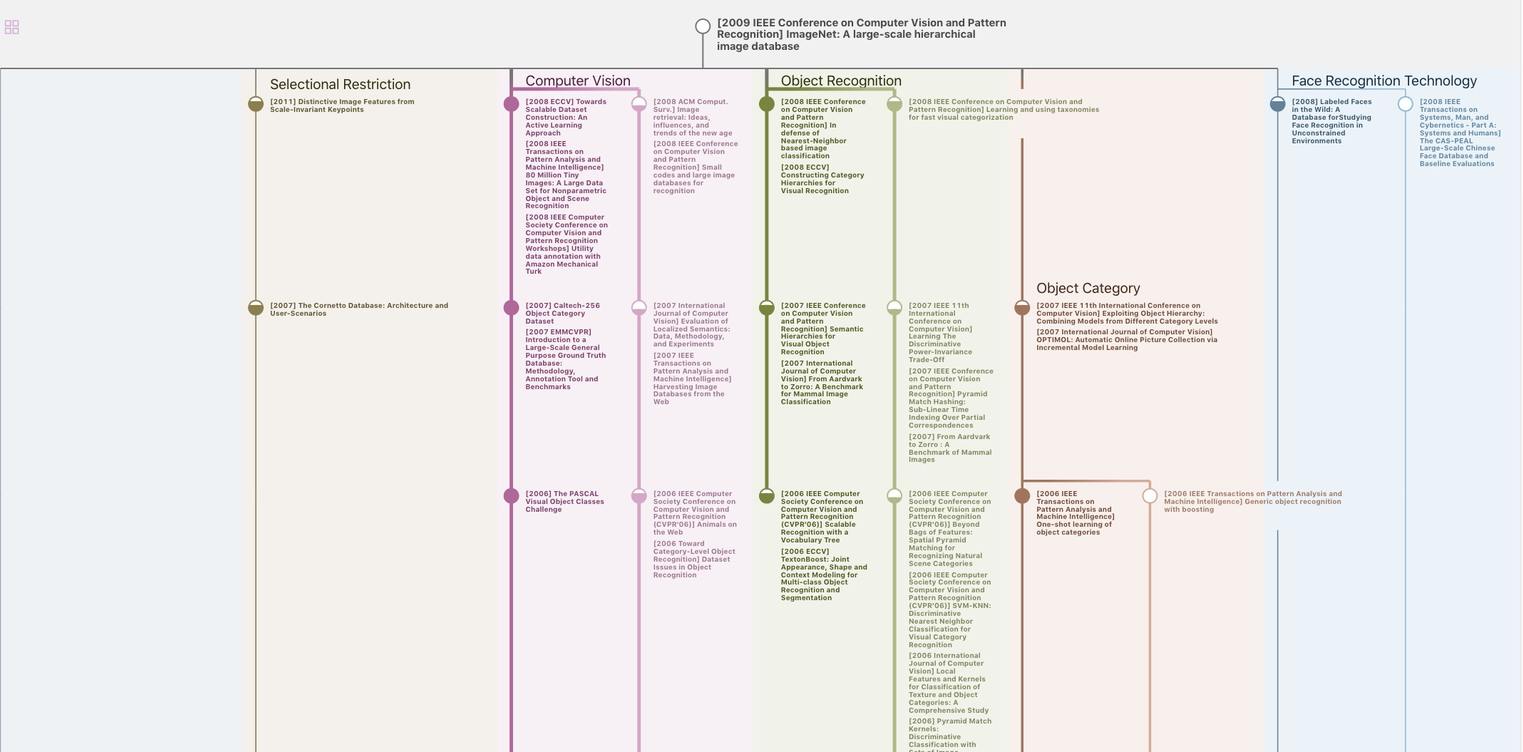
生成溯源树,研究论文发展脉络
Chat Paper
正在生成论文摘要